Reconstructing Ocean Subsurface Temperature and Salinity from Sea Surface Information Based on Dual Path Convolutional Neural Networks
JOURNAL OF MARINE SCIENCE AND ENGINEERING(2023)
摘要
Satellite remote sensing can provide observation information of the sea surface, and using the sea surface information to reconstruct the subsurface temperature (ST) and subsurface salinity (SS) information has significant application values. This study proposes an intelligent algorithm based on Dual Path Convolutional Neural Networks (DP-CNNs) to reconstruct the ST and SS. The DP-CNN can integrate known information including sea surface temperature (SST), sea surface salinity (SSS), and sea surface height (SSH) to reconstruct the ST and SS. The reconstruction model based on DP-CNN can solve the problem of detail information loss in traditional CNN (Convolutional Neural Network) models. This study performs experiments for the South China Sea under different seasons using reanalysis data. The experimental results show that the DP-CNN models have higher reconstruction accuracy than the CNN models, and this proves that DP-CNNs effectively mitigate the loss of detailed information in the CNN models. Compared with the ground truth data, the ST/SS reconstruction results of the DP-CNN model exhibited a high coefficient of determination (0.93/0.86) and a low root mean square error (around 0.31 ?/0.05 PSU). Therefore, the DP-CNN models can be used as an effective approach to reconstruct ST and SS using sea surface information.
更多查看译文
关键词
dual path convolutional neural networks,deep learning,subsurface ocean temperature and salinity,satellite remote sensing observation
AI 理解论文
溯源树
样例
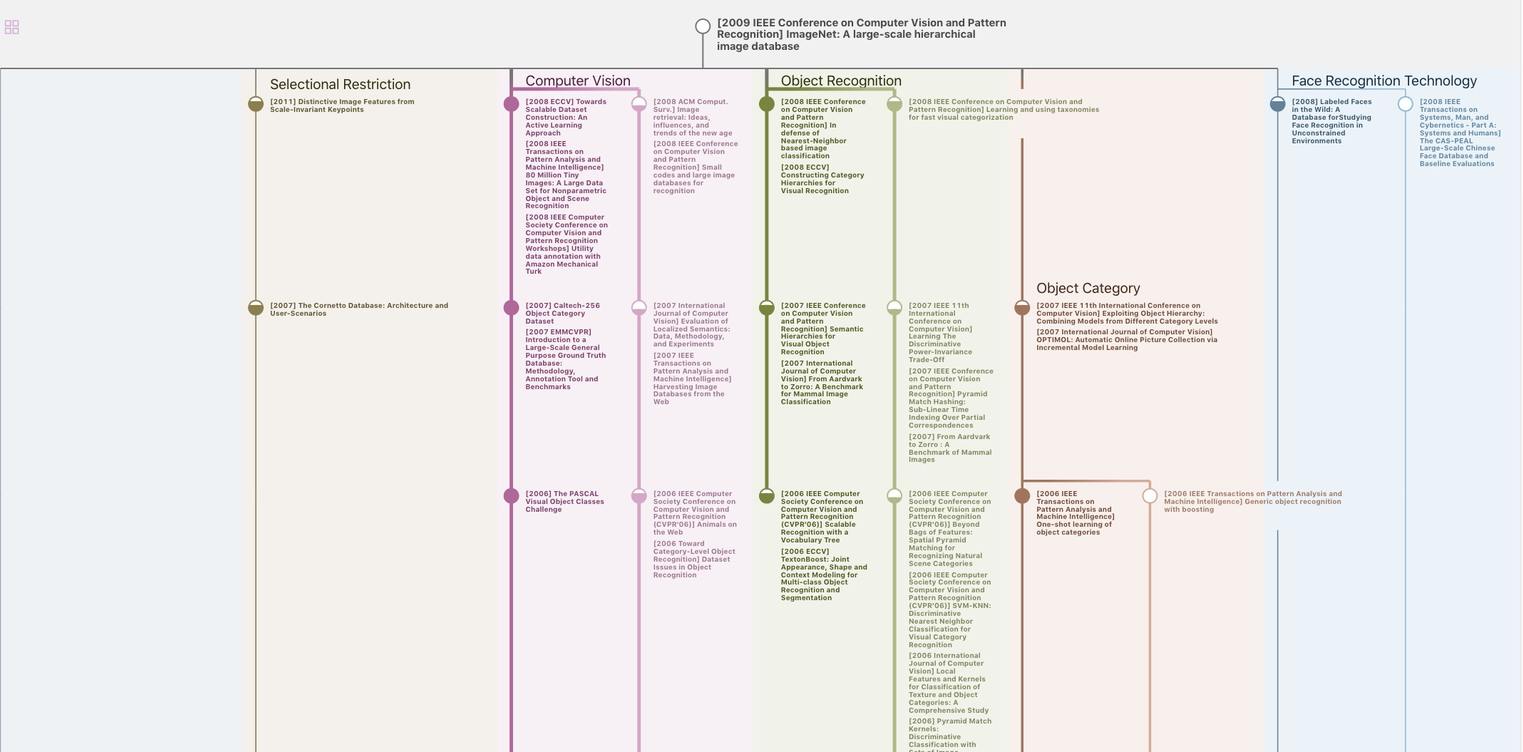
生成溯源树,研究论文发展脉络
Chat Paper
正在生成论文摘要