A Robust Model Averaging Approach for Partially Linear Models with Responses Missing at Random
Scandinavian journal of statistics(2023)
摘要
In this paper, with an assumed parametric model for the selection probability function, a robust model averaging estimation method is proposed for partially linear models with responses missing at random. The method is based on a weighted Mallows-type criterion. The method is robust in the sense that the asymptotic optimality holds true as long as the true model of the selection probability function is some measurable function of its assumed model. The optimal weight vector for model averaging is obtained by minimizing the weighted Mallows-type criterion. It is shown that the robust model averaging method achieves the lowest possible squared error asymptotically. Some simulation studies were conducted to evaluate the proposed method. An application to two real examples are provided as illustration.
更多查看译文
关键词
asymptotic optimality,inverse probability weighting,Mallows-type criterion,model misspecification,selection probability function
AI 理解论文
溯源树
样例
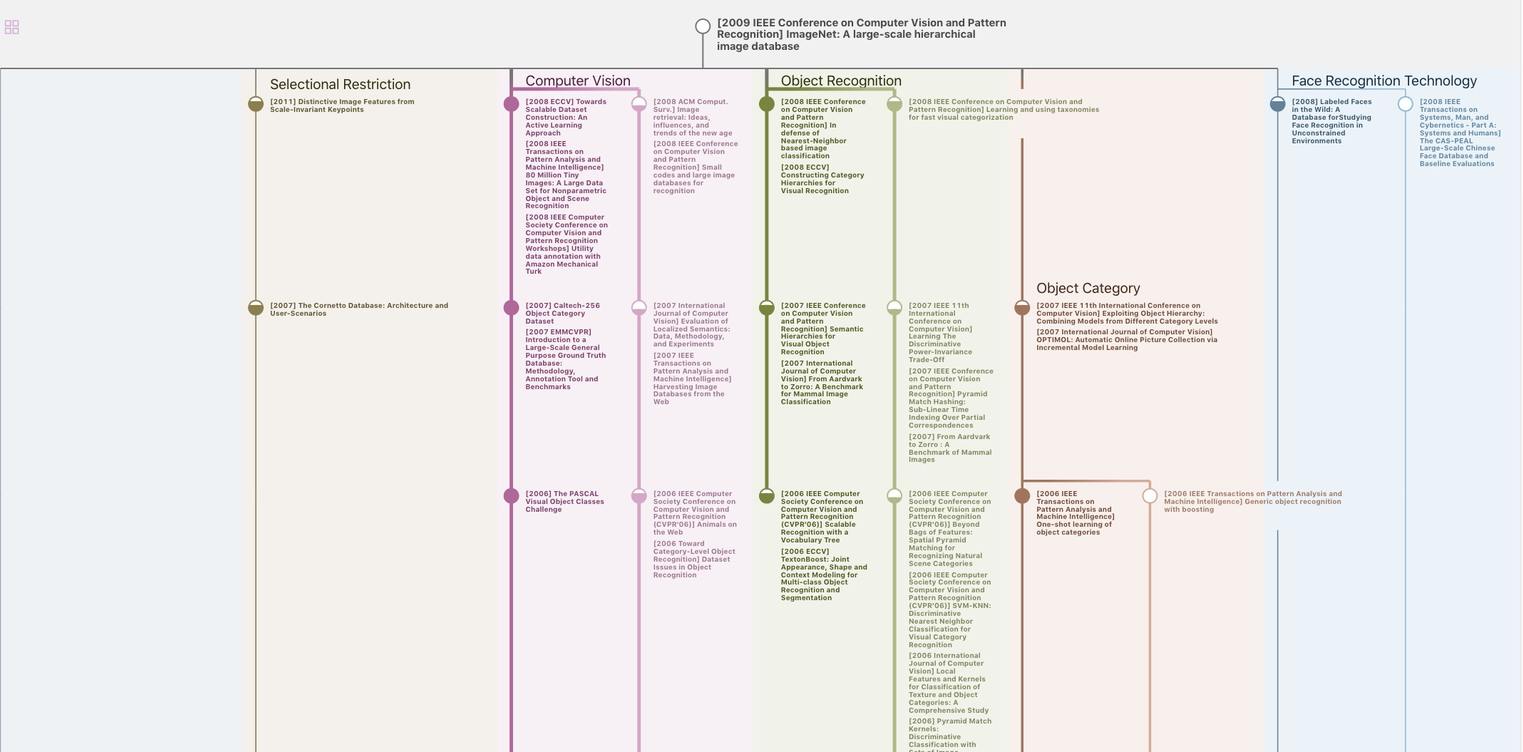
生成溯源树,研究论文发展脉络
Chat Paper
正在生成论文摘要