Interpreting Conv-LSTM for Spatio-Temporal Soil Moisture Prediction in China
AGRICULTURE-BASEL(2023)
摘要
Soil moisture (SM) is a key variable in Earth system science that affects various hydrological and agricultural processes. Convolutional long short-term memory (Conv-LSTM) networks are widely used deep learning models for spatio-temporal SM prediction, but they are often regarded as black boxes that lack interpretability and transparency. This study aims to interpret Conv-LSTM for spatio-temporal SM prediction in China, using the permutation importance and smooth gradient methods for global and local interpretation, respectively. The trained Conv-LSTM model achieved a high R2 of 0.92. The global interpretation revealed that precipitation and soil properties are the most important factors affecting SM prediction. Furthermore, the local interpretation showed that the seasonality of variables was more evident in the high-latitude regions, but their effects were stronger in low-latitude regions. Overall, this study provides a novel approach to enhance the trust-building for Conv-LSTM models and to demonstrate the potential of artificial intelligence-assisted Earth system modeling and understanding element prediction in the future.
更多查看译文
关键词
explainable artificial intelligence,deep learning,soil moisture prediction,interpretation
AI 理解论文
溯源树
样例
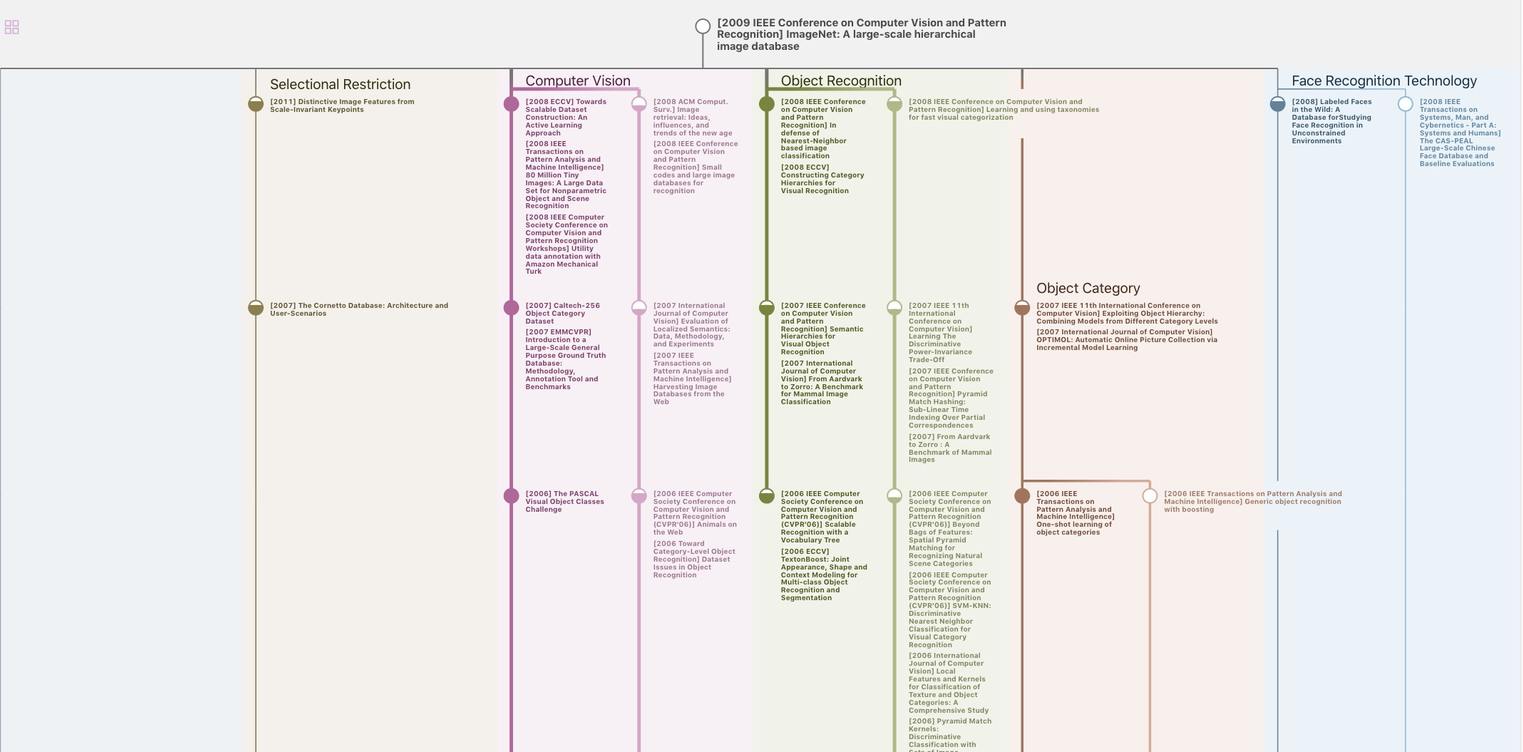
生成溯源树,研究论文发展脉络
Chat Paper
正在生成论文摘要