An active learning reliability algorithm using DMSSA-optimized Kriging model and parallel infilling strategy
QUALITY AND RELIABILITY ENGINEERING INTERNATIONAL(2023)
摘要
Due to the limited sample sizes and highly complicated performance functions, how to improve the reliability calculation accuracy and efficiency is an important issue for complex equipment working in harsh environments. This paper proposes an active learning reliability algorithm based on the double-mutation slap swarm algorithm-optimized (DMSSA) Kriging surrogate model, parallel infilling strategy and subset simulation (SS). In the method, the uniform design (UD) is employed to select the initial sampling points and their real responses of performance functions are estimated. The DMSSA integrates Cauchy and Gauss mutation to explore the optimal hyperparameter of Kriging model with high accuracy. The parallel infilling strategy combines the expected improvement (EI), minimizing prediction (MP) and mean squared error (MSE) criteria to find new sampling points, which are adaptively added into the database in each iteration to update the design of experiment (DoE). Ultimately, the SS method is employed to deal with the small failure probability problems. Five various examples are analyzed to verify the calculation accuracy and efficiency of the proposed method.
更多查看译文
关键词
double-mutation slap swarm algorithm, failure probability, Kriging surrogate model, parallel infilling strategy, subset simulation
AI 理解论文
溯源树
样例
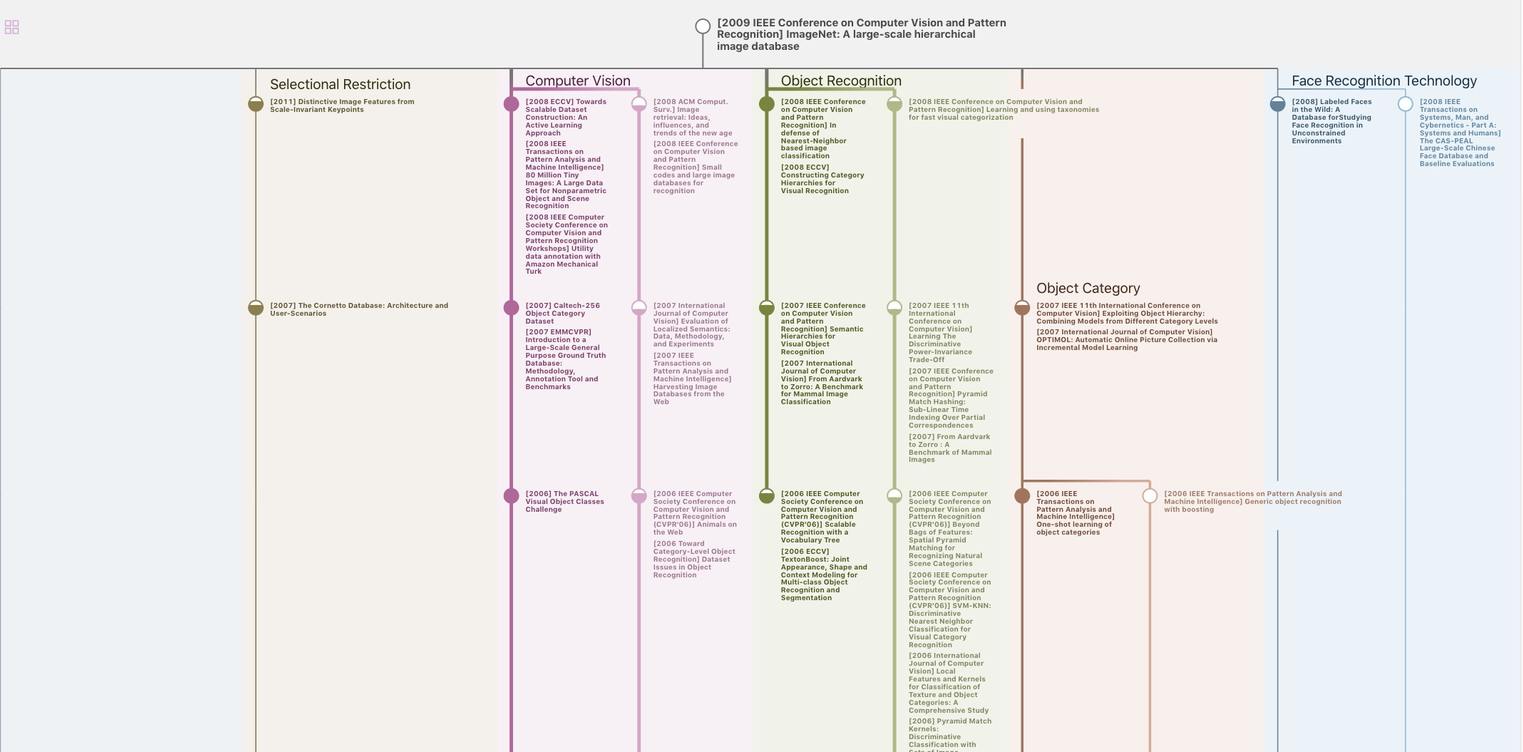
生成溯源树,研究论文发展脉络
Chat Paper
正在生成论文摘要