A Deep Learning Pipeline Using Prior Knowledge for Automatic Evaluation of Placenta Accreta Spectrum Disorders With MRI
JOURNAL OF MAGNETIC RESONANCE IMAGING(2024)
摘要
Background: The diagnosis of prenatal placenta accreta spectrum (PAS) with magnetic resonance imaging (MRI) is highly dependent on radiologists' experience. A deep learning (DL) method using the prior knowledge that PAS-related signs are generally found along the utero-placental borderline (UPB) may help radiologists, especially those with less experience, to mitigate this issue. Purpose: To develop a DL tool for antenatal diagnosis of PAS using T2-weighted MR images. Study Type: Retrospective. Subjects: Five hundred and forty pregnant women with clinically suspected PAS disorders from two institutions, divided into training (409), internal test (103), and external test (28) datasets. Field Strength/Sequence: Sagittal T2-weighted fast spin echo sequence at 1.5 T and 3 T. Assessment: An nnU-Net was trained for placenta segmentation. The UPB straightening approach was used to extract the utero-placental boundary region. The UPB image was then fed into DenseNet-PAS for PAS diagnosis. DenseNet-PP learnt placental position information to improve the PAS diagnosis performance. Three radiologists with 8, 10, and 12 years of experience independently evaluated the images. Two radiologists marked the placenta tissue. Histopathological findings were the reference standard. Statistical Tests: Area under the curve (AUC) was used to evaluate the classification. Dice coefficient evaluated the segmentation between radiologists and the model performance. The Mann-Whitney U-test or the chi-squared test assessed the significance of differences. Decision curve analysis was used to determine clinical effectiveness. DeLong's test was used to compare AUCs. Results: Of the 540 patients, 170 had PAS disorders confirmed by histopathology. The DL model using UPB images and placental position yielded the highest AUC of 0.860 and 0.897 in internal test and external test cohorts, respectively, significantly exceeding the performance of three radiologists (internal test AUC, 0.737-0.770). Data Conclusion: By extracting the UPB image, this fully automatic DL pipeline achieved high accuracy and may assist radiologists in PAS diagnosis using MRI.
更多查看译文
关键词
prenatal diagnosis,placenta accreta,computer assisted diagnosis,deep learning
AI 理解论文
溯源树
样例
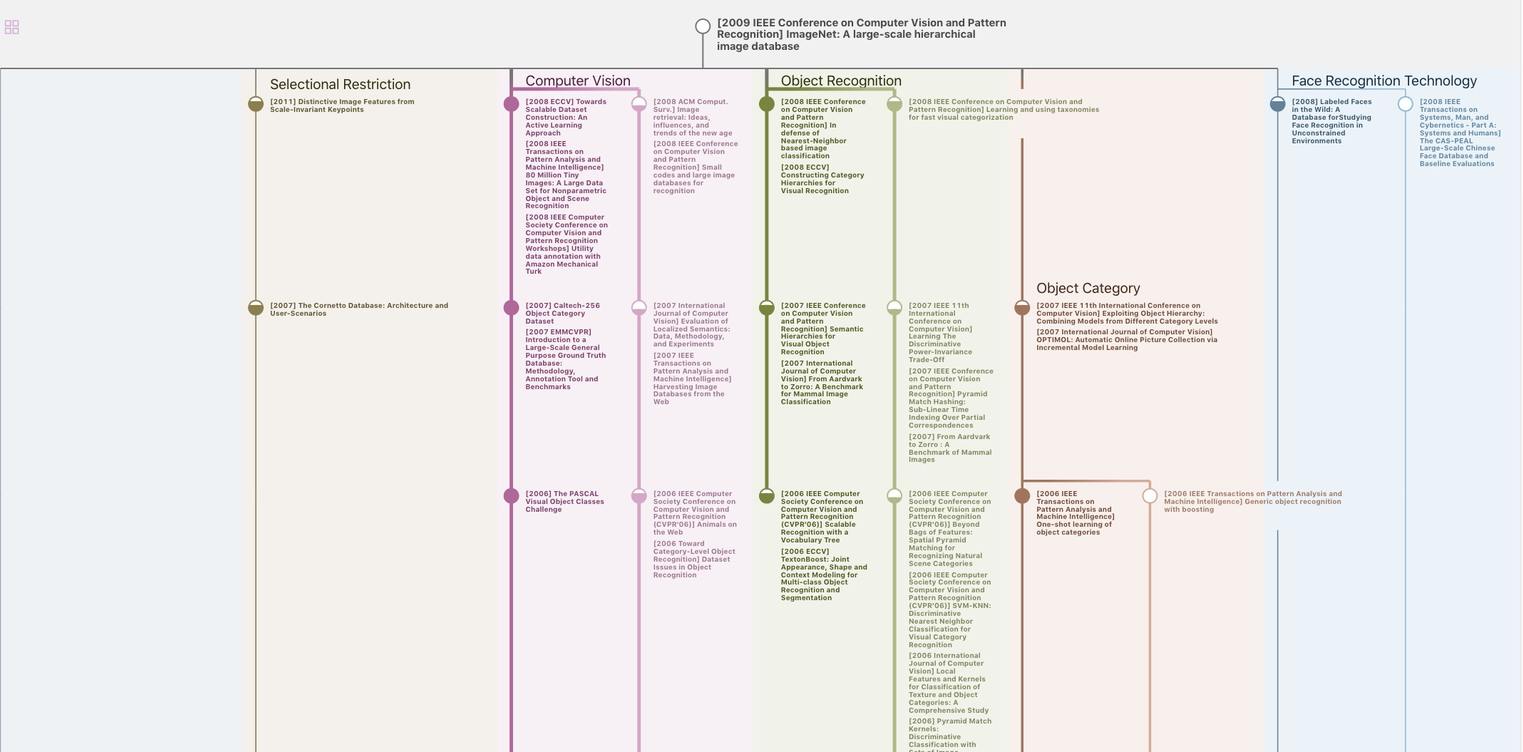
生成溯源树,研究论文发展脉络
Chat Paper
正在生成论文摘要