Prediction of Sintered Density of Binary W(Mo) Alloys Using Machine Learning
Rare metals/Rare Metals(2023)
摘要
Powder metallurgy is the optimal method for the consolidation and preparation of W(Mo) alloys, which exhibit excellent application prospects at high temperatures. The properties of W(Mo) alloys are closely related to the sintered density. However, controlling the sintered density and porosity of these alloys is still challenging. In the past, the regulation methods mainly focused on time-consuming and costly trial-and-error experiments. In this study, the sintering data for more than a dozen W(Mo) alloys constituted a small-scale dataset, including both solid and liquid phases sintering. Furthermore, simple descriptors were used to predict the sintered density of W(Mo) alloys based on the descriptor selection strategy and machine learning method (ML), where ML algorithm included the least absolute shrinkage and selection operator (Lasso) regression, k-nearest neighbor (k-NN), random forest (RF), and multi-layer perceptron (MLP). The results showed that the interpretable descriptors extracted by our proposed selection strategy and the MLP neural network achieved a high prediction accuracy (R > 0.950). By further predicting the sintered density of W(Mo) alloys using different sintering processes, the error between the predicted and experimental values was less than 0.063, confirming the application potential of the model.
更多查看译文
关键词
Sintered density,W(Mo) alloy,Machine learning (ML),Interpretable descriptors,Multi-layer perceptron (MLP)
AI 理解论文
溯源树
样例
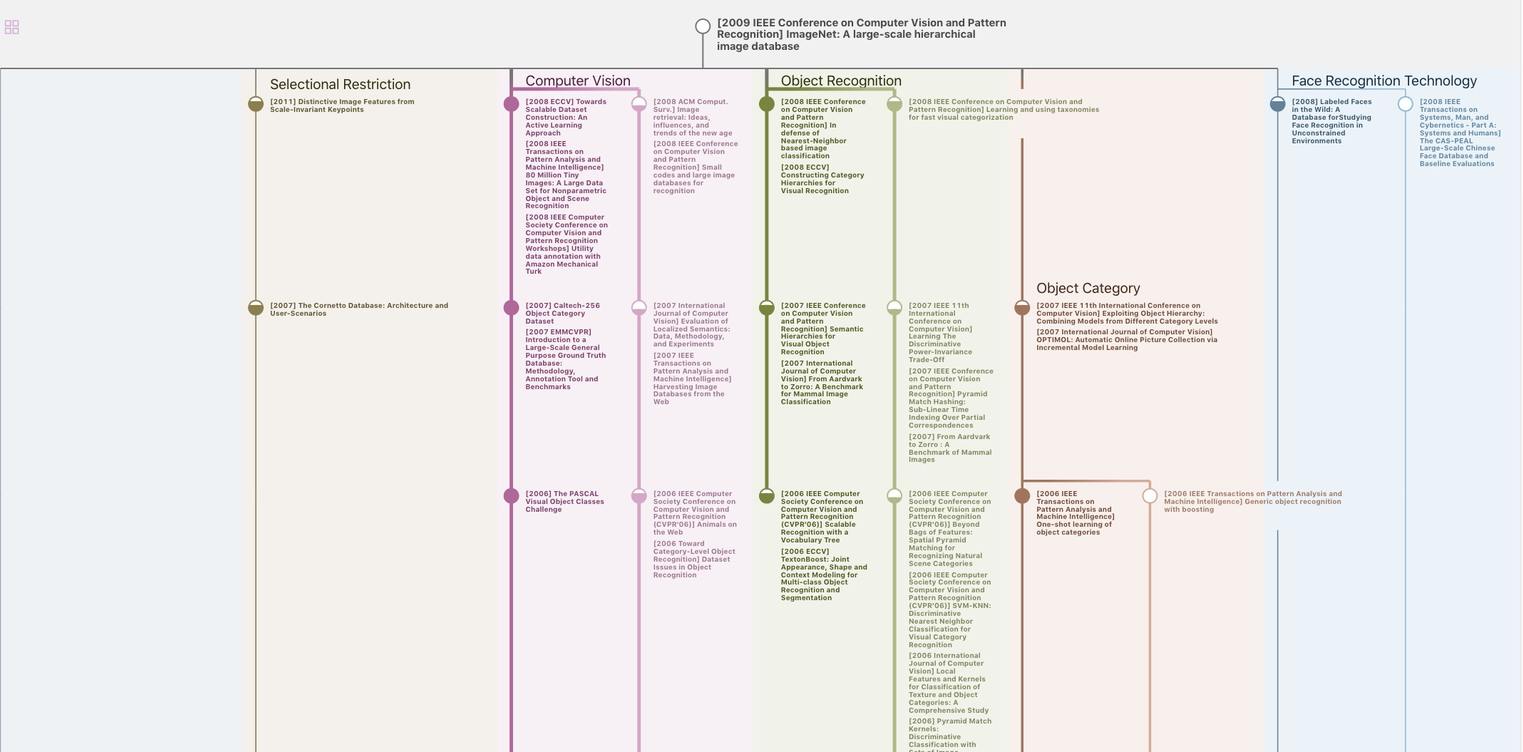
生成溯源树,研究论文发展脉络
Chat Paper
正在生成论文摘要