Universum parametric ν -support vector regression for binary classification problems with its applications
ANNALS OF OPERATIONS RESEARCH(2023)
摘要
Universum data sets, a collection of data sets that do not belong to any specific class in a classification problem, give previous information about data in the mathematical problem under consideration to enhance the classifiers’ generalization performance. Recently, some researchers have integrated Universum data into the existing models to propose new models which result in improved classification performance. Inspired by these Universum models, an efficient parametric ν -support vector regression with Universum data ( 𝔘 Par- ν -SVR) is proposed in this work. This method, which finds two non-parallel hyperplanes by solving one optimization problem and considers heteroscedastic noise, overcomes some common disadvantages of the previous methods. The 𝔘 Par- ν -SVR includes unlabeled samples that don’t belong to any class in the training process, which results in a quadratic programming problem. Two approaches are proposed to solve this problem. The first approach derives the dual formulation using the Lagrangian function and KKT conditions. Furthermore, a least squares parametric ν -support vector regression with Universum data (named LS- 𝔘 Par- ν -SVR) is suggested to further increase the generalization performance. The LS- 𝔘 Par- ν -SVR solves a single system of linear equations, instead of addressing a quadratic programming problem in the dual formulation. Numerical experiments on artificial, UCI, credit card, NDC, and handwritten digit recognition data sets show that the suggested Universum model and its solving methodologies improve prediction accuracy.
更多查看译文
关键词
Universum,Parametric ν -support vector,Least squares,Dual problem,Twin support vector machine
AI 理解论文
溯源树
样例
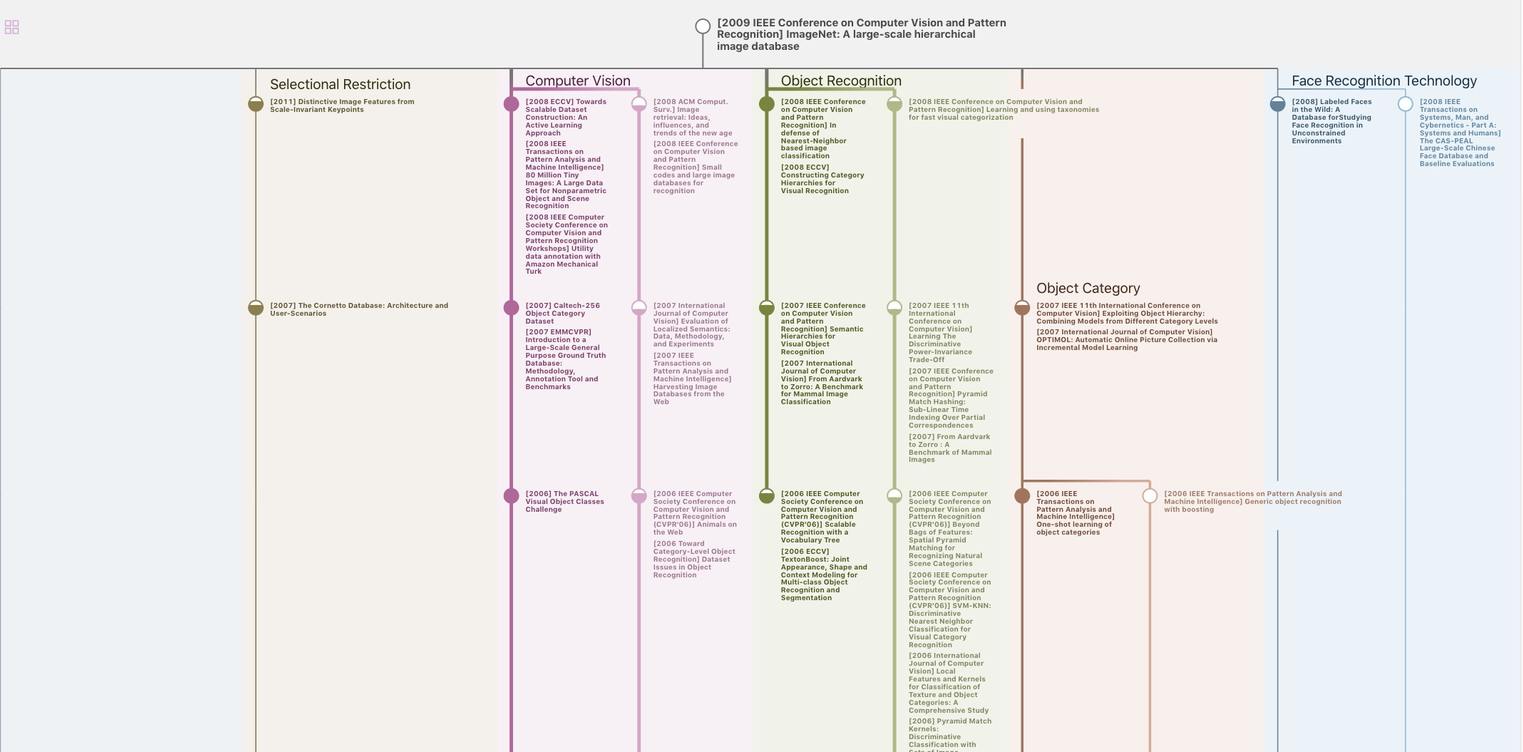
生成溯源树,研究论文发展脉络
Chat Paper
正在生成论文摘要