Precipitation Nowcasting Based on Deep Learning over Guizhou, China
ATMOSPHERE(2023)
摘要
Accurate precipitation nowcasting (lead time: 0-2 h), which requires high spatiotemporal resolution data, is of great relevance in many weather-dependent social and operational activities. In this study, we are aiming to construct highly accurate deep learning (DL) models to directly obtain precipitation nowcasting at 6-min intervals for the lead time of 0-2 h. The Convolutional Long Short-Term Memory (ConvLSTM) and Predictive Recurrent Neural Network (PredRNN) models were used as comparative DL models, and the Lucas-Kanade (LK) Optical Flow method was selected as a traditional extrapolation baseline. The models were trained with high-quality datasets (resolution: 1 min) created from precipitation observations recorded by automatic weather stations in Guizhou Province (China). A comprehensive evaluation of the precipitation nowcasting was performed, which included consideration of the root mean square error, equitable threat score (ETS), and probability of detection (POD). The evaluation indicated that the reduction of the number of missing values and data normalization boosted training efficiency and improved the forecasting skill of the DL models. Increasing the time series length of the training set and the number of training samples both improved the POD and ETS of the DL models and enhanced nowcasting stability with time. Training with the Hea-P dataset further improved the forecasting skill of the DL models and sharply increased the ETS for thresholds of 2.5, 8, and 15 mm, especially for the 1-h lead time. The PredRNN model trained with the Hea-P dataset (time series length: 8 years) outperformed the traditional LK Optical Flow method for all thresholds (0.1, 1, 2.5, 8, and 15 mm) and obtained the best performance of all the models considered in this study in terms of ETS. Moreover, the Method for Object-Based Diagnostic Evaluation on a rainstorm case revealed that the PredRNN model, trained well with high-quality observation data, could both capture complex nonlinear characteristics of precipitation more accurately than achievable using the LK Optical Flow method and establish a reasonable mapping network during drastic changes in precipitation. Thus, its results more closely matched the observations, and its forecasting skill for thresholds exceeding 8 mm was improved substantially.
更多查看译文
关键词
precipitation nowcasting,deep learning,guizhou
AI 理解论文
溯源树
样例
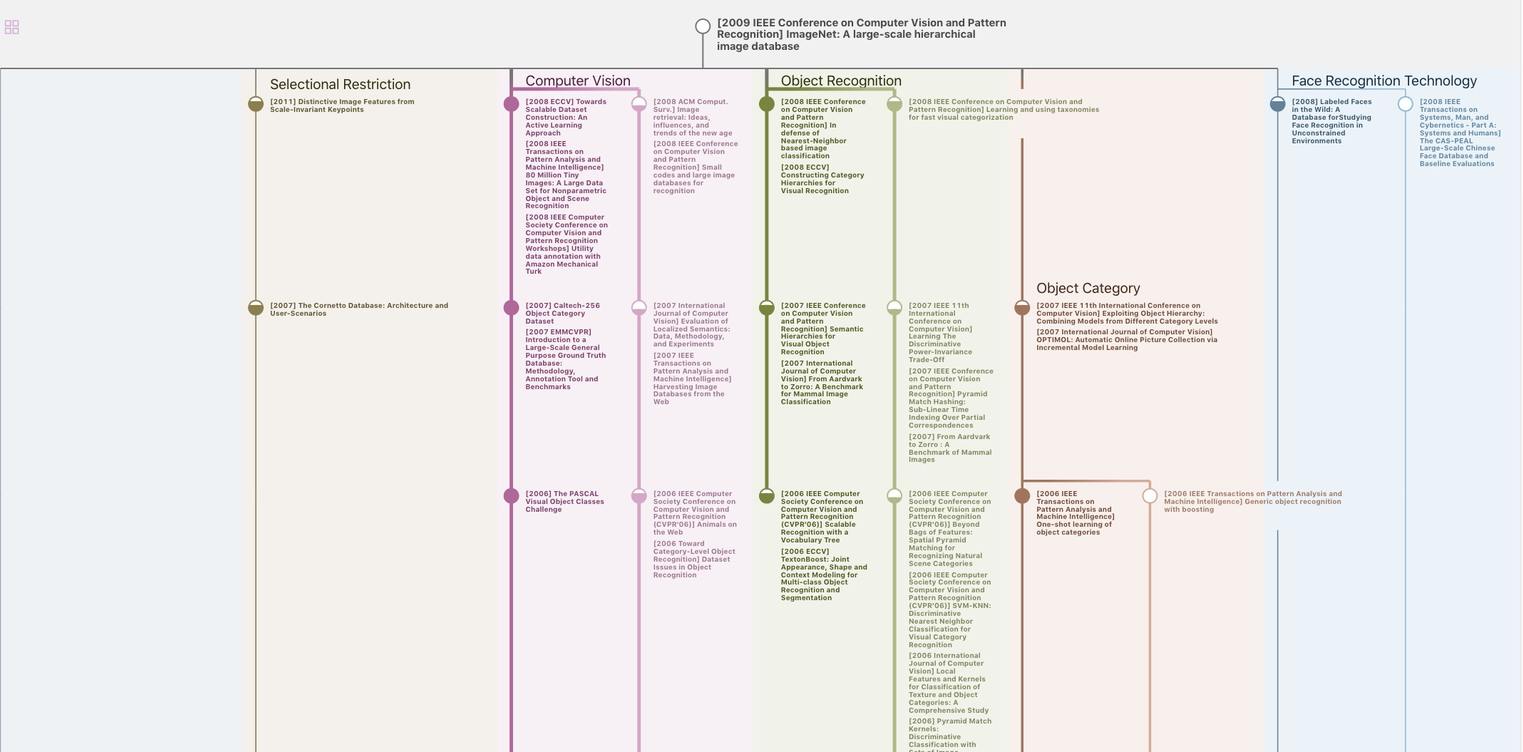
生成溯源树,研究论文发展脉络
Chat Paper
正在生成论文摘要