A new large-scale learning algorithm for generalized additive models
Mach. Learn.(2023)
摘要
Additive model plays an important role in machine learning due to its flexibility and interpretability in the prediction function. However, solving large-scale additive models is a challenging task due to several difficulties. Until now, scaling up additive models is still an open problem. To address this challenging problem, in this paper, we propose a new doubly stochastic optimization algorithm for solving the generalized additive models (DSGAM). We first propose a generalized formulation of additive models without the orthogonal hypothesis on the basis function. After that, we propose a wrapper algorithm to optimize the generalized additive models. Importantly, we introduce a doubly stochastic gradient algorithm (DSG) to solve an inner subproblem in the wrapper algorithm, which can scale well in sample size and dimensionality simultaneously. Finally, we prove the fast convergence rate of our DSGAM algorithm. The experimental results on various large-scale benchmark datasets not only confirm the fast convergence of our DSGAM algorithm, but also show a huge reduction of computational time compared with existing algorithms, while retaining the similar generalization performance.
更多查看译文
关键词
Additive model,Doubly stochastic gradient,Wrapper algorithm
AI 理解论文
溯源树
样例
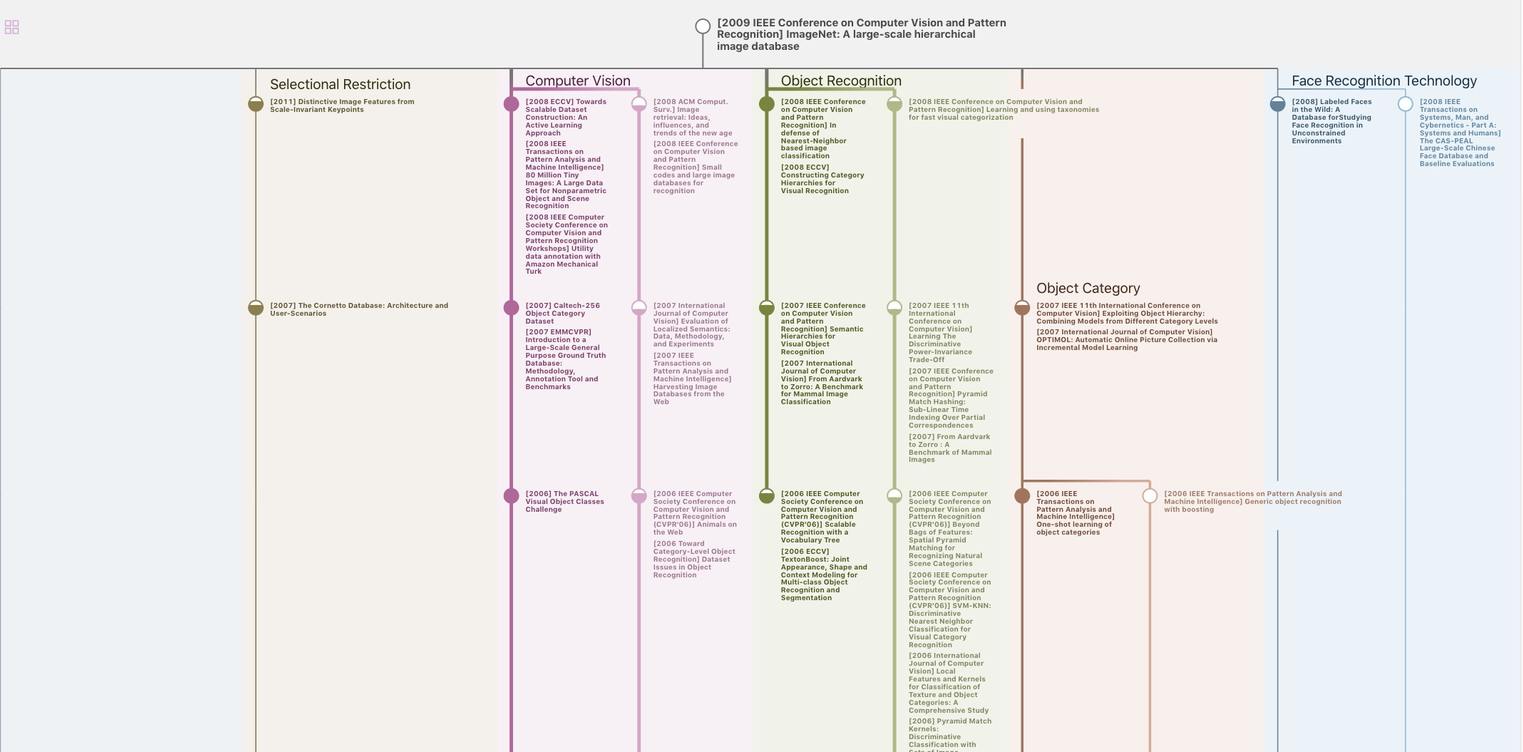
生成溯源树,研究论文发展脉络
Chat Paper
正在生成论文摘要