Joint Sparse Locality Preserving Regression for Discriminative Learning
IEEE TRANSACTIONS ON EMERGING TOPICS IN COMPUTATIONAL INTELLIGENCE(2024)
摘要
Ridge Regression (RR) is a classical method that is widely used in multiple regression analysis. However, traditional RR does not take the local geometric structure of data into consideration for discriminative learning and it is sensitive to outliers as it is based on L-2-norm. To address this problem, this article proposes a novel method called Joint Sparse Locality Preserving Regression (JSLPR) for discriminative learning. JSLPR not only applies L-2,L-1-norm on both loss function and regularization term but also takes the local geometric structure of the data into consideration. The use of L-2,L-1-norm can guarantee the robustness to outliers or noises and the joint sparsity for effective feature selection. Taking the local geometric structure into consideration can improve the performance of the feature extraction and selection method when the data lie on a manifold. To solve the optimization problem of JSLPR, an iterative algorithm is proposed and the convergence of the algorithm is also proven. Experiments on four famous face databases are conducted and the results show the merit of the proposed JSLPR on feature extraction and selection.
更多查看译文
关键词
Regression,jointly sparse,discriminative learning,feature selection,locality preserving
AI 理解论文
溯源树
样例
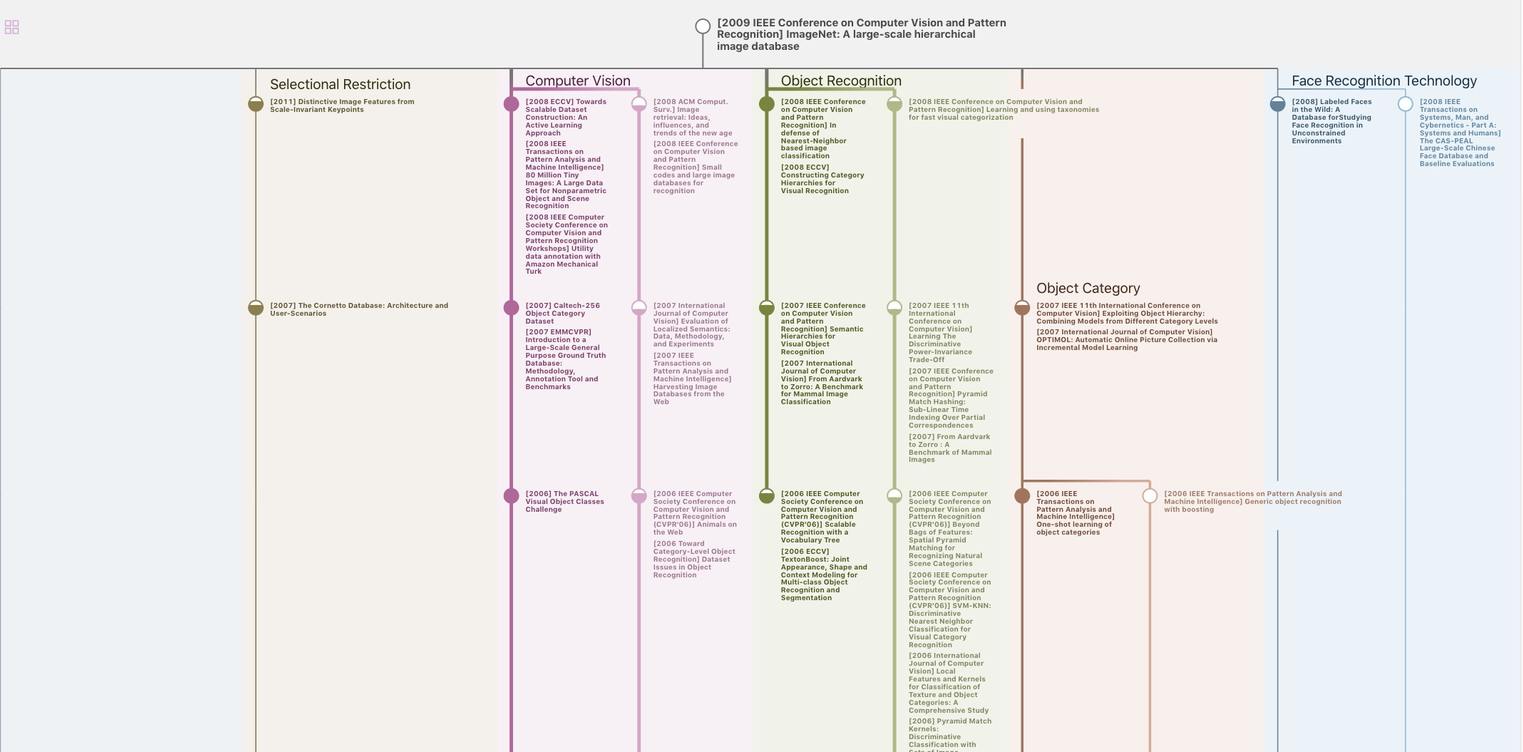
生成溯源树,研究论文发展脉络
Chat Paper
正在生成论文摘要