Flow Learning Based Dual Networks for Low-Light Image Enhancement
NEURAL PROCESSING LETTERS(2023)
摘要
The deep learning-based low-light image enhancement task aims to learn a mapping that converts low-light images to normally exposed images by training with paired or unpaired datasets. Most of these existing methods are based on convolutional neural networks, which largely limits the network’s ability to learn global information. Meanwhile, the reconstruction loss or adversarial loss they adopt often cannot accurately measure the visual distance between the prediction and the target, resulting in blurred regions in the enhancement results. In this paper, we propose a novel flow learning based dual networks (FDN), which consists of a dual network and a flow learning based model. The dual network is mainly composed of a reidual-based Unet encoder and a residual-based Swin Transformer encoder, which can make up for the lack of global information processing and has more advantages in processing deep and shallow information. Moreover, we use a single loss function named negative log-likelihood to train the entire network, which enables the flow models to adequately learn the complex conditional distribution of normally exposed images and avoid blurry outputs. Experimental results on two benchmark datasets show that the proposed FDN method can achieve the sate-of-the-art performances on low-light image enhancement task.
更多查看译文
关键词
Low-light image enhancement,Conditional normalizing flow,Residual,Unet,Swin transformer
AI 理解论文
溯源树
样例
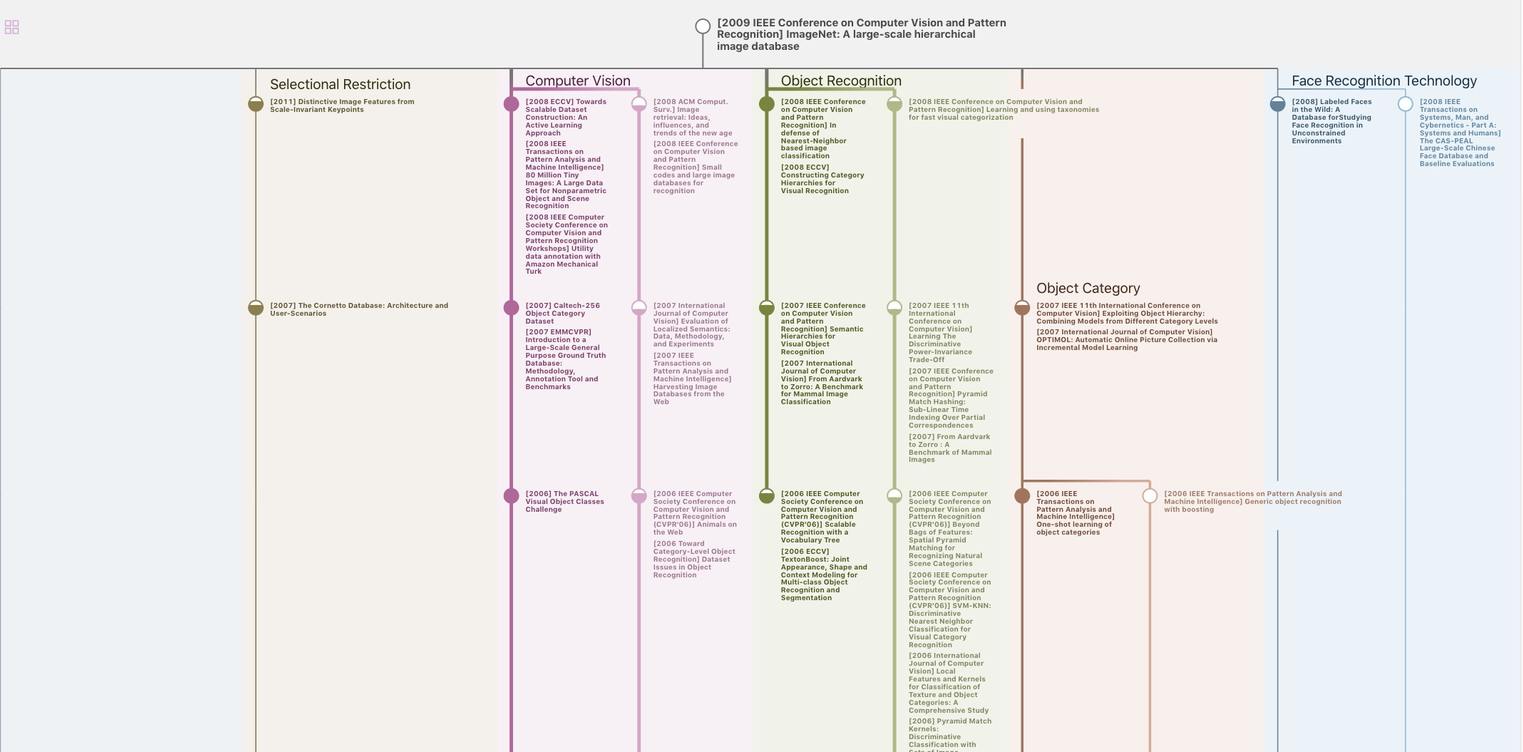
生成溯源树,研究论文发展脉络
Chat Paper
正在生成论文摘要