Mixed-decomposed convolutional network: A lightweight yet efficient convolutional neural network for ocular disease recognition
CAAI TRANSACTIONS ON INTELLIGENCE TECHNOLOGY(2023)
摘要
Eye health has become a global health concern and attracted broad attention. Over the years, researchers have proposed many state-of-the-art convolutional neural networks (CNNs) to assist ophthalmologists in diagnosing ocular diseases efficiently and precisely. However, most existing methods were dedicated to constructing sophisticated CNNs, inevitably ignoring the trade-off between performance and model complexity. To alleviate this paradox, this paper proposes a lightweight yet efficient network architecture, mixed-decomposed convolutional network (MDNet), to recognise ocular diseases. In MDNet, we introduce a novel mixed-decomposed depthwise convolution method, which takes advantage of depthwise convolution and depthwise dilated convolution operations to capture low-resolution and high-resolution patterns by using fewer computations and fewer parameters. We conduct extensive experiments on the clinical anterior segment optical coherence tomography (AS-OCT), LAG, University of California San Diego, and CIFAR-100 datasets. The results show our MDNet achieves a better trade-off between the performance and model complexity than efficient CNNs including MobileNets and MixNets. Specifically, our MDNet outperforms MobileNets by 2.5% of accuracy by using 22% fewer parameters and 30% fewer computations on the AS-OCT dataset.
更多查看译文
关键词
artificial intelligence,deep learning,deep neural networks,image analysis,image classification,medical applications,medical image processing
AI 理解论文
溯源树
样例
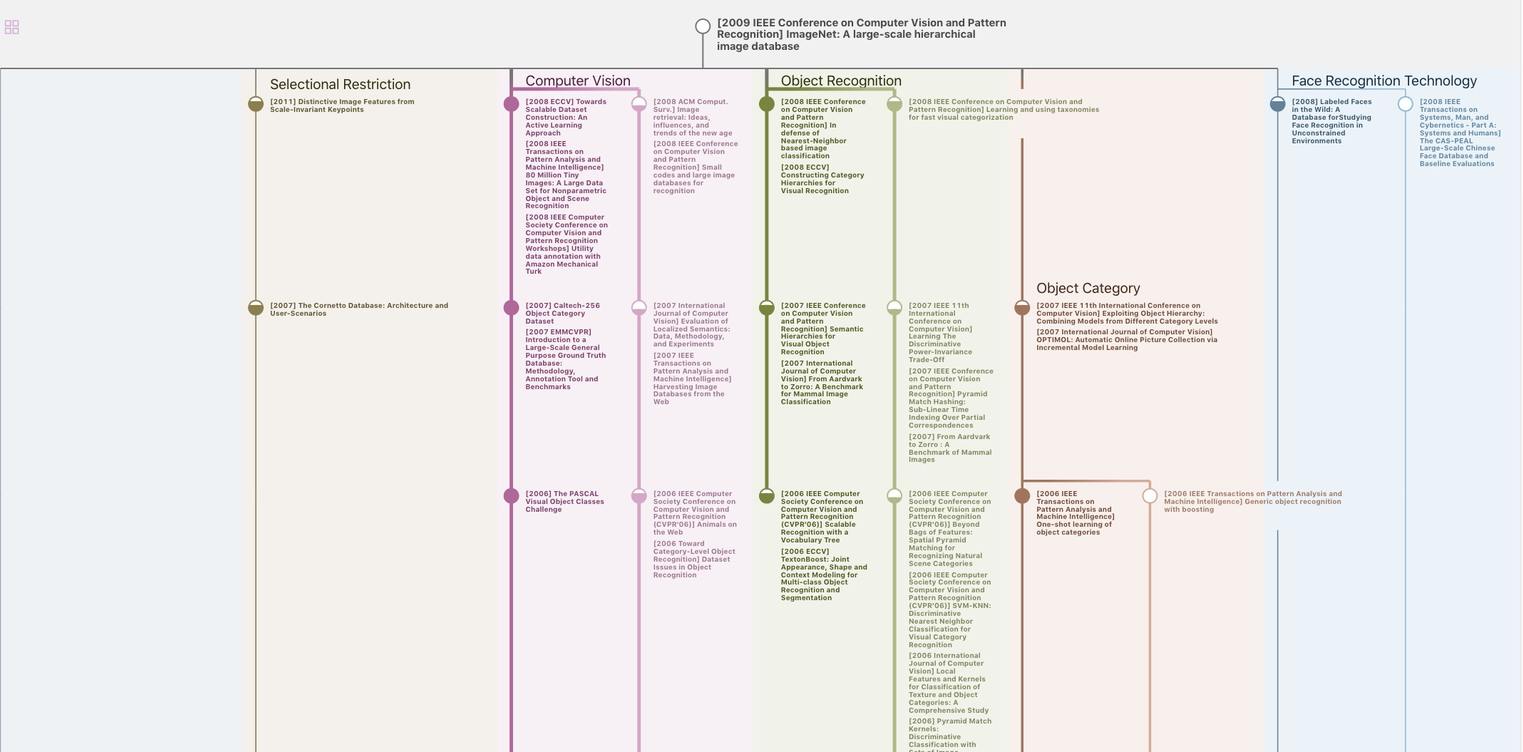
生成溯源树,研究论文发展脉络
Chat Paper
正在生成论文摘要