CoGCN: co-occurring item-aware GCN for recommendation
NEURAL COMPUTING & APPLICATIONS(2023)
摘要
Graph convolution networks (GCNs) play an increasingly vital role in recommender systems, due to their remarkable relation modeling and representation capabilities. Concretely, they can capture high-order semantic correlations within sparse bipartite interaction graphs, thereby enhancing user–item collaborative encodings. Despite the exciting prospects, the existing GCN-based models mainly focus on user–item interactions and seldom consider effectiveness of the side item co-occurrence information on user behavior guidance, resulting in limited performance improvement. Therefore, we propose a novel side item co-occurrence information-aware GCN model. Specifically, we first decouple the original heterogeneous relation graph into corresponding user–item and item–item subgraphs for user–item interaction and item co-occurrence relation modeling. Thereafter, we conduct adaptive iterative aggregation on these subgraphs for user intention understanding and co-occurring item correlation perception. Finally, we present two semantic fusion strategies for sufficient user–item semantic collaborative learning, thereby boosting the overall recommendation performance. Extensive comparison experiments are conducted on three benchmark datasets to justify the superiority of our model.
更多查看译文
关键词
Recommender system,Collaborative filtering,Graph neural network,Item co-occurrence
AI 理解论文
溯源树
样例
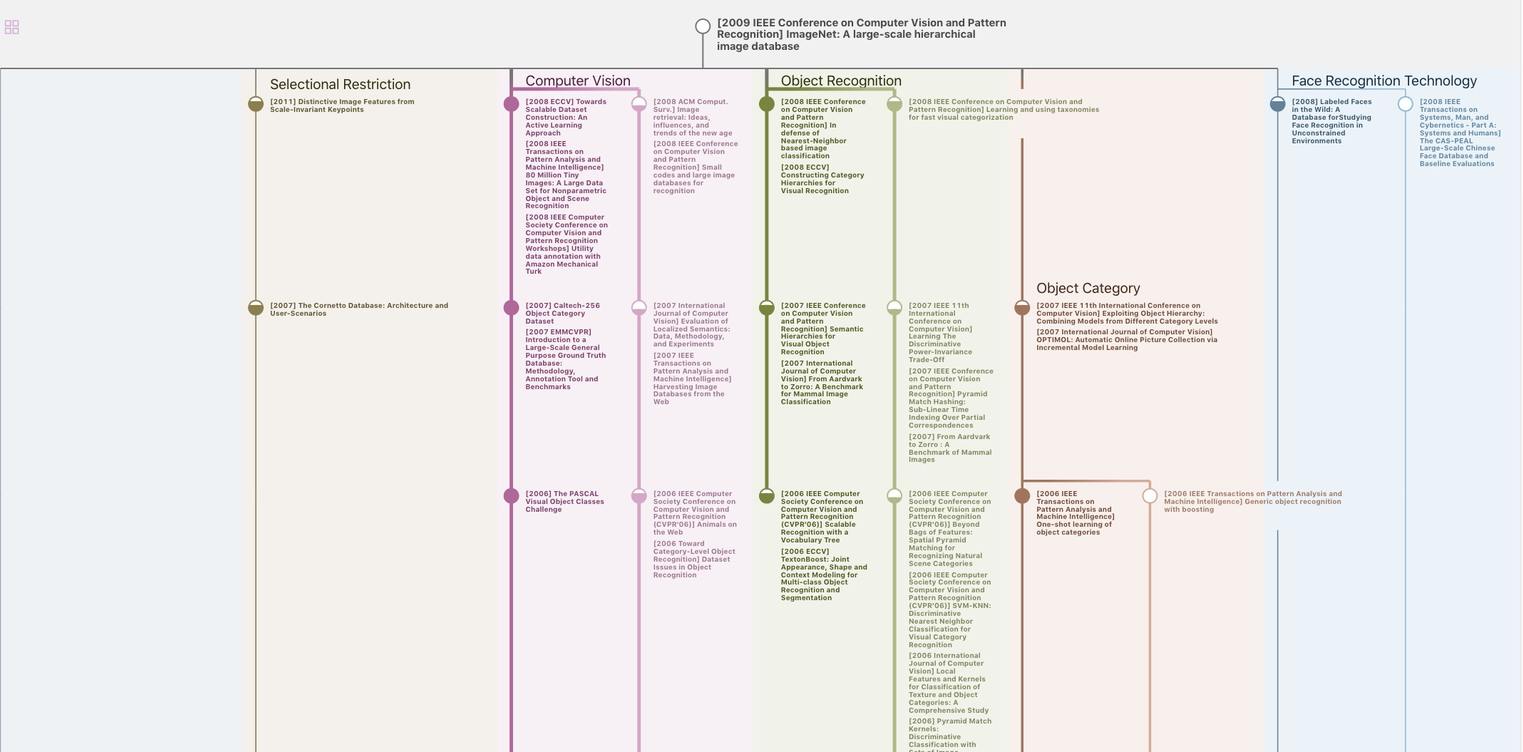
生成溯源树,研究论文发展脉络
Chat Paper
正在生成论文摘要