AMGAN: An Attribute-Matched Generative Adversarial Network for UAV Virtual Sample Generation
NEURAL PROCESSING LETTERS(2023)
摘要
The recognition and detection of unmanned aerial vehicles (UAV) usually face the difficulty of insufficient samples. Given a limited number of real UAV images, it is a challenging task to generate virtual UAV images to enrich both the diversity and quantity of training samples. Aiming at this problem, we propose a novel attribute-matched generative adversarial network (AMGAN) that can migrate a UAV object from a single background to a complex background. AMGAN consists of three parts: the basic network, the pairing network, and the background constraint. Image attributes are first disentangled and then reorganized by the basic network, which is prone to attribute collapse. Then the pairing network introduces attribute-level discriminators to make the same-type attributes match each other correctly. Furthermore, the background constraint is added to guide model convergence and eliminate the attribute residue problem. Qualitative experimental results show that AMGAN can generate a large number of high-fidelity virtual UAV images in various backgrounds. Quantitative experimental results on small-scale datasets demonstrate that when these generated images are used for data augmentation, both the diversity and quantity of samples can be greatly increased, boosting the UAV recognition performance.
更多查看译文
关键词
Generative adversarial networks,Unmanned aerial vehicles,Virtual sample generation,Data augmentation,Attribute matching
AI 理解论文
溯源树
样例
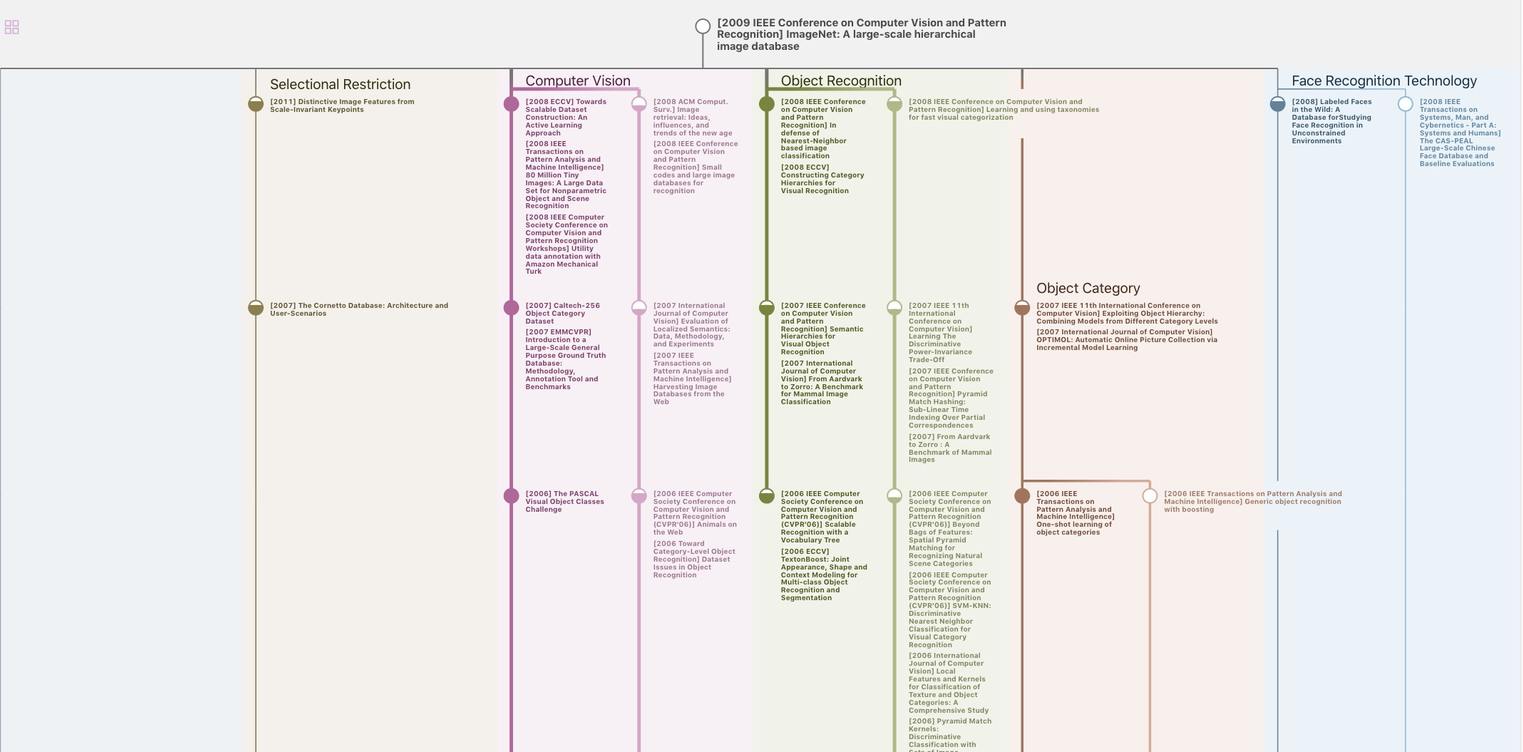
生成溯源树,研究论文发展脉络
Chat Paper
正在生成论文摘要