Investigating the Power of LSTM-Based Models in Solar Energy Forecasting
PROCESSES(2023)
摘要
Solar is a significant renewable energy source. Solar energy can provide for the world's energy needs while minimizing global warming from traditional sources. Forecasting the output of renewable energy has a considerable impact on decisions about the operation and management of power systems. It is crucial to accurately forecast the output of renewable energy sources in order to assure grid dependability and sustainability and to reduce the risk and expense of energy markets and systems. Recent advancements in long short-term memory (LSTM) have attracted researchers to the model, and its promising potential is reflected in the method's richness and the growing number of papers about it. To facilitate further research and development in this area, this paper investigates LSTM models for forecasting solar energy by using time-series data. The paper is divided into two parts: (1) independent LSTM models and (2) hybrid models that incorporate LSTM as another type of technique. The Root mean square error (RMSE) and other error metrics are used as the representative evaluation metrics for comparing the accuracy of the selected methods. According to empirical studies, the two types of models (independent LSTM and hybrid) have distinct advantages and disadvantages depending on the scenario. For instance, LSTM outperforms the other standalone models, but hybrid models generally outperform standalone models despite their longer data training time requirement. The most notable discovery is the better suitability of LSTM as a predictive model to forecast the amount of solar radiation and photovoltaic power compared with other conventional machine learning methods.
更多查看译文
关键词
solar irradiance forecasting,renewable energy,photovoltaic power forecasting,long short-term memory,hybrid model,deep learning
AI 理解论文
溯源树
样例
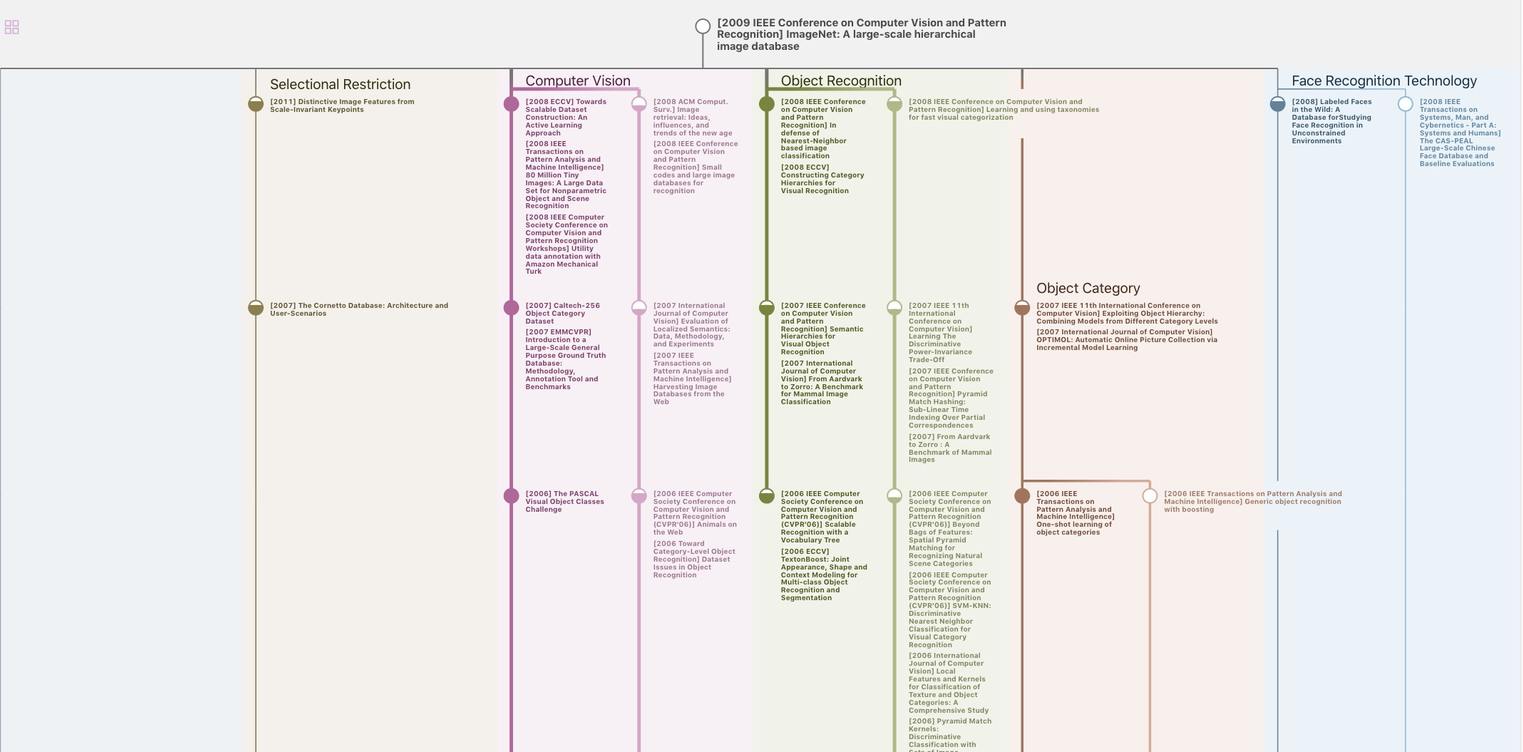
生成溯源树,研究论文发展脉络
Chat Paper
正在生成论文摘要