SMGNN: span-to-span multi-channel graph neural network for aspect-sentiment triplet extraction
JOURNAL OF INTELLIGENT INFORMATION SYSTEMS(2023)
摘要
Extracting Aspect Sentiment Triplets is a relatively new method for conducting sentiment analysis. The triplet extraction is carried out in an end-to-end way by more recent models; however, they focus a significant amount of emphasis on the interactions between each target word and opinion word. Thus, they are not capable of achieving their goals or expressing thoughts that are comprised of more than one word. More recently, In addition, there are attempts to develop a span-based method that can deal with thoughts comprised of more than one word. However, the spans are built based on the sequential information between words ignoring the syntactic structures and semantic correlations between words. We propose a Span-to-Span Multi-channel Graph Neural Network (SMGCN) to address the above issues to extract aspect and opinion words in pairs. This will allow us to address the concerns that have been raised. When attempting to anticipate the emotional connection that exists between targets and opinions, the method that we have proposed takes into account the interaction that exists between the entire span of targets and opinions. The model leverages both syntactic structures and semantic correlations to build span representations simultaneously. The model is able to make predictions based on the semantics of full spans with more consistency regarding the sentiment. Our model outperforms the baselines to extract the sentiment triplets, as shown by experimental findings obtained from three different publicly available datasets.
更多查看译文
关键词
Extracting aspect sentiment triplets,Graph neural network,Sentiment analysis,Aspect-opinion pair extraction
AI 理解论文
溯源树
样例
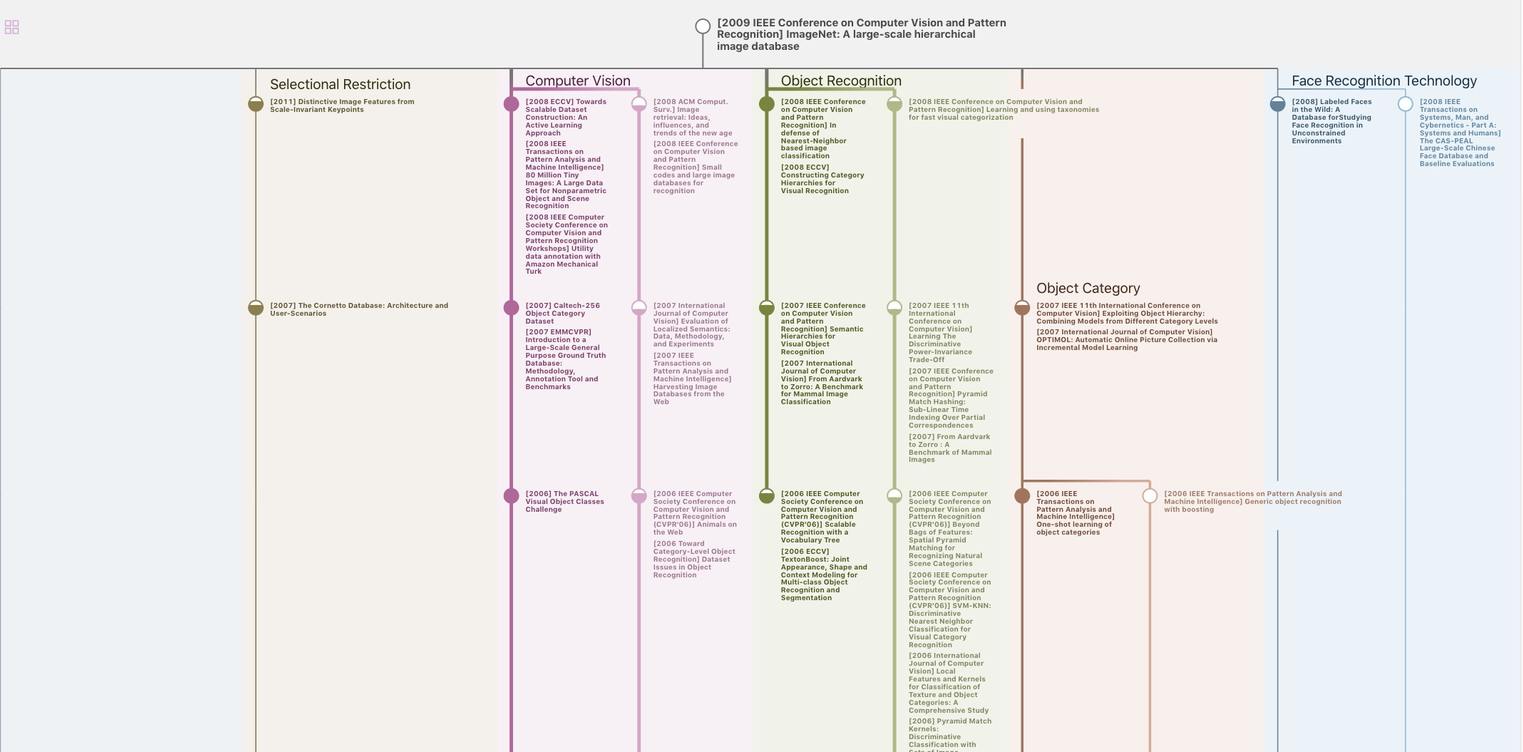
生成溯源树,研究论文发展脉络
Chat Paper
正在生成论文摘要