SHFW: second-order hybrid fusion weight–median algorithm based on machining learning for advanced IoT data analytics
WIRELESS NETWORKS(2023)
摘要
IoT applications have greatly improved the convenience of our lives, generating large amounts of IoT data. How to efficiently use these data to feed IoT applications has become a significant research problem, and many studies have used machine learning methods for advanced IoT data analytics. However, the prediction models of these studies generally suffer from the problem of easy overfitting, and insufficient work done on the analysis and selection of features. In terms of prediction models, this paper proposes a second-order hybrid fusion weight–median (W–M) algorithm, which first ensures the sensitivity of the data by weighted average fusion and then reduces the impact of extreme values on the data by median fusion. In terms of feature analysis and selection, this study constructed feature crosses by studying time and weather factors and proposes a second-order feature selection, recursive feature elimination-cross validation-Pearson correlation coefficient (RFECV-PCC) algorithm, which combines the RFECV method and Pearson correlation coefficient for feature selections. Experiments were conducted to evaluate the prediction results using root mean square logarithmic error (RMSLE) and comparison of the simulation results of the dataset, which the real-time data measurement showed that the W–M algorithm has the strongest RMSLE generalization ability, further improving the prediction accuracy after feature selection by the RFECV-PCC algorithm.
更多查看译文
关键词
hybrid fusion weight–median,weight–median algorithm,machining learning,second-order
AI 理解论文
溯源树
样例
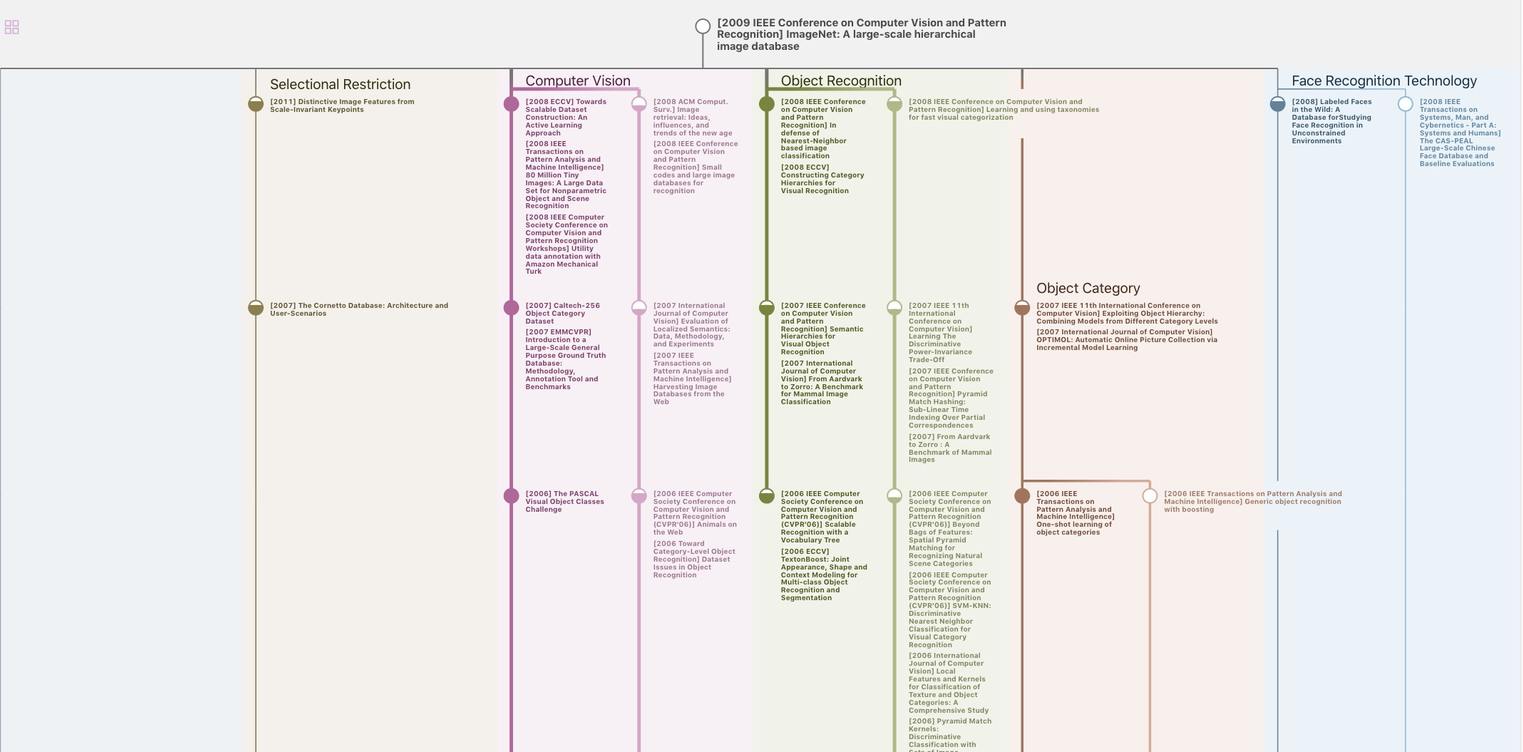
生成溯源树,研究论文发展脉络
Chat Paper
正在生成论文摘要