Large-scale, batch-effect-free augmented quantitative phase imaging by generative learning
HIGH-SPEED BIOMEDICAL IMAGING AND SPECTROSCOPY VIII(2023)
摘要
We present an unprecedented, generative deep learning model (named beGAN) in reconstructing batch-effect-free quantitative phase image (QPI). By employing the high-throughput microfluidic multimodal imaging flow cytometry platform (i.e. multi-ATOM), our model demonstrated a robust QPI prediction from brightfield on various lung cancer cell lines (>800,000 cells). With batch-free QPI, biophysical phenotypes of cells are unified across batches and a significant improvement from 33.61% to 91.34% is achieved on the cross-batches cancer cell lines classification. This work unveil an avenue on overcoming batch effect with deep learning at single-cell imaging level.
更多查看译文
AI 理解论文
溯源树
样例
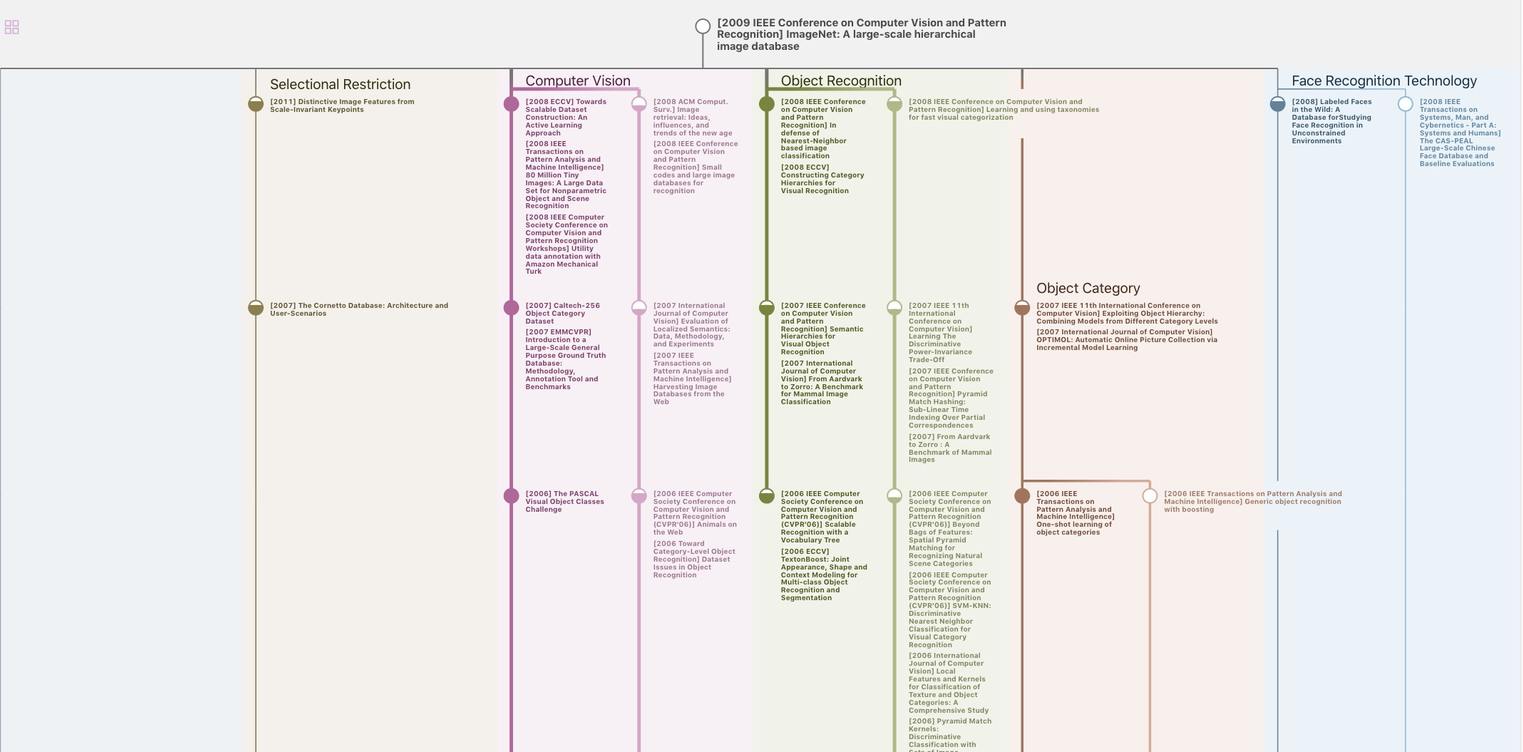
生成溯源树,研究论文发展脉络
Chat Paper
正在生成论文摘要