Efficient and scalable DBSCAN framework for clustering continuous trajectories in road networks.
Int. J. Geogr. Inf. Sci.(2023)
摘要
Clustering the trajectories of vehicles moving on road networks is a key data mining technique for understanding human mobility patterns, as well as their interactions with urban environments. The development of efficient and scalable trajectory clustering algorithms, however, still faces challenges because of the computational costs when measuring similarities among a large number of network-constrained trajectories. To address this problem, a novel trajectory clustering framework based on the well-developed Density-Based Spatial Clustering of Applications with Noise (DBSCAN) approach is proposed. This proposed framework accurately quantifies similarities using a trajectory representation of continuous polylines in the space and time dimensions, and does not require trajectory discretization. Further, the proposed framework utilizes the space-time buffering concept to formulate e-neighborhood queries that directly retrieve the e-neighbors of trajectories and thus avoids computing a trajectory similarity matrix. State-of-the-art trajectory databases and index structures are incorporated to further improve trajectory clustering performance. A comprehensive case study was carried out using an open dataset of 20,161 trajectories. Results show that the proposed framework efficiently executed trajectory clustering on the large test dataset within 3 min. This was approximately 2,700 times faster than existing DBSCAN algorithms.
更多查看译文
关键词
Network-constrained trajectory,trajectory clustering,space-time buffering,spatiotemporal big data,transport informatics
AI 理解论文
溯源树
样例
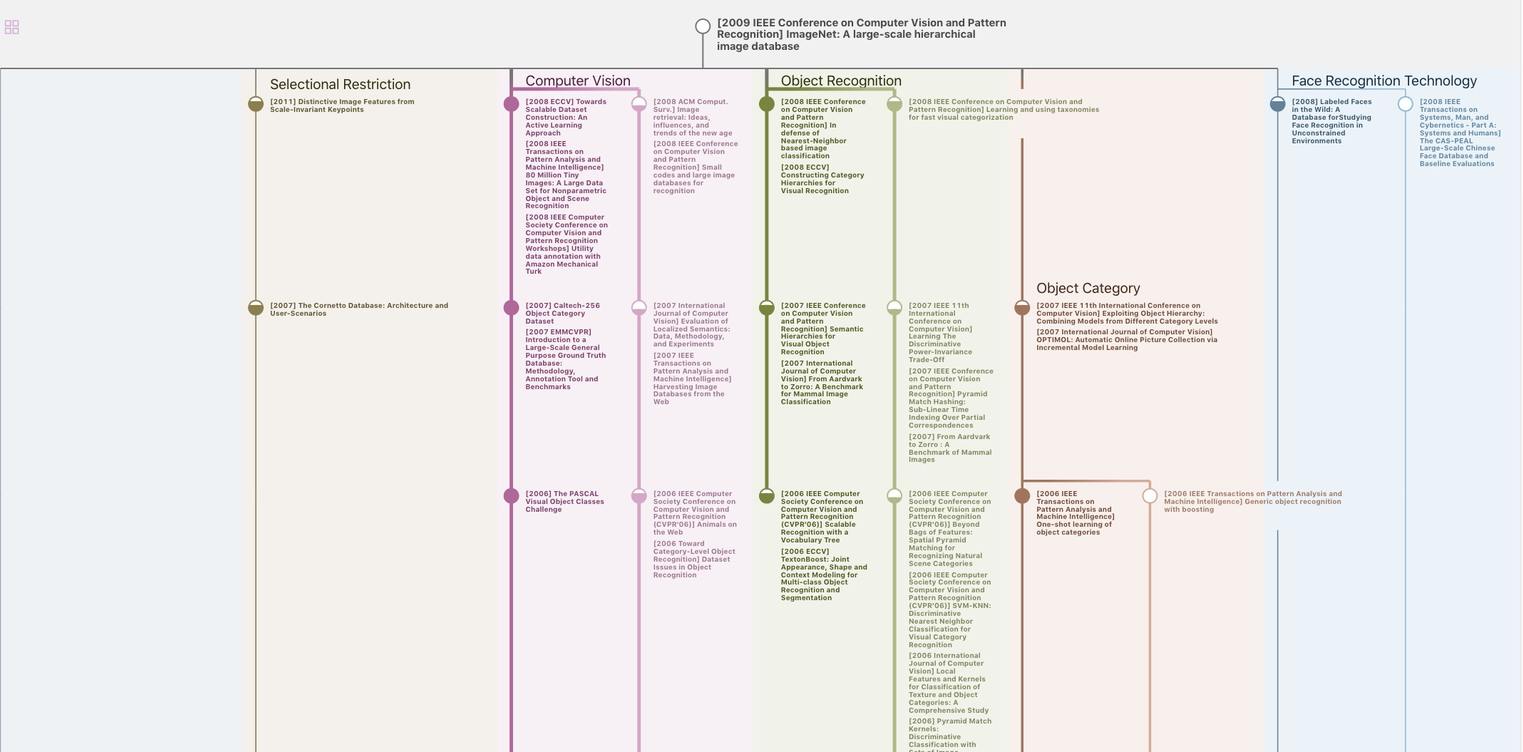
生成溯源树,研究论文发展脉络
Chat Paper
正在生成论文摘要