Deep Ring-Block-Wise Network for Hyperspectral Image Classification.
IEEE transactions on neural networks and learning systems(2023)
摘要
Deep learning has achieved many successes in the field of the hyperspectral image (HSI) classification. Most of existing deep learning-based methods have no consideration of feature distribution, which may yield lowly separable and discriminative features. From the perspective of spatial geometry, one excellent feature distribution form requires to satisfy both properties, i.e., block and ring. The block means that in a feature space, the distance of intraclass samples is close and the one of interclass samples is far. The ring represents that all class samples are overall distributed in a ring topology. Accordingly, in this article, we propose a novel deep ring-block-wise network (DRN) for the HSI classification, which takes full consideration of feature distribution. To obtain the good distribution used for high classification performance, in this DRN, a ring-block perception (RBP) layer is built by integrating the self-representation and ring loss into a perception model. By such way, the exported features are imposed to follow the requirements of both block and ring, so as to be more separably and discriminatively distributed compared with traditional deep networks. Besides, we also design an optimization strategy with alternating update to obtain the solution of this RBP layer model. Extensive results on the Salinas, Pavia Centre, Indian Pines, and Houston datasets have demonstrated that the proposed DRN method achieves the better classification performance in contrast to the state-of-the-art approaches.
更多查看译文
关键词
Block,deep ring-block-wise network (DRN),hyperspectral image (HSI) classification,ring
AI 理解论文
溯源树
样例
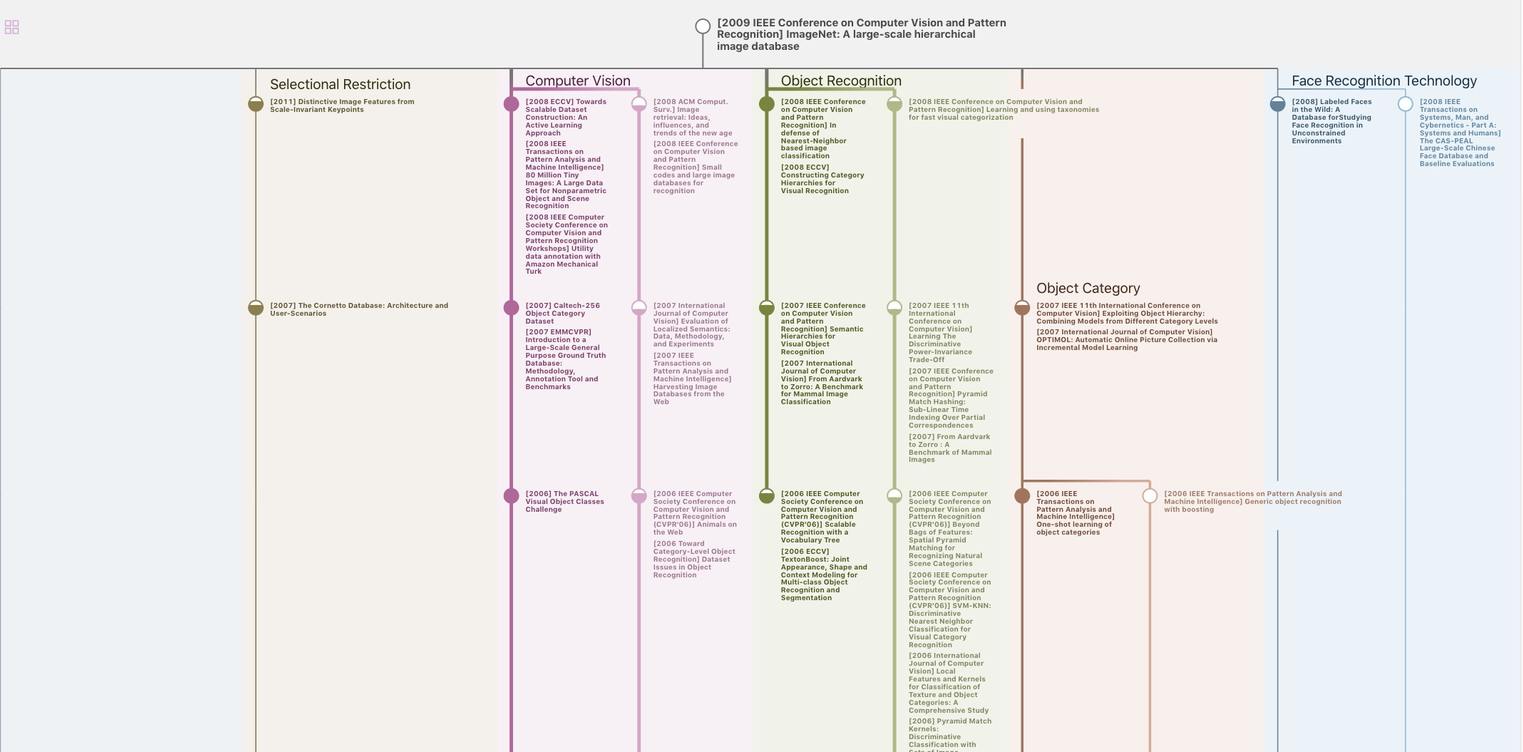
生成溯源树,研究论文发展脉络
Chat Paper
正在生成论文摘要