One-Step Multiview Fuzzy Clustering With Collaborative Learning Between Common and Specific Hidden Space Information.
IEEE transactions on neural networks and learning systems(2023)
摘要
Multiview data are widespread in real-world applications, and multiview clustering is a commonly used technique to effectively mine the data. Most of the existing algorithms perform multiview clustering by mining the commonly hidden space between views. Although this strategy is effective, there are two challenges that still need to be addressed to further improve the performance. First, how to design an efficient hidden space learning method so that the learned hidden spaces contain both shared and specific information of multiview data. Second, how to design an efficient mechanism to make the learned hidden space more suitable for the clustering task. In this study, a novel one-step multiview fuzzy clustering (OMFC-CS) method is proposed to address the two challenges by collaborative learning between the common and specific space information. To tackle the first challenge, we propose a mechanism to extract the common and specific information simultaneously based on matrix factorization. For the second challenge, we design a one-step learning framework to integrate the learning of common and specific spaces and the learning of fuzzy partitions. The integration is achieved in the framework by performing the two learning processes alternately and thereby yielding mutual benefit. Furthermore, the Shannon entropy strategy is introduced to obtain the optimal views weight assignment during clustering. The experimental results based on benchmark multiview datasets demonstrate that the proposed OMFC-CS outperforms many existing methods.
更多查看译文
关键词
Clustering methods,Learning systems,Clustering algorithms,Data mining,Collaboration,Feature extraction,Partitioning algorithms,Common space,fuzzy clustering,multiview data,one-step clustering,specific space
AI 理解论文
溯源树
样例
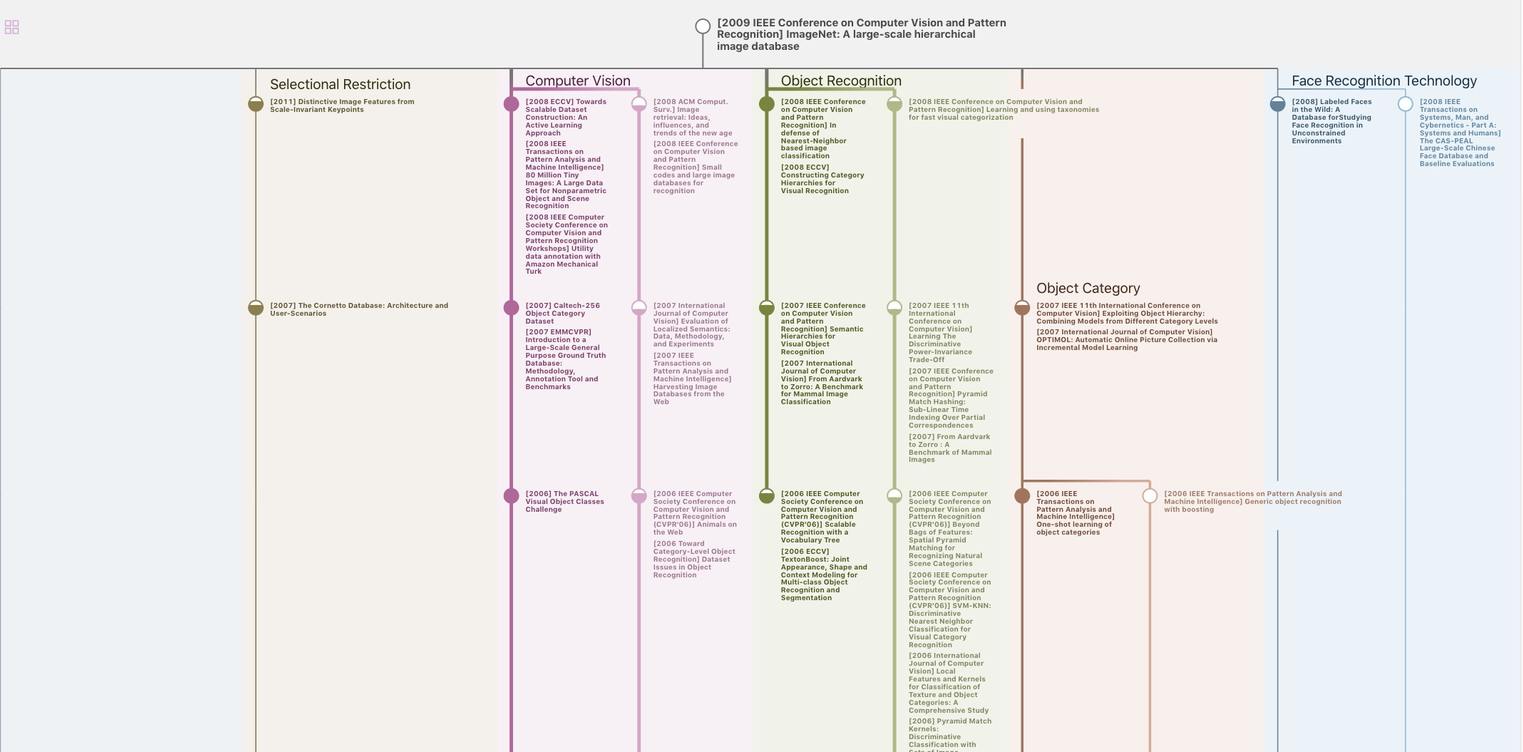
生成溯源树,研究论文发展脉络
Chat Paper
正在生成论文摘要