Generating complete synthetic datasets for high-resolution amplitude-versus-offset attributes deep learning inversion
GEOPHYSICAL PROSPECTING(2023)
摘要
Deep learning has been used in seismic exploration to solve seismic inversion problems, however it requires sufficient and diverse training samples and labels to obtain satisfactory results. Insufficient training labels are a common problem since labels usually come from well-logging data, which are limited and sparsely distributed. This can result in a trained network with poor generalizability. A novel complete synthetic dataset-driven method utilizing convolutional neural network is presented for seismic amplitude-versus-offset attributes P and G inversion. Gaussian simulation sampling with physical constraints is used to generate a complete elastic parameter dataset by traversing the entire elastic parameter model space. By randomly combining elastic parameters from the full elastic parameters model space, sufficient synthetic pre-stack amplitude-versus-offset gathers and attribute datasets are generated for training the convolutional neural network. Compared with the limited real data-driven convolutional neural network, the complete synthetic dataset-driven convolutional neural network has better generalizability. Broadband training labels improve the accuracy and resolution of the complete synthetic dataset-driven convolutional neural network's inversion results beyond that of the conventional least-square data-fitting method. The complete synthetic dataset-driven convolutional neural network is robust for processing noise-contaminated seismic data, but if the frequency band of the labels for training the network is too wide and the signal-to-noise ratio of pre-stack amplitude-versus-offset gathers is too low, the quality of the inversion results will reduce. The Marmousi II model and field data examples show that the novel complete synthetic dataset-driven convolutional neural network can extract higher-resolution amplitude-versus-offset attributes P and G.
更多查看译文
关键词
AVO attributes inversion, complete synthetic datasets, deep learning
AI 理解论文
溯源树
样例
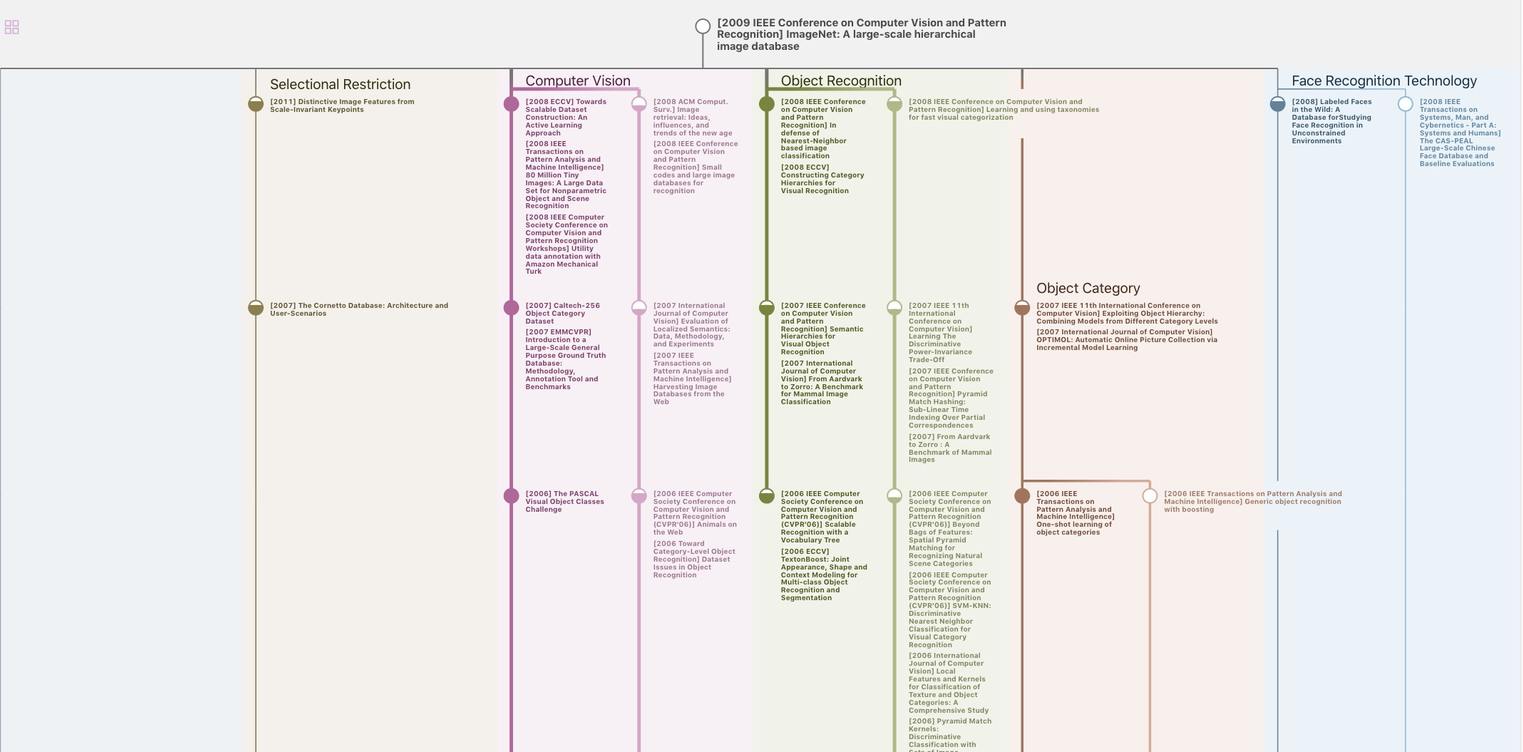
生成溯源树,研究论文发展脉络
Chat Paper
正在生成论文摘要