Simultaneous Dimension Reduction and Variable Selection for Multinomial Logistic Regression
INFORMS JOURNAL ON COMPUTING(2023)
摘要
Multinomial logistic regression is a useful model for predicting the probabilities of multiclass outcomes. Because of the complexity and high dimensionality of some data, it is challenging to fit a valid model with high accuracy and interpretability. We propose a novel sparse reduced-rank multinomial logistic regression model to jointly select variables and reduce the dimension via a nonconvex row constraint. We develop a block-wise iterative algorithm with a majorizing surrogate function to efficiently solve the optimization problem. From an algorithmic aspect, we show that the output estimator enjoys consistency in estimation and sparsity recovery even in a high-dimensional setting. The finite sample performance of the proposed method is investigated via simulation studies and two real image data sets. The results show that our proposal has competitive performance in both estimation accuracy and computation time.
更多查看译文
关键词
high-dimensional data,multinomial logistic regression,reduced-rank regression,variable selection
AI 理解论文
溯源树
样例
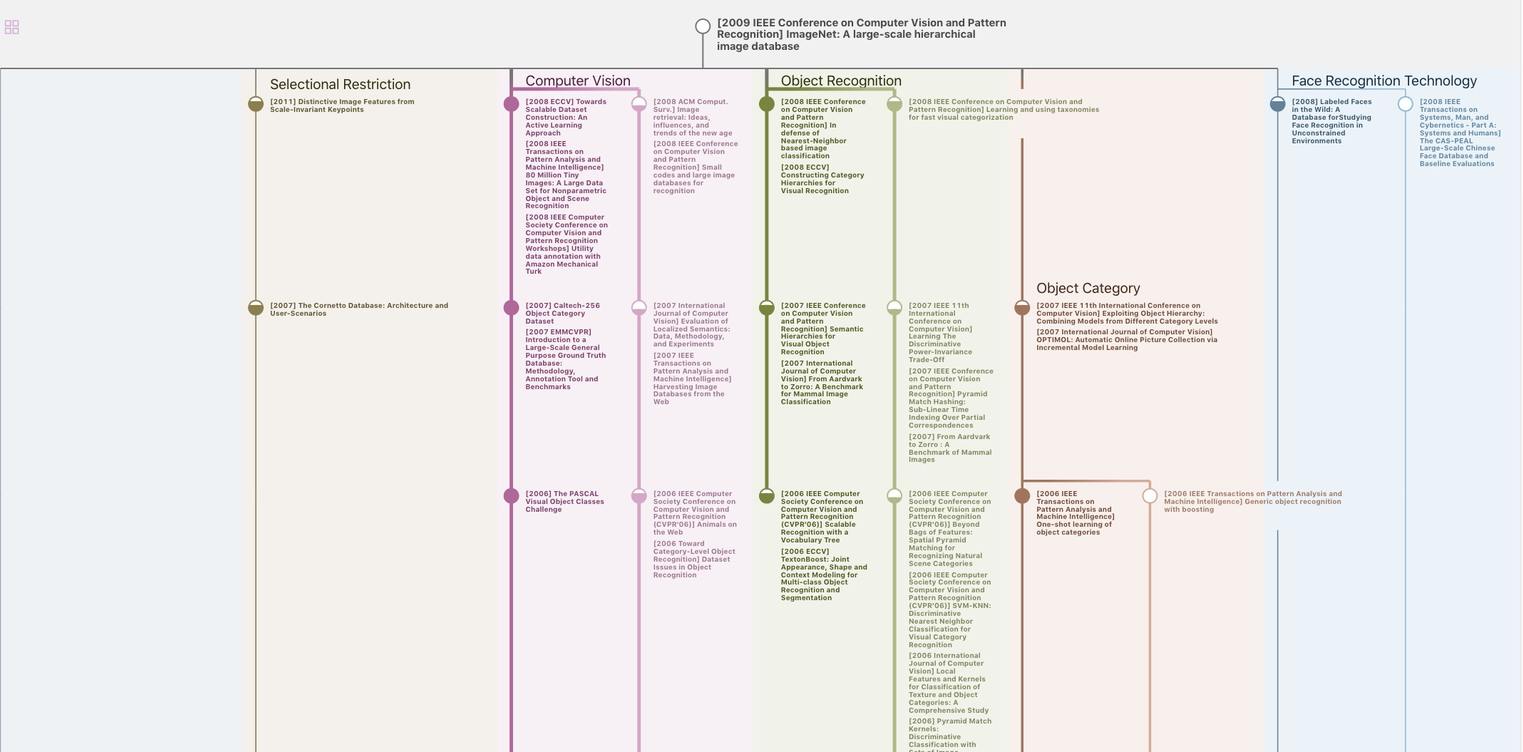
生成溯源树,研究论文发展脉络
Chat Paper
正在生成论文摘要