EEDD: Edge-Guided Energy-Based PCB Defect Detection
ELECTRONICS(2023)
摘要
Printed circuit board (PCB) defect detection is critical for ensuring the safety of electronic devices, especially in the space industry. Non-reference-based methods, typically the deep learning methods, suffer from a large amount of annotated data requirements and poor interpretability. In contrast, conventional reference-based methods achieve higher detection accuracy by comparing with a template image but rely on precise image alignment and face the challenge of fine defects detection. To solve the problem, we propose a novel Edge-guided Energy-based PCB Defect Detection method (EEDD). We focus on the salient edge characteristic of PCB images and regard the functional defects as contour differences and then propose a novel energy measurement method for PCB contour anomaly detection. We introduce the energy transformation using the edge information of the template and test image, then Speeded-Up Robust Features method (SURF) is used for image alignment, and finally achieve defect detection by measuring the energy anomaly score pixel by pixel with the proposed energy-based defect localization and contour flood fill methods. Our method excels in detecting multi-scale defects, particularly tiny defects, and is robust against interferences such as non-finely aligned images and edge spurs. Experiments on the DeepPCB-A dataset and our high-resolution PCB dataset (HDPCB) show that the proposed method outperforms state-of-the-art methods in PCB defect-detection tasks.
更多查看译文
关键词
printed circuit board,tiny defect detection,edge feature,energy transformation,energy anomaly score,contour flood fill
AI 理解论文
溯源树
样例
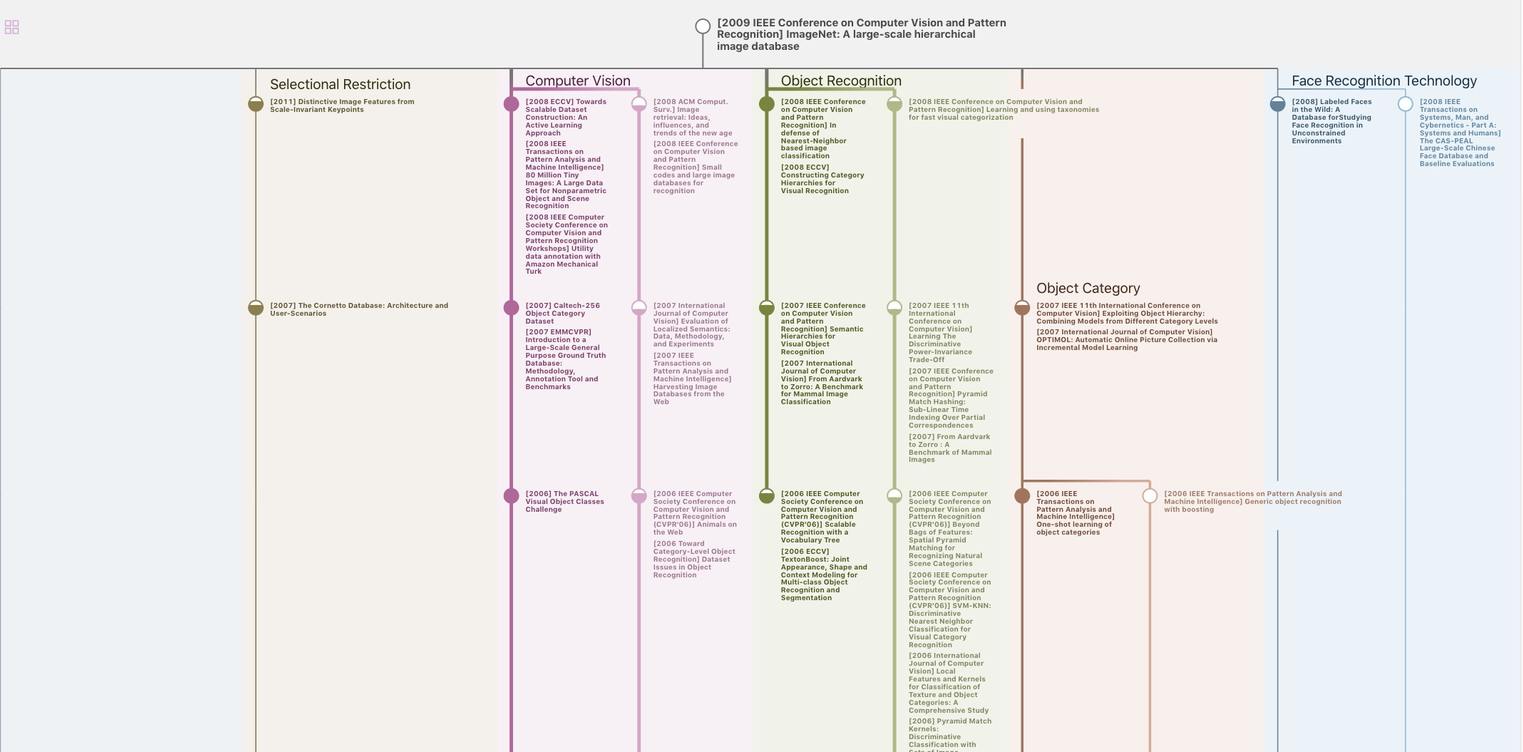
生成溯源树,研究论文发展脉络
Chat Paper
正在生成论文摘要