PM2.5 forecasting based on transformer neural network and data embedding
EARTH SCIENCE INFORMATICS(2023)
摘要
Forecasting time series data is a big challenge due to the temporal and multivariate dependencies in the data. In this paper, we present a new approach named as TPPM25 (Transformer-based Prediction of PM2.5) for forecasting PM2.5, a key air quality indicator. It is based on the state-of-the-art Transformer neural network and various data embedding techniques. By performing attention calculations among features over time steps, TPPM25 mimics cognitive attention and selectively enhances essential parts of the input data while diminishing other parts. TPPM25 is able to effectively capture temporal relations to multiple influencing meteorological features. Experiments demonstrate its effectiveness by comparing with a cutting-edge ensemble deep learning model from Zhang et al. (Inf Sci 544:427-445, 2021). Our TPPM25 model outperforms Zhang et al.'s model under the same experimental setting on a well-researched benchmark dataset. As Zhang et al.'s model is restricted to univariate PM2.5 prediction, our TPPM25 model bypasses this restriction and further improves the prediction accuracy when considering more influencing meteorological features. Moreover, our TPPM25 model is able to maintain high prediction accuracy over longer periods of time as compared to the Long-Short Term Memory (LSTM) and Bidirectional LSTM models.
更多查看译文
关键词
PM2.5 forecasting, Transformer neural networks, Time series analysis, Air pollution
AI 理解论文
溯源树
样例
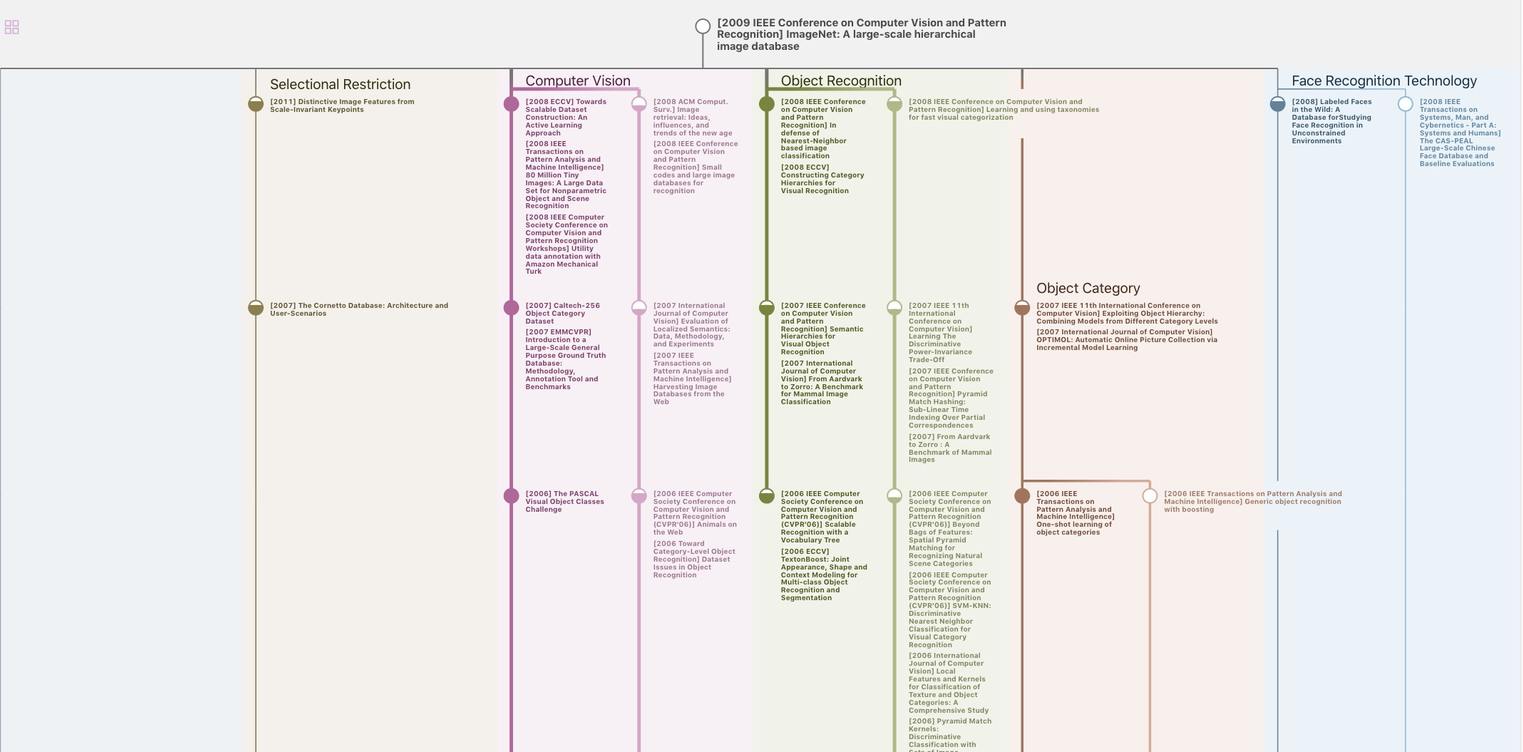
生成溯源树,研究论文发展脉络
Chat Paper
正在生成论文摘要