Chained Gaussian processes to estimate battery health degradation with uncertainties
JOURNAL OF ENERGY STORAGE(2023)
摘要
Estimating the average health degradation of a new battery design is a crucial objective for manufacturers to estimate its value. Furthermore, to quantify financial risks, associated uncertainties should be modeled precisely. From a data-driven perspective, Gaussian process regression (GPR) is often a method of choice since it simultaneously learns complex models and naturally includes uncertainties. However, GPR methods generally rely on a stationarity assumption which imposes severe constraints on uncertainties. In this paper, we illustrate the limits of standard GPR and show that the Chained Gaussian processes, a more general framework introduced in the Machine learning community, is a useful alternative, allowing more accurate quantification of uncertainties.
更多查看译文
关键词
Lithium-ion batteries,Uncertainties,Cell-to-cell variations,Gaussian process regression,Stationarity,Chained Gaussian processes
AI 理解论文
溯源树
样例
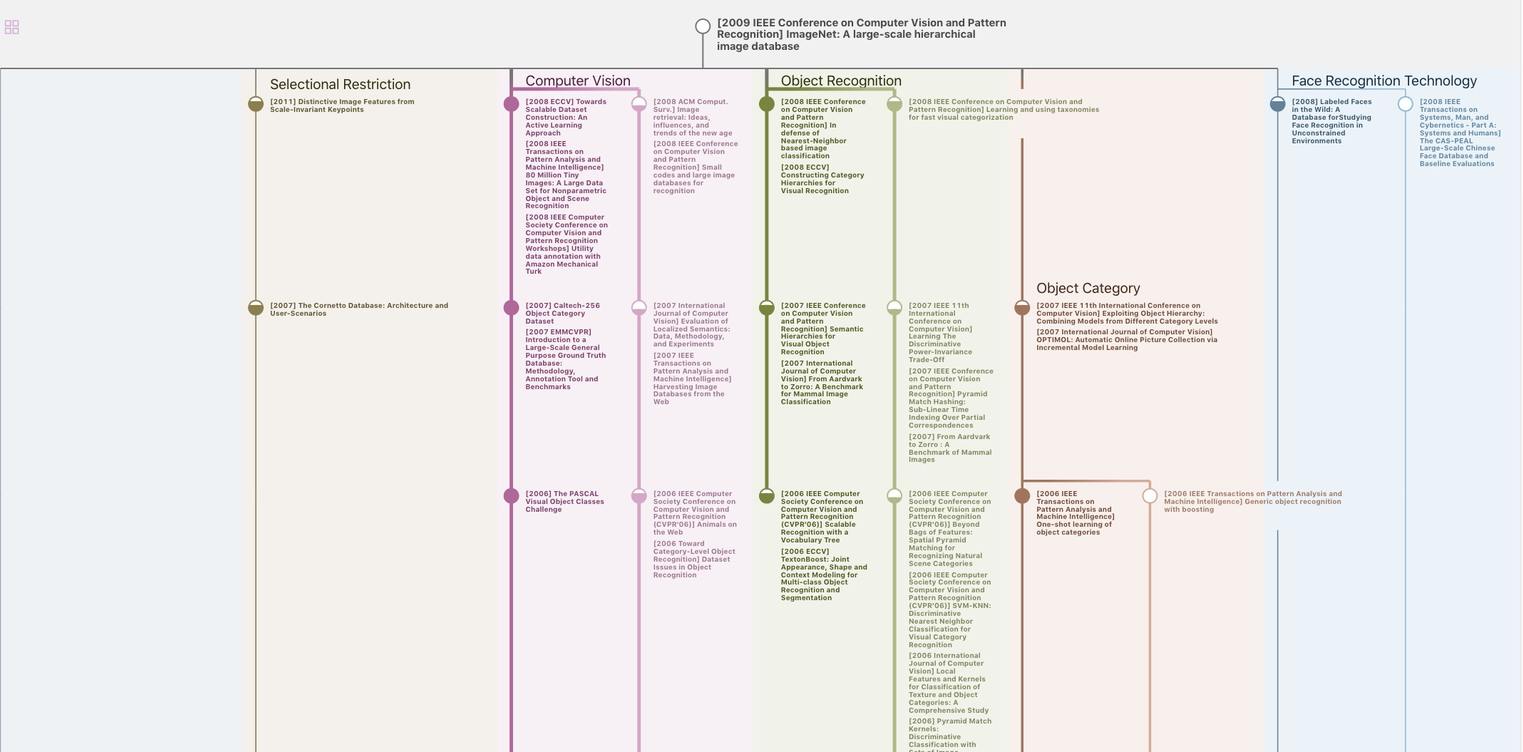
生成溯源树,研究论文发展脉络
Chat Paper
正在生成论文摘要