Spatiotemporally continuous estimates of daily 1-km PM2.5 concentrations and their long-term exposure in China from 2000 to 2020
JOURNAL OF ENVIRONMENTAL MANAGEMENT(2023)
摘要
Monitoring long-term variations in fine particulate matter (PM2.5) is essential for environmental management and epidemiological studies. While satellite-based statistical/machine-learning methods can be used for esti-mating high-resolution ground-level PM2.5 concentration data, their applications have been hindered by limited accuracy in daily estimates during years without PM2.5 measurements and massive missing values due to satellite retrieval data. To address these issues, we developed a new spatiotemporal high-resolution PM2.5 hindcast modeling framework to generate the full-coverage, daily, 1-km PM2.5 data for China for the period 2000-2020 with improved accuracy. Our modeling framework incorporated information on changes in observation variables between periods with and without monitoring data and filled gaps in PM2.5 estimates induced by satellite data using imputed high-resolution aerosol data. Compared to previous hindcast studies, our method achieved su-perior overall cross-validation (CV) R2 and root-mean-square error (RMSE) of 0.90 and 12.94 mu g/m3 and significantly improved the model performance in years without PM2.5 measurements, raising the leave-one-year-out CV R2 [RMSE] to 0.83 [12.10 mu g/m3] at a monthly scale (0.65 [23.29 mu g/m3] at a daily scale). Our long-term PM2.5 estimates show a sharp decline in PM2.5 exposure in recent years, but the national exposure level in 2020 still exceeded the first annual interim target of the 2021 World Health Organization air quality guidelines. The proposed hindcast framework represents a new strategy to improve air quality hindcast modeling and can be applied to other regions with limited air quality monitoring periods. These high-quality estimates can support both long-and short-term scientific research and environmental management of PM2.5 in China.
更多查看译文
关键词
concentrations,exposure,long-term
AI 理解论文
溯源树
样例
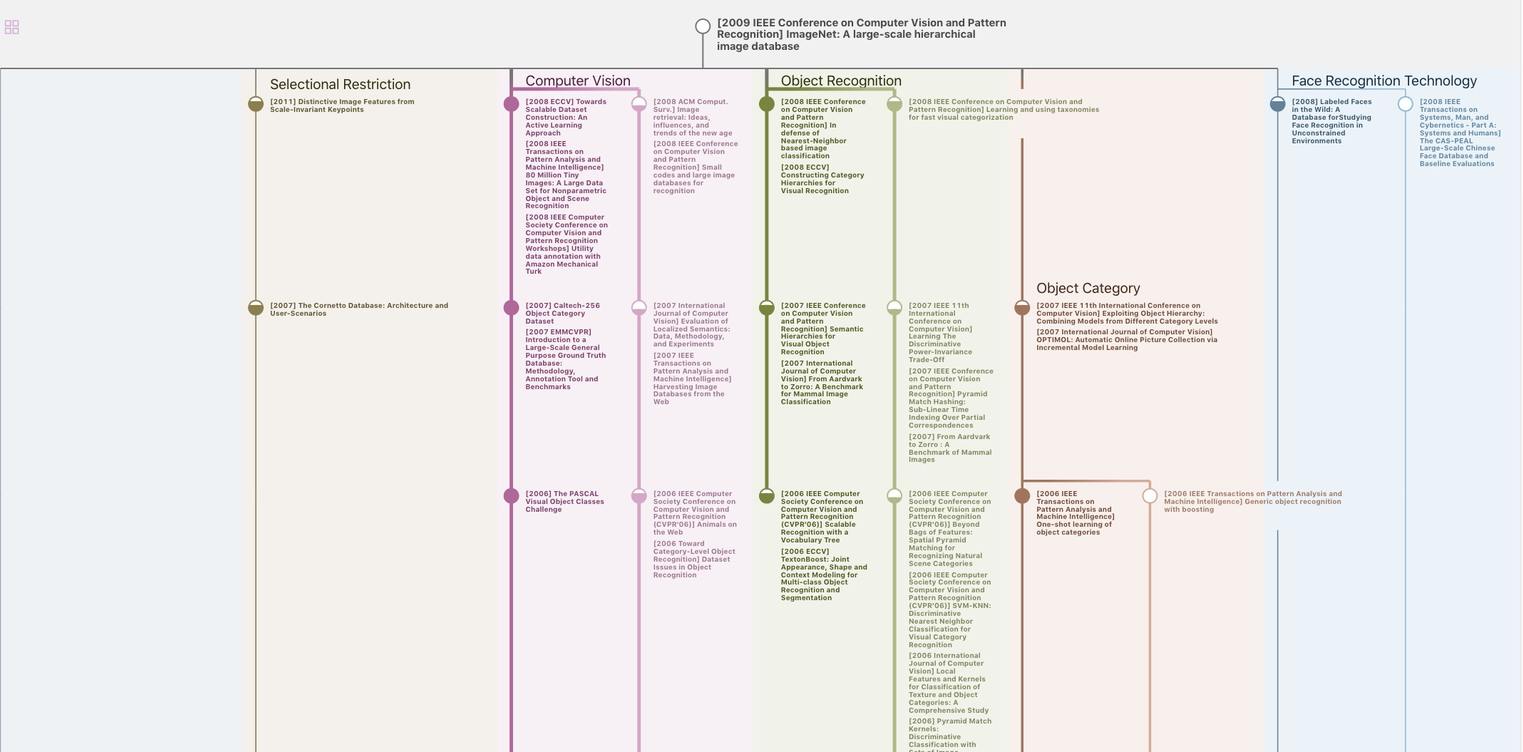
生成溯源树,研究论文发展脉络
Chat Paper
正在生成论文摘要