Joint Confidence Region Approach to Ranking Hotspot Locations Considering Uncertainty in Expected Risk Estimates
TRANSPORTATION RESEARCH RECORD(2024)
摘要
Network screening or crash hotspot identification is an essential task of all road safety improvement programs. The most common approach to network screening is to use statistical models to predict the expected risk at the locations of interest and then rank them accordingly. The predicted risk used for ranking is mostly in the form of point estimates, without any consideration of the inherent uncertainty with the estimates, which could lead to identifying a wrong list of crash hotspots. This study aims to fill this research gap by employing a frequentist approach to finding a joint confidence region of risk for ranking locations and identification of hotspots. A case study on three-legged minor approach stop-controlled intersections in Kitchener, Ontario, is conducted to illustrate the proposed approach. Crash risk is modeled using a combination of a hierarchical full Bayesian negative binomial model and a multinomial logit model, which are then used to estimate the 95% confidence interval of the expected risk. For each location, the confidence region of rankings is obtained on the basis of the expected risk estimates. The results show that considering uncertainty in the crash hotspot identification process can lead to varied ranking positions for each location. In fact, considering uncertainty, the true value of the estimated crash risk is unknown. By quantifying uncertainty, it can be concluded that the true value of the estimated risk follows a distribution with different probabilities. As a result, consideration of uncertainty in the road safety analysis may help to identify hotspots more accurately.
更多查看译文
关键词
network screening,safety performance functions,uncertainty quantification,crash risk modeling,confidence interval,hierarchical full Bayesian model,multinomial logit model
AI 理解论文
溯源树
样例
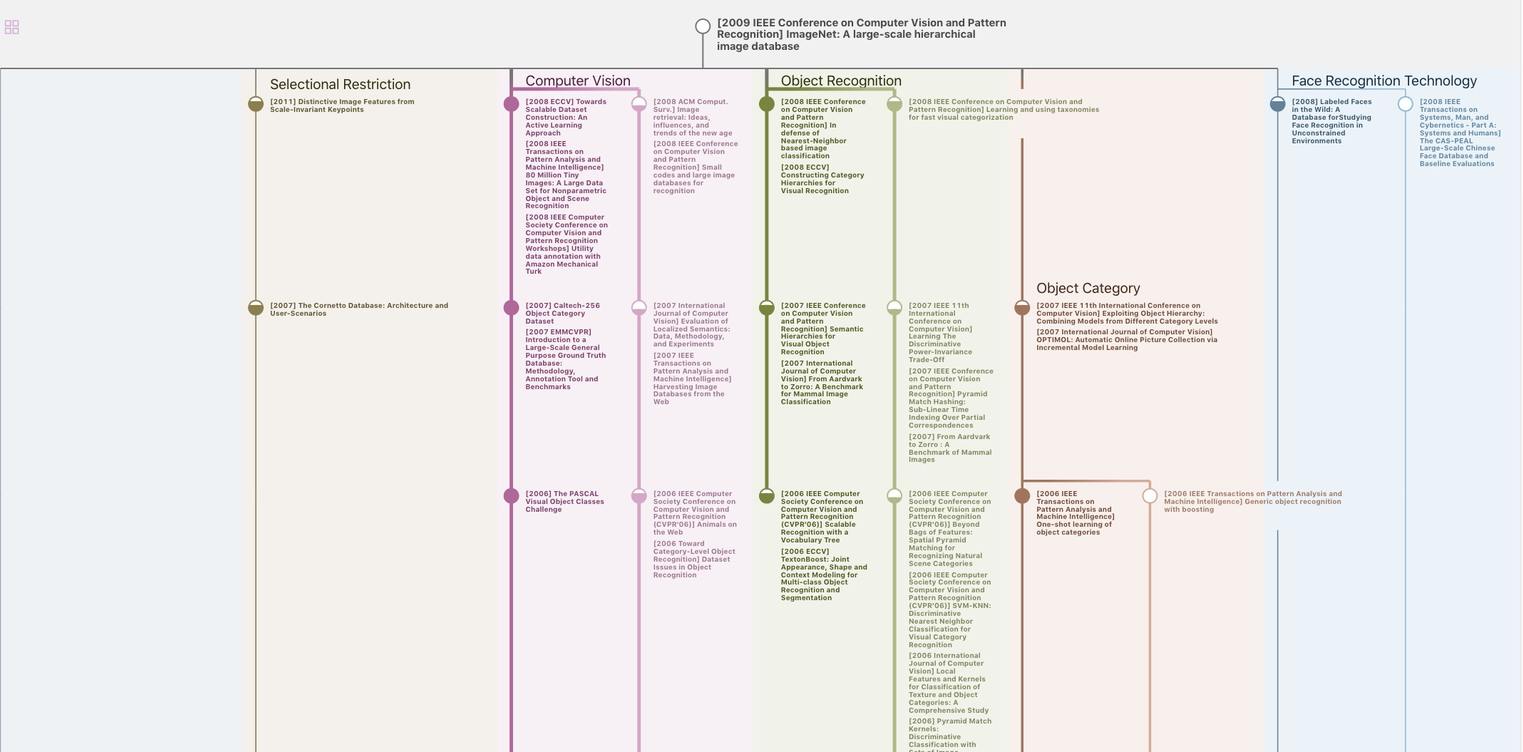
生成溯源树,研究论文发展脉络
Chat Paper
正在生成论文摘要