A machine learning approach for mapping surface urban heat island using environmental and socioeconomic variables: a case study in a medium-sized Brazilian city
ENVIRONMENTAL EARTH SCIENCES(2023)
摘要
Smart cities must deal with climate change and find solutions to mitigate phenomena such as urban heat islands (UHI). The land surface temperature (LST) extracted from thermal images is a primary source of information to study UHI, characterizing the surface urban heat islands (SUHI). In addition to LST, environmental and socioeconomic variables have been adopted to explain the SUHI phenomenon. Although machine learning algorithms have potential in several areas, their application in the study of the contribution of these variables in the prediction of LST to characterize SUHI is still unknown. Therefore, the work proposes a machine learning approach to fill this gap. The LST was extracted from 15 Landsat 8 images from 2019 to 2021. Data on socioeconomic variables were obtained from the official demographic census, and environmental variables were extracted from Sentinel-2 and Planet images. Six algorithms were tested to assess the ability to estimate the LST based on the above-mentioned variables. The results showed that the Decision Tree algorithm had the best performance ( r = 0.96, MAE = 1.49 °C and RMSE = 1.88 °C), followed by Random Forest. In addition, the inclusion of all seasons of the year and socioeconomic variables was shown to be relevant to the results. The main contribution of this work is to verify if the algorithms can optimize the SUHI characterization process, analyzing the influence exerted by the studied variables. In the social sphere, the information produced can help urban planning in the construction of smart cities.
更多查看译文
关键词
urban heat island,mapping surface,machine learning approach,medium-sized
AI 理解论文
溯源树
样例
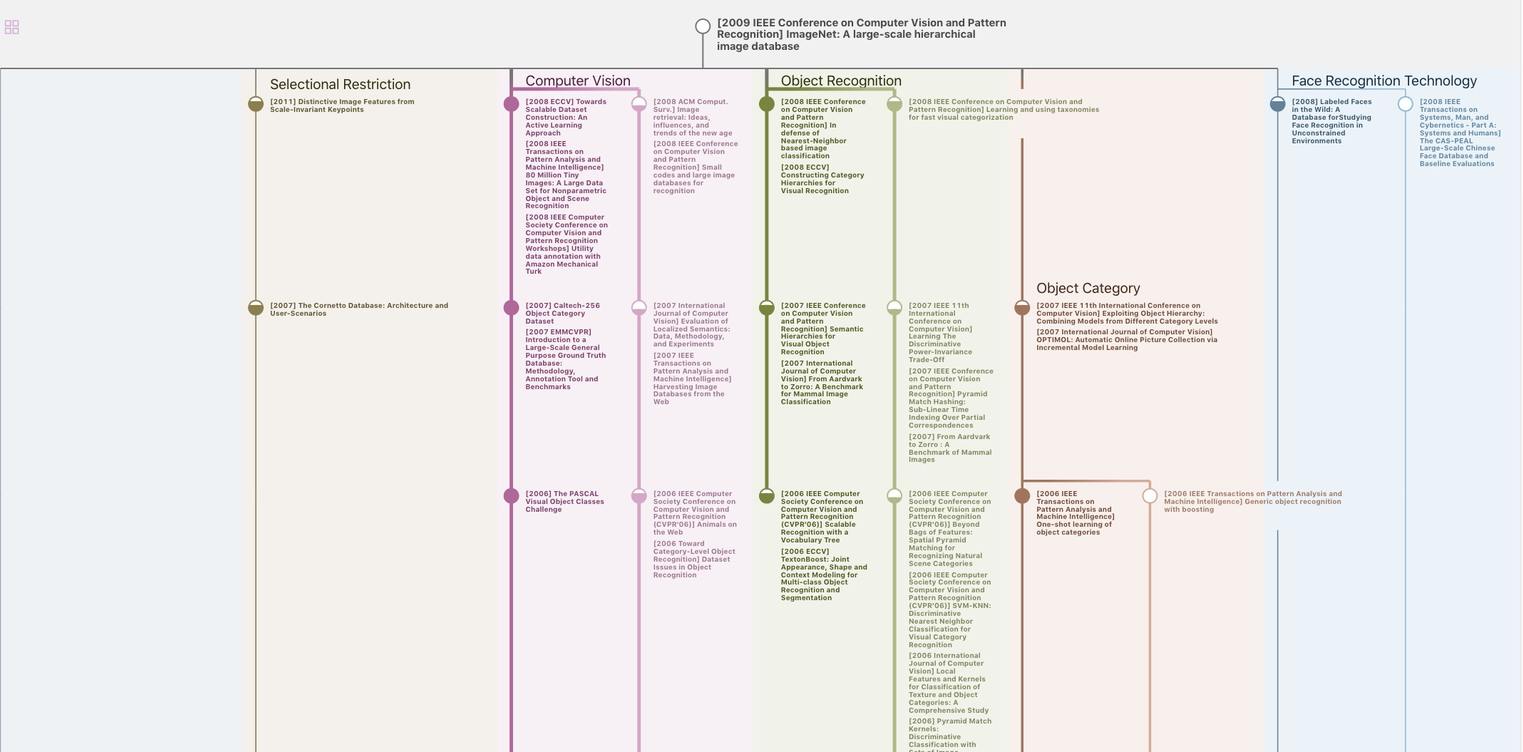
生成溯源树,研究论文发展脉络
Chat Paper
正在生成论文摘要