Convolutional Long Short-Term Memory Two-Dimensional Bidirectional Graph Convolutional Network for Taxi Demand Prediction
SUSTAINABILITY(2023)
摘要
With the rise of the online ride-hailing market, taxi demand prediction has received more and more research interest in intelligent transportation. However, most traditional research methods mainly focused on the demand based on the original point and ignored the intention of the passenger's destination. At the same time, many forecasting methods need sufficient investigation and data processing, which undoubtedly increases the complexity and operability of forecasting problems. Therefore, we regard the current taxi demand prediction as an origin-destination problem in order to provide more accurate predictions for the taxi demand problem. By combining a spatial network based on graph convolutional network (GCN) and a temporal network of convolutional long short-term memory (Conv-LSTM), we propose a new spatial-temporal model of Conv-LSTM two-dimensional bidirectional GCN (CTBGCN) to uncover the potential correlation between origin and destination. We utilize the temporal network for effective temporal information and the spatial network of multi-layers to get the implicit origin-destination correlation. Numerical results suggest that the proposed method outperforms the state-of-the-art baseline and other traditional methods.
更多查看译文
关键词
taxi demand forecasting, origin-destination, bidirectional convolution
AI 理解论文
溯源树
样例
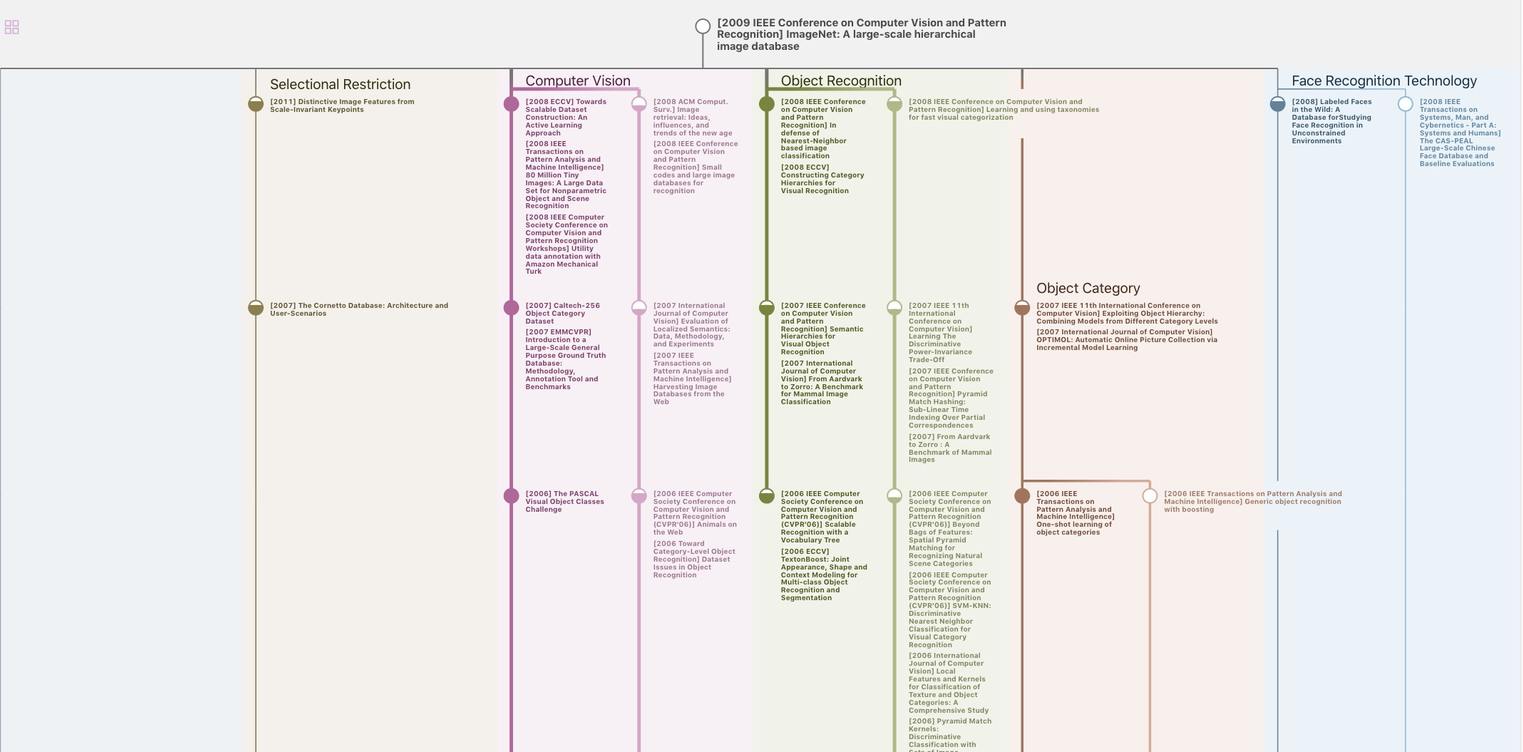
生成溯源树,研究论文发展脉络
Chat Paper
正在生成论文摘要