SUNFIT: A Machine Learning-Based Sustainable University Field Training Framework for Higher Education
SUSTAINABILITY(2023)
摘要
With the rapid advances in Information Technology (IT), the focus on engaging computing students to gain practical experience in the IT industry before graduation is becoming increasingly complex without incorporating pedagogical strategies of success in curricula. The goal is to enable computing major students to gain in-depth knowledge and practical understanding of the IT working environment before graduating through essential industry-driven practical skills based on international standards and best practices. Unfortunately, tracking and analyzing students' practical skills performance during their IT field training programs, which are conducted primarily off-campus at various public and private organizations, before, during, and after the training period, is a daunting task for both the college instructors and the industry trainers. To overcome these challenges, this paper introduces a Sustainable University Field Training (SUNFIT) framework, which is a pedagogical approach towards mining the educational data using machine learning to integrate and measure the field training programs against the internationally recognized accreditation standards such as Accreditation Board for Engineering and Technology (ABET). The study employs machine learning models aimed at continuously measuring and monitoring international ABET accreditation requirements on computing major courses' academic data, elucidating student performance across various semesters, integrating best practices, and producing an evidence-based rationale approach for evaluating weak learning outcomes (LOs) with minimal manual intervention, as well as preventing faculty-specific portfolio errors. The proposed approach could be easily developed by academics, researchers, or even students, and for a variety of purposes, including enhancing poor student outcomes (SOs). In addition, various data mining and machine learning approaches have been investigated over field training assessment data for successful prediction in subsequent cycles. The results are promising, with Naive Bayes obtaining the highest accuracy of 90.54% followed by J48 and PART algorithms at 87.83%.
更多查看译文
关键词
machine learning,computing programs,data management,higher education,curriculum development,practical training
AI 理解论文
溯源树
样例
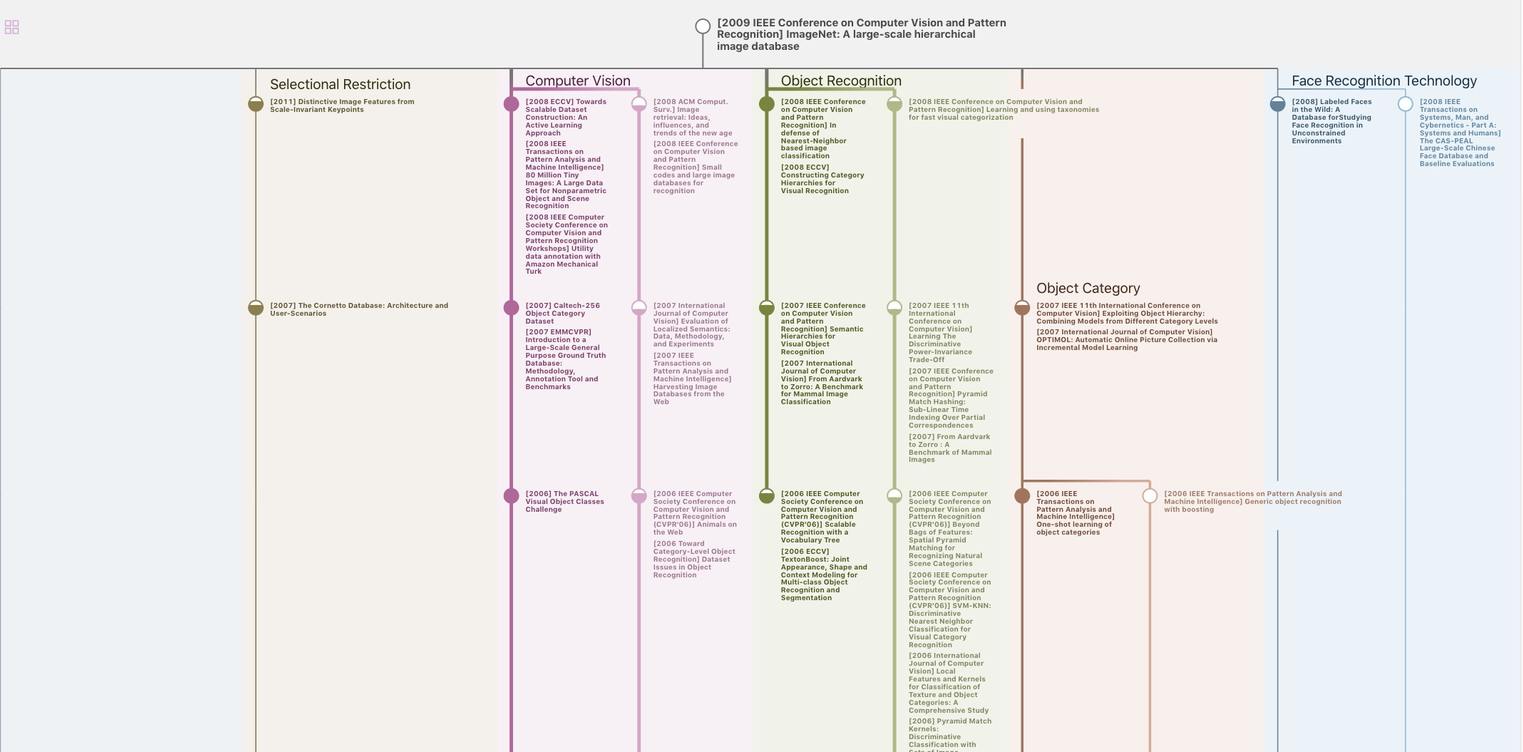
生成溯源树,研究论文发展脉络
Chat Paper
正在生成论文摘要