Assimilation of Blended Satellite Soil Moisture Data Products to Further Improve Noah-MP Model Skills
JOURNAL OF HYDROLOGY(2023)
摘要
Microwave satellite remote sensing has enabled observations of soil moisture (SM) at the global scale, and multiple SM data products have been developed in the past decades. However, single-sensor-based measurements are insufficient for continuous spatiotemporal coverage. In the context of its climate program, the Climate Change Initiative, the European Space Agency (ESA) has developed robust, long term, global scale, multi instrument satellite derived time series of climate data record for key component of the climate system, including soil moisture (CCI), while the Soil Moisture Operational Product System (SMOPS) was specifically developed by National Oceanic and Atmospheric Administration (NOAA) to offer the real time blended SM datasets through merging all available individual products. Before combining, all individual SM data ingested into both SMOPS and CCI blended products are scaled to Global Land Data Assimilation System (GLDAS) 0-10 cm SM climatology. Benefiting from land surface model evolution and the availability of high-quality forcing data, GLDAS has become more comprehensive to track SM changes and dynamic trends. The development of GLDAS and the scaling procedure in CCI and SMOPS leave an open scientific and operational question: do the blended satellite SM data products have added value comparing to the GLDAS product? This study clearly reveals that both CCI and SMOPS can provide the reliable SM observations with independent information, although their climatology matches well with GLDAS. Relative to assimilation of GLDAS 0-10 cm SM data, Noah-MP model can be further improved by assimilating the blended satellite SM observations with respect to the quality-controlled in situ measurements. The strong consistency of results presented in this paper proves that the blended satellite SM data products are more useful than the GLDAS product in terms of improving Noah-MP model performance.
更多查看译文
关键词
soil moisture,assimilation
AI 理解论文
溯源树
样例
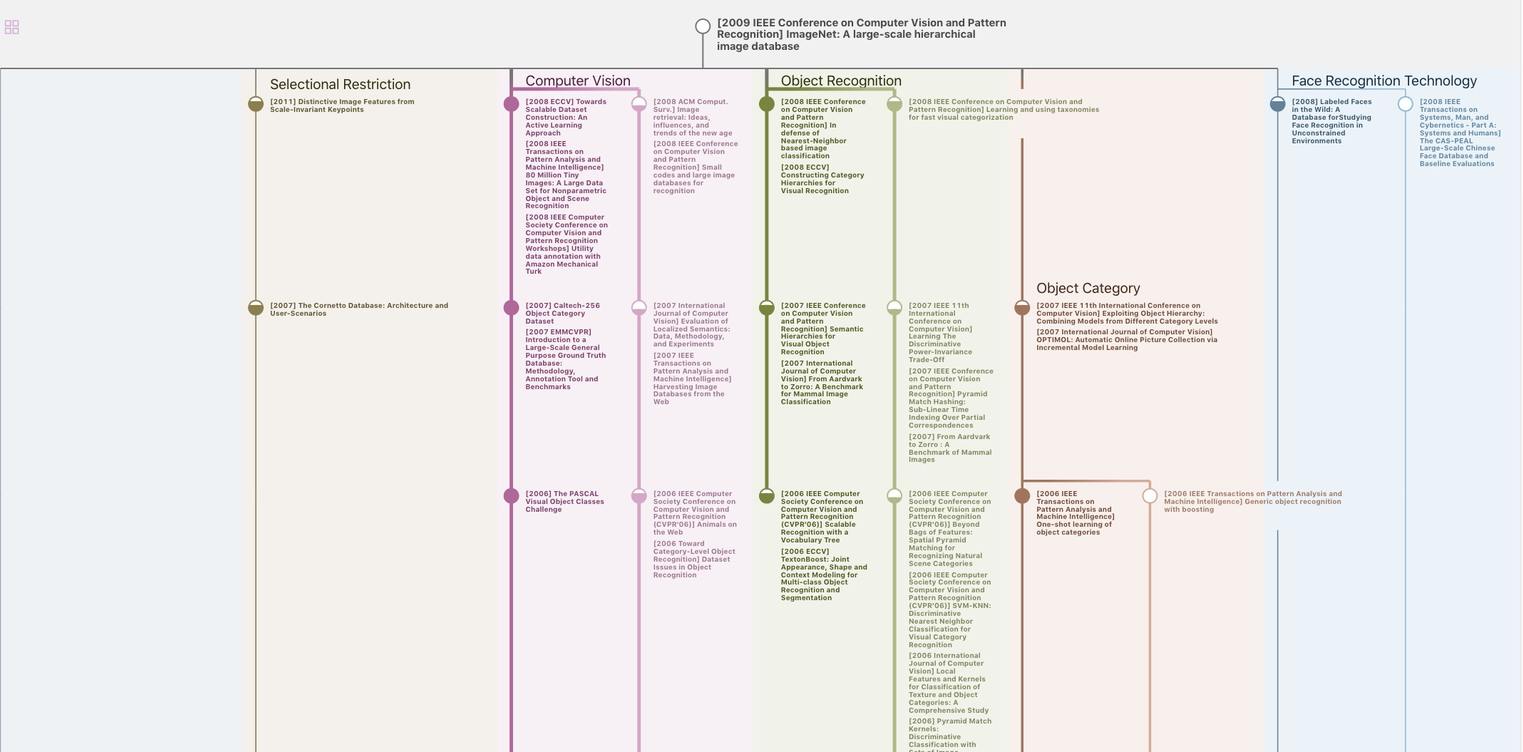
生成溯源树,研究论文发展脉络
Chat Paper
正在生成论文摘要