Changes of terrestrial water storage during 1981-2020 over China based on dynamic-machine learning model
JOURNAL OF HYDROLOGY(2023)
摘要
A reliable estimation of long-term change in terrestrial water storage (TWS) is critical for a sustainable man-agement of freshwater resources and infrastructures. Although the Gravity Recovery and Climate Experiment (GRACE) satellites provide unprecedented accurate TWS anomaly (TWSA) observation since 2002, the limited time span and the gaps between the GRACE and GRACE-Follow On datasets hinder their climatological appli-cations. Here we apply LightGBM, a more accurate and efficient machine learning model, to merge the land surface model simulation, human activities, land properties, and establish a dynamic-machine learning model that can reasonably depict the impacts of climate variation and human interventions on TWSA. We apply the hybrid model to reconstruct the historical TWSA during 1981-2020 over China, which shows reliable results and is consistent with other independent datasets including drought index, TWS change based on water balance, and water withdrawal dataset. The reconstructed TWSA shows significant drier (wetter) trend in North (South) China during the past 40 years, while the TWS variability has increased over 60% regions. We find the change of TWSA is mainly attributed to the climate variation in humid regions, but the human interventions dominate the decline of TWSA in arid and semiarid areas, such as the Yellow and Hai river basins (contributing about 70%). Our findings imply the potential of using artificial intelligence to represent human interventions, which is still un-certain in physical models. Our physical-machine learning model can be applied in long-term prediction and projection of TWSA and its components as well.
更多查看译文
关键词
Terrestrial water storage,Human interventions,Climate change,Machine learning,Dynamic model
AI 理解论文
溯源树
样例
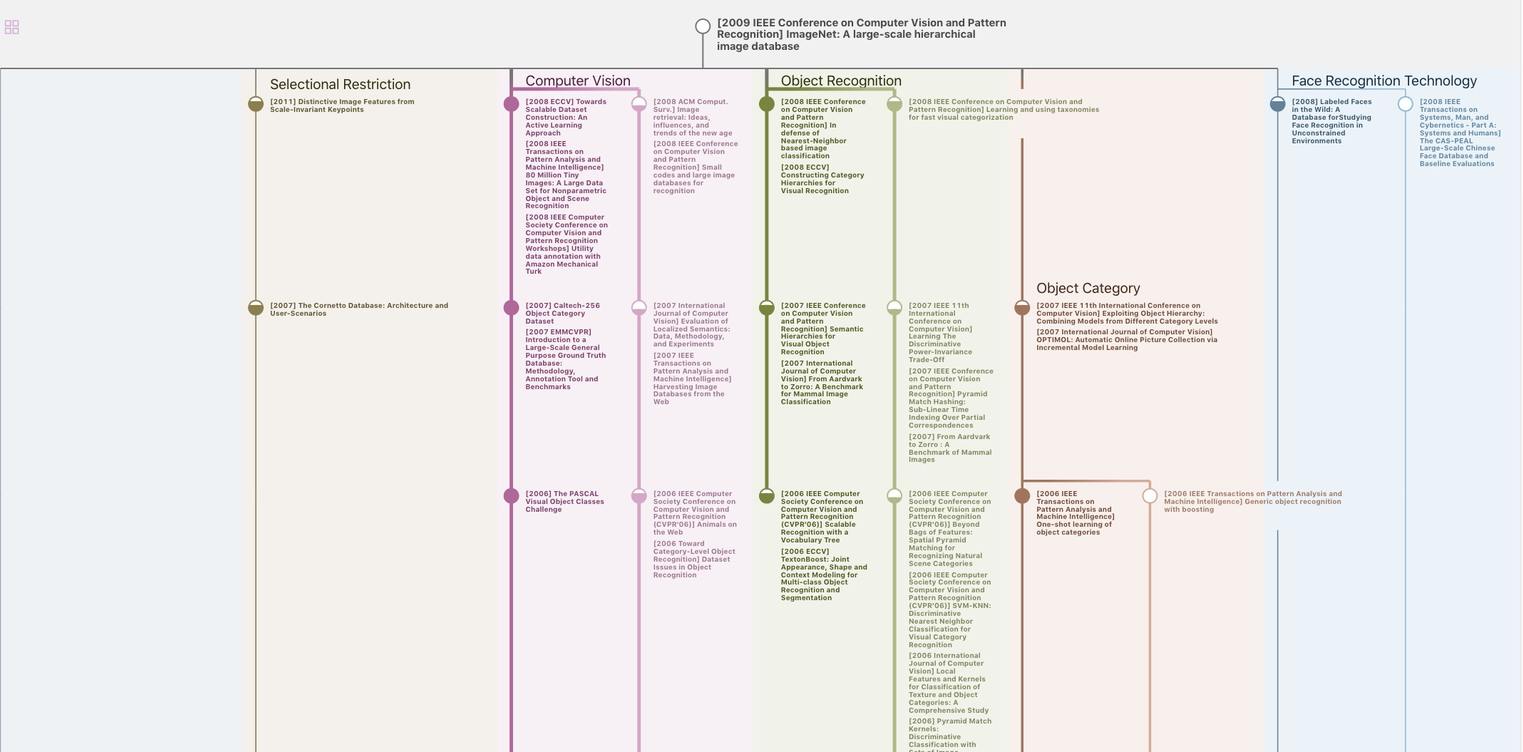
生成溯源树,研究论文发展脉络
Chat Paper
正在生成论文摘要