Data-driven reconstruction of partially observed dynamical systems
NONLINEAR PROCESSES IN GEOPHYSICS(2023)
摘要
The state of the atmosphere, or of the ocean, cannot be exhaustively observed. Crucial parts might remain out of reach of proper monitoring. Also, defining the exact set of equations driving the atmosphere and ocean is virtually impossible because of their complexity. The goal of this paper is to obtain predictions of a partially observed dynamical system without knowing the model equations. In this data-driven context, the article focuses on the Lorenz-63 system, where only the second and third components are observed and access to the equations is not allowed. To account for those strong constraints, a combination of machine learning and data assimilation techniques is proposed. The key aspects are the following: the introduction of latent variables, a linear approximation of the dynamics and a database that is updated iteratively, maximizing the likelihood. We find that the latent variables inferred by the procedure are related to the successive derivatives of the observed components of the dynamical system. The method is also able to reconstruct accurately the local dynamics of the partially observed system. Overall, the proposed methodology is simple, is easy to code and gives promising results, even in the case of small numbers of observations.
更多查看译文
关键词
reconstruction,data-driven
AI 理解论文
溯源树
样例
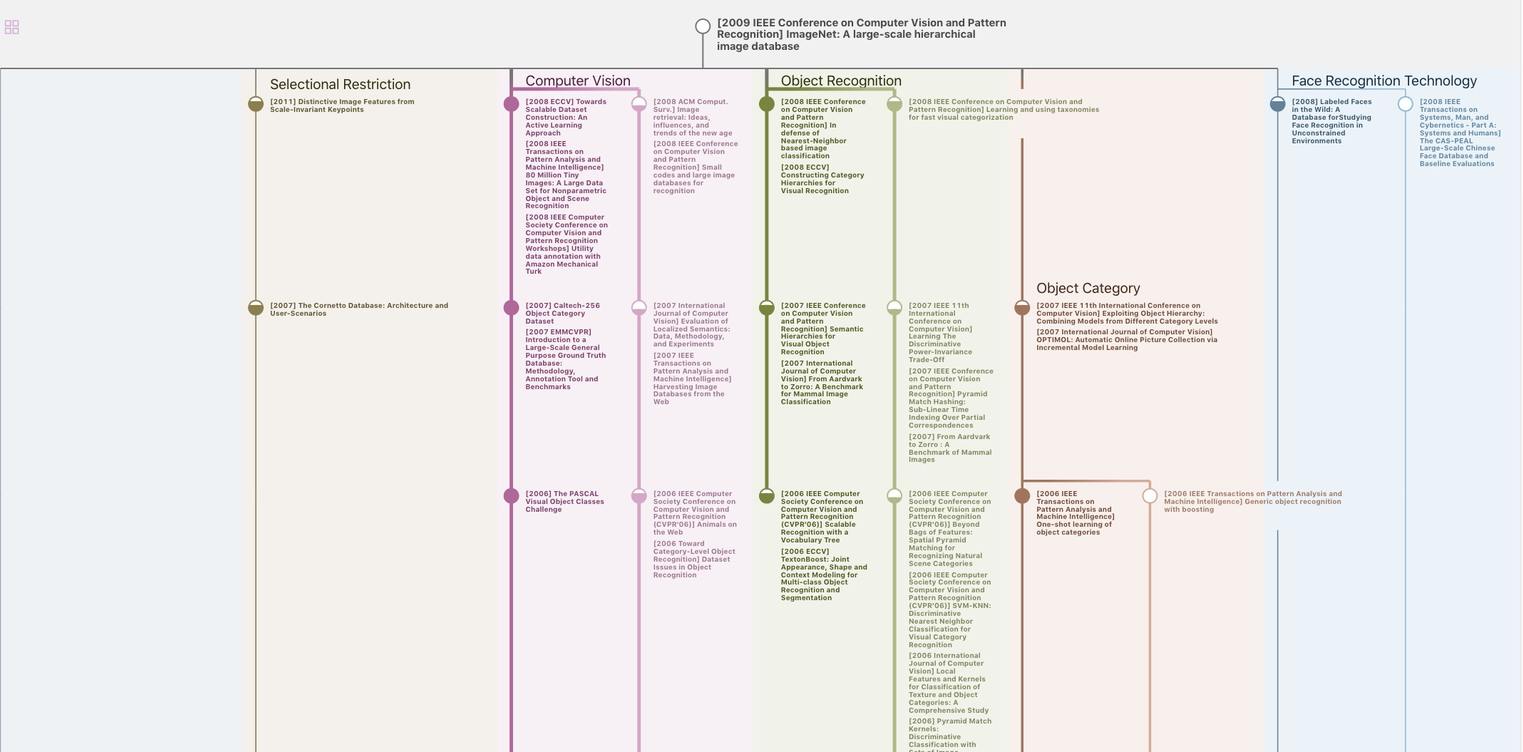
生成溯源树,研究论文发展脉络
Chat Paper
正在生成论文摘要