Augmentation-based discriminative meta-learning for cross-machine few-shot fault diagnosis
Science China Technological Sciences(2023)
摘要
Deep learning methods have demonstrated promising performance in fault diagnosis tasks. Although the scarcity of data in industrial scenarios limits the practical application of such methods, transfer learning effectively tackles this issue through cross-machine knowledge transfer. Nevertheless, the cross-machine few-shot problem, which is a more general industrial scenario, has been rarely investigated. Existing studies have not considered the cross-machine domain shift problem, which results in poor testing performance. This paper proposes an augmentation-based discriminative meta-learning method to address this issue. In the meta-training process, signal transformation is proposed to increase the meta-task diversity for more robust feature learning, and multi-scale learning is combined for more adaptive feature embedding. In the meta-testing process, limited labeled fault information is used to promote model generalization in the target domain through quasi-meta-training based on data augmentation. Furthermore, a novel hyperbolic prototypical loss is proposed for more discriminative feature representation and separable category prototypes by designing a hyperbolic decision boundary. Cross-machine few-shot diagnosis experiments were conducted using three datasets from different machines, namely, the bearing, motor, and gear datasets. The effectiveness of the proposed method was verified through ablation and comparison studies.
更多查看译文
关键词
fault diagnosis,few-shot learning,meta-learning,data augmentation,cross-machine,discriminative loss
AI 理解论文
溯源树
样例
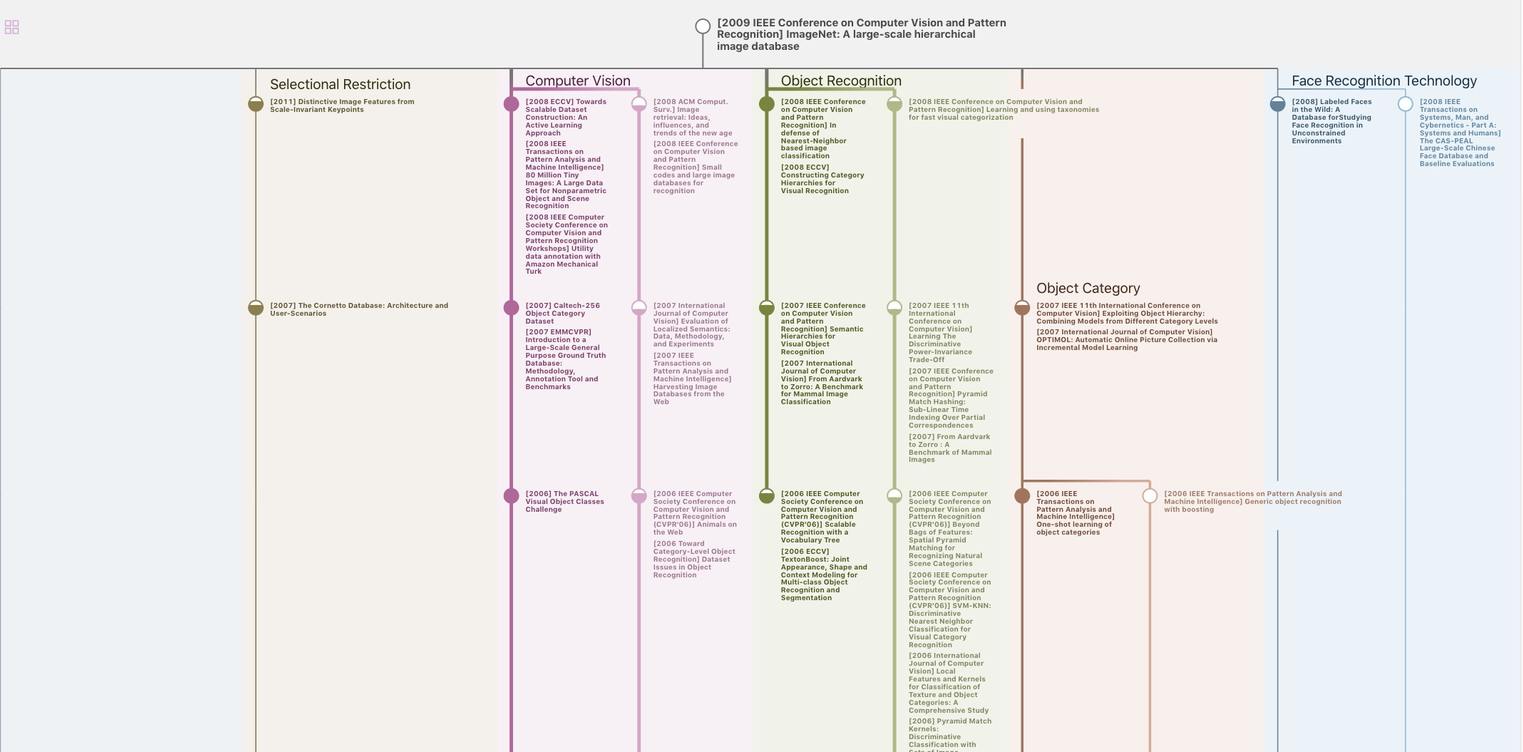
生成溯源树,研究论文发展脉络
Chat Paper
正在生成论文摘要