Exploring fast-inferring in transformer backboned model for fatigue crack detection and propagation tracking for proton exchange membrane
JOURNAL OF POWER SOURCES(2023)
摘要
The research on degradation and failure of proton exchange membrane (PEM) is the basis on cell failure related research. Automatic crack propagation tracking is required to make it possible to provide detailed data of PEM fatigue behavior. However, the special characteristics of PEM require higher performance on local-global attention and noise filtering. To meet this requirement as well as fast inferring, CrackTracker, a Transformerbackboned model, was proposed by introducing a nonlinear projection, developing a new channel-self attention and a new training strategy. Detailed comparisons with four most widely used models on three datasets demonstrated the superiority of CrackTracker in accuracy, efficiency, and generalization performance. The inferring latency on a CPU server of CrackTracker is 16.5 times faster than the slowest one but its Dice coefficient is 3.2% higher than the lowest one. The positional-embedding free design allows CrackTracker flexible to images size. The practical applications on the published X-ray CT images of PEM cracks show that CrackTR can accurately detect the number, area, and segment of cracks in fuel cells, which is very helpful in process safety management. CrackTracker provides a new perspective in network design, especially for industrial applications where sample size and computational cost are tightly limited.
更多查看译文
关键词
Fatigue crack identification,Semantic segmentation,U-shaped structure,Polymer proton exchange membrane,Attention mechanism
AI 理解论文
溯源树
样例
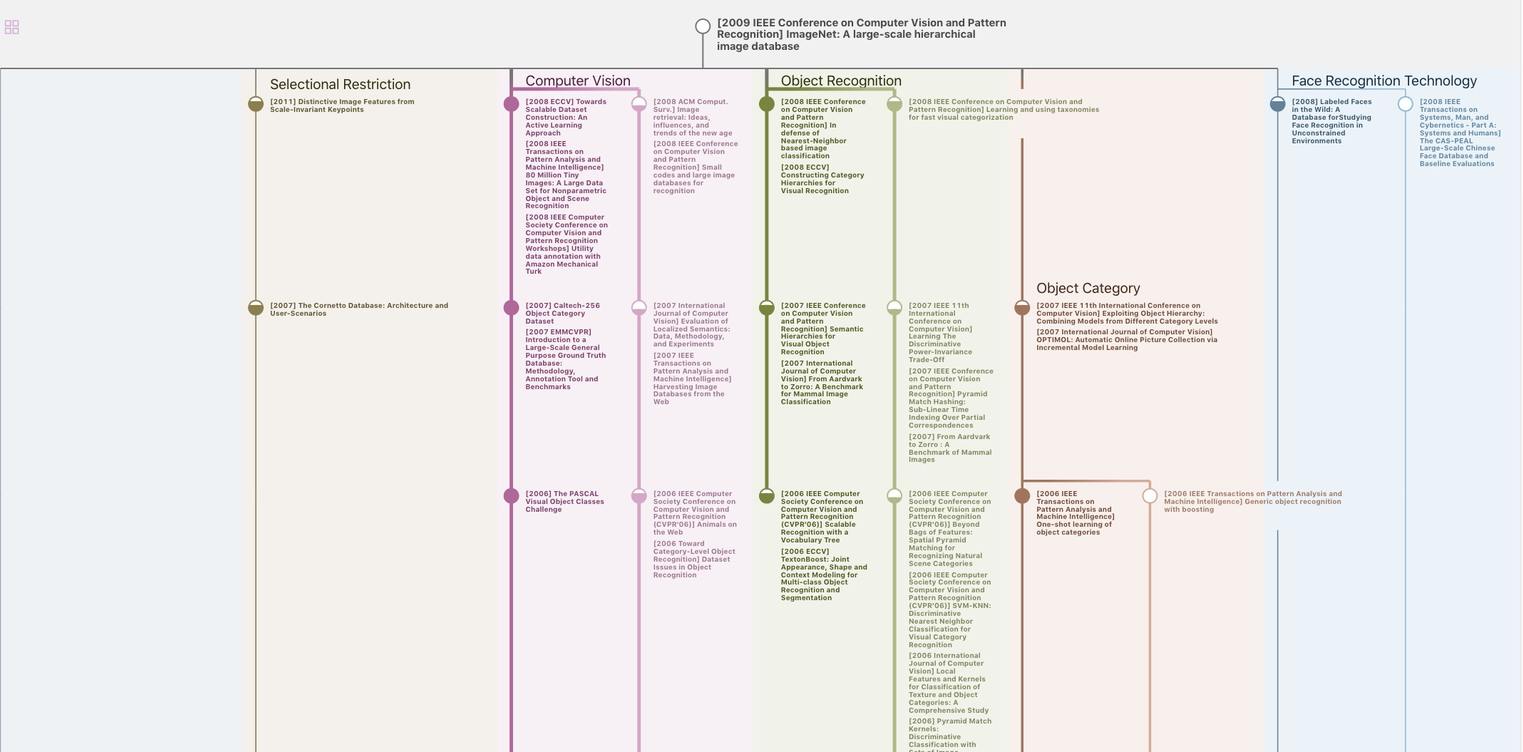
生成溯源树,研究论文发展脉络
Chat Paper
正在生成论文摘要