Variational bayesian analysis of nonhomogeneous hidden markov models with long and ultralong sequences
ANNALS OF APPLIED STATISTICS(2023)
摘要
Nonhomogeneous hidden Markov models (NHMMs) are useful in mod-eling sequential and autocorrelated data. Bayesian approaches, particularly Markov chain Monte Carlo (MCMC) methods, are principal statistical in-ference tools for NHMMs. However, MCMC sampling is computationally demanding, especially for long observation sequences. We develop a vari-ational Bayes (VB) method for NHMMs, which utilizes a structured varia-tional family of Gaussian distributions with factorized covariance matrices to approximate target posteriors, combining a forward-backward algorithm and stochastic gradient ascent in estimation. To improve efficiency and handle ul-tralong sequences, we further propose a subsequence VB (SVB) method that works on subsamples. The SVB method exploits the memory decay property of NHMMs and uses buffers to control for bias caused by breaking sequen-tial dependence from subsampling. We highlight that the local nonhomogene-ity of NHMMs substantially affects the required buffer lengths and propose the use of local Lyapunov exponents that characterize local memory decay rates of NHMMs and adaptively determine buffer lengths. Our methods are validated in simulation studies and in modeling ultralong sequences of cus-tomers' telecom records to uncover the relationship between their mobile In-ternet usage behaviors and conventional telecommunication behaviors.
更多查看译文
关键词
nonhomogeneous hidden markov models,variational bayesian analysis,sequences
AI 理解论文
溯源树
样例
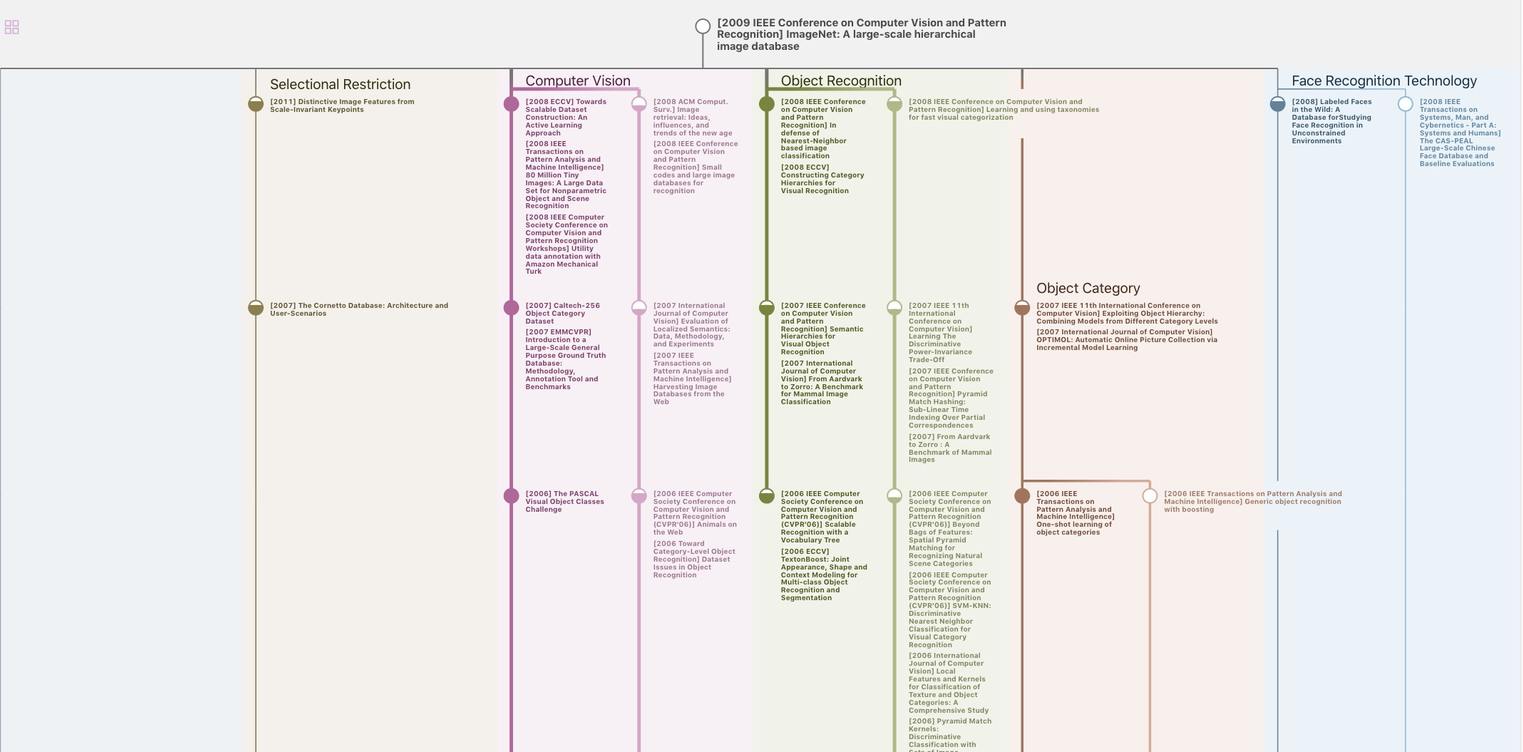
生成溯源树,研究论文发展脉络
Chat Paper
正在生成论文摘要