A Multi-Agent Reinforcement Learning Approach to the Dynamic Job Shop Scheduling Problem
Sustainability(2023)
摘要
In a production environment, scheduling decides job and machine allocations and the operation sequence. In a job shop production system, the wide variety of jobs, complex routes, and real-life events becomes challenging for scheduling activities. New, unexpected events disrupt the production schedule and require dynamic scheduling updates to the production schedule on an event-based basis. To solve the dynamic scheduling problem, we propose a multi-agent system with reinforcement learning aimed at the minimization of tardiness and flow time to improve the dynamic scheduling techniques. The performance of the proposed multi-agent system is compared with the first-in–first-out, shortest processing time, and earliest due date dispatching rules in terms of the minimization of tardy jobs, mean tardiness, maximum tardiness, mean earliness, maximum earliness, mean flow time, maximum flow time, work in process, and makespan. Five scenarios are generated with different arrival intervals of the jobs to the job shop production system. The results of the experiments, performed for the 3 × 3, 5 × 5, and 10 × 10 problem sizes, show that our multi-agent system overperforms compared to the dispatching rules as the workload of the job shop increases. Under a heavy workload, the proposed multi-agent system gives the best results for five performance criteria, which are the proportion of tardy jobs, mean tardiness, maximum tardiness, mean flow time, and maximum flow time.
更多查看译文
关键词
dynamic job shop scheduling problem,multi-agent system,reinforcement learning,Industry 4.0,dispatching rules
AI 理解论文
溯源树
样例
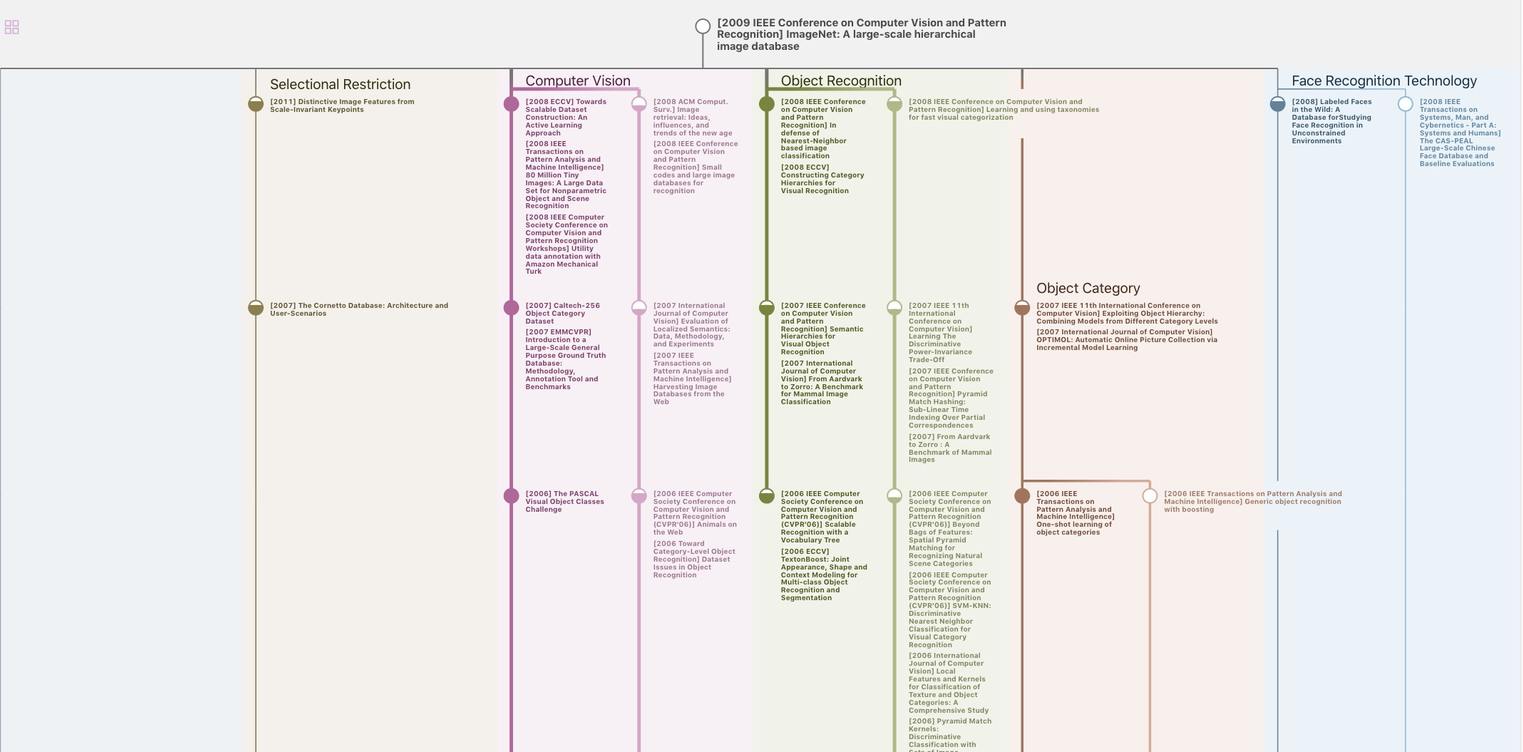
生成溯源树,研究论文发展脉络
Chat Paper
正在生成论文摘要