Attention Based Multi-Agent Reinforcement Learning for Demand Response in Grid-Responsive Buildings
APPLIED ENERGY(2023)
摘要
Integrating renewable energy resources and deploying energy management devices offer great opportunities to develop autonomous energy management systems in grid-responsive buildings. Demand response can promote enhancing demand flexibility and energy efficiency while reducing consumer costs. In this work, we propose a novel multi-agent deep reinforcement learning (MADRL) based approach with an agent assigned to individual buildings to facilitate demand response programs with diverse loads, including space heating/cooling and electrical equipment. Achieving real-time autonomous demand response in networks of buildings is challenging due to uncertain system parameters, the dynamic market price, and complex coupled operational constraints. To develop a scalable approach for automated demand response in networks of interconnected buildings, coordination between buildings is necessary to ensure demand flexibility and the grid's stability. We propose a MADRL technique that utilizes an actor-critic algorithm incorporating shared attention mechanism to enable effective and scalable real-time coordinated demand response in grid-responsive buildings. The presented case studies demonstrate the ability of the proposed approach to obtain decentralized cooperative policies for electricity costs minimization and efficient load shaping without knowledge of building energy systems. The viability of the proposed control approach is also demonstrated by a reduction of over 6% net load demand compared to standard reinforcement learning approaches, deep deterministic policy gradient, and soft actor-critic algorithm, as well as a tailored MADRL approach for demand response.
更多查看译文
关键词
Demand response,Deep reinforcement learning,Multi -agent,Buildings
AI 理解论文
溯源树
样例
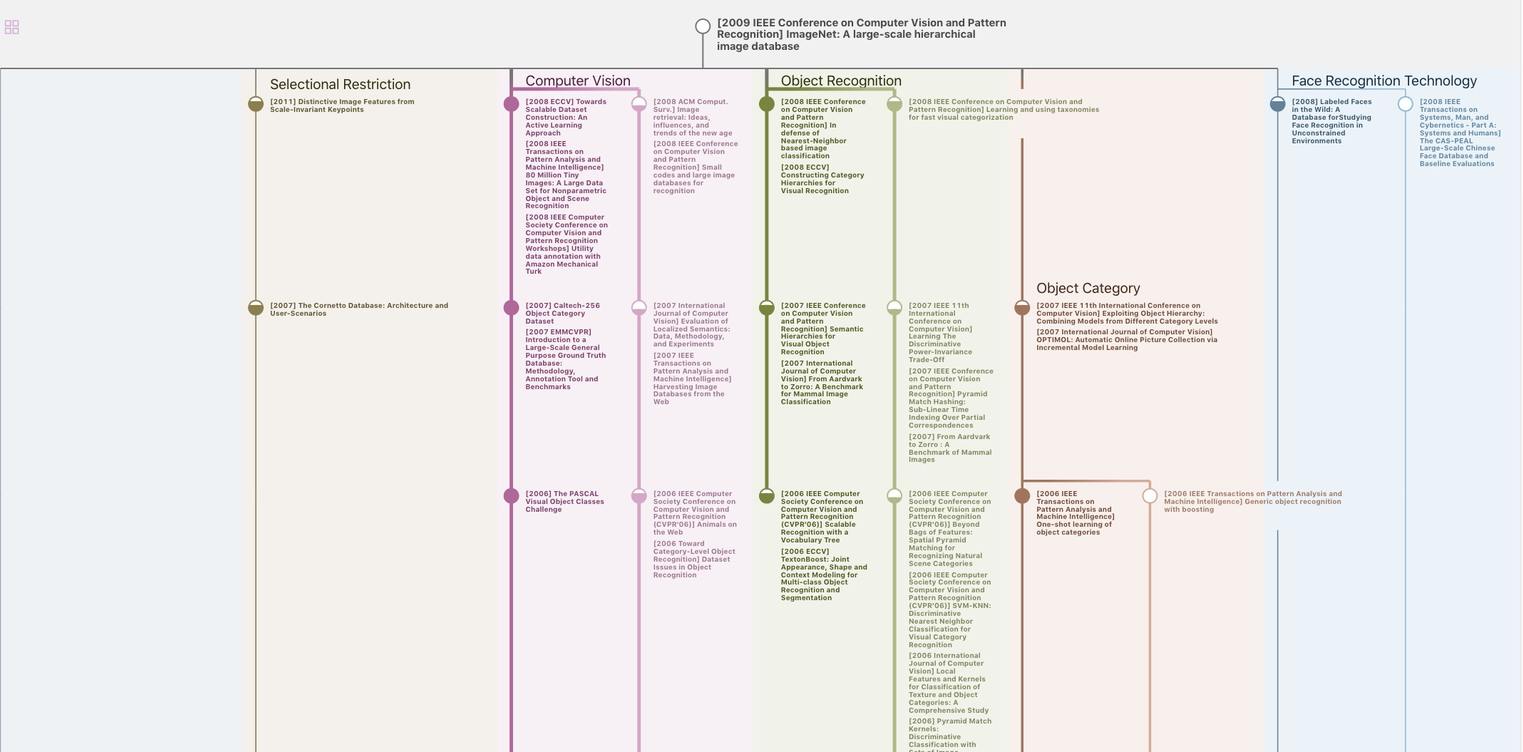
生成溯源树,研究论文发展脉络
Chat Paper
正在生成论文摘要