Regularized K-means Through Hard-Thresholding
JOURNAL OF MACHINE LEARNING RESEARCH(2022)
摘要
We study a framework for performing regularized K-means, based on direct penalization of the size of the cluster centers. Different penalization strategies are considered and compared in a theoretical analysis and an extensive Monte Carlo simulation study. Based on the results, we propose a new method called hard-threshold K-means (HTK-means), which uses an t0 penalty to induce sparsity. HTK-means is a fast and competitive sparse clustering method which is easily interpretable, as is illustrated on several real data examples. In this context, new graphical displays are presented and used to gain further insight into the data sets.
更多查看译文
关键词
clustering, penalized, variable selection, t0
AI 理解论文
溯源树
样例
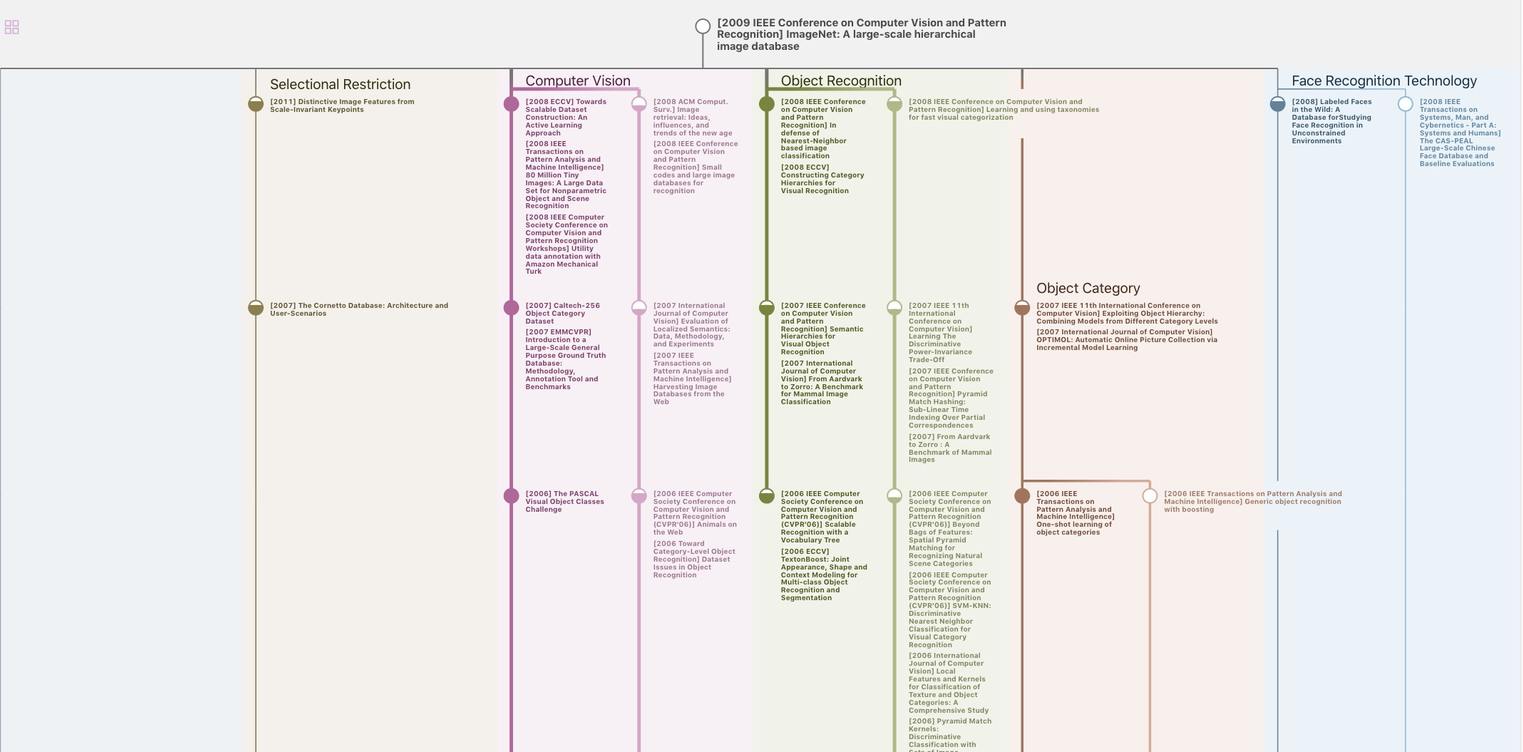
生成溯源树,研究论文发展脉络
Chat Paper
正在生成论文摘要