Application of improved physics-informed neural networks for nonlinear consolidation problems with continuous drainage boundary conditions
ACTA GEOTECHNICA(2024)
摘要
In this paper, improved physics-informed neural networks (PINNs) with hard constraints (PINNs-H) are introduced to simulate the variation of the excess pore water pressure in the nonlinear consolidation problems with continuous drainage boundary conditions. In the PINNs-H, we modify the network architecture to automatically satisfy the corresponding initial and boundary conditions accurately, and obtain high-precision soil consolidation behaviors. The accuracy and effectiveness of the presented PINNs-H are demonstrated on two examples of the nonlinear consolidation models. Specifically, the results indicate that based on less training data, we may better predict the consolidation behaviors through the PINNs-H. Furthermore, the training data required by the PINNs-H is significantly less than the grid point data of the finite difference method (FDM), and the PINNs-H exhibits a better memory advantage. For the inverse problem, we find that on the basis of less observed data of the excess pore water pressure, the PINNs can provide a great estimate to the interface parameters of the continuous drainage boundary conditions, and effectively resist the noise interference. We also use the PINNs and PINNs-H to identify the nonlinear factor, and reveal that PINNs-H can provide high-precision predicted results, whereas the PINNs fail.
更多查看译文
关键词
Continuous drainage boundary conditions,Hard constraints,Numerical solutions,Parameter inversion,Physics-informed neural network
AI 理解论文
溯源树
样例
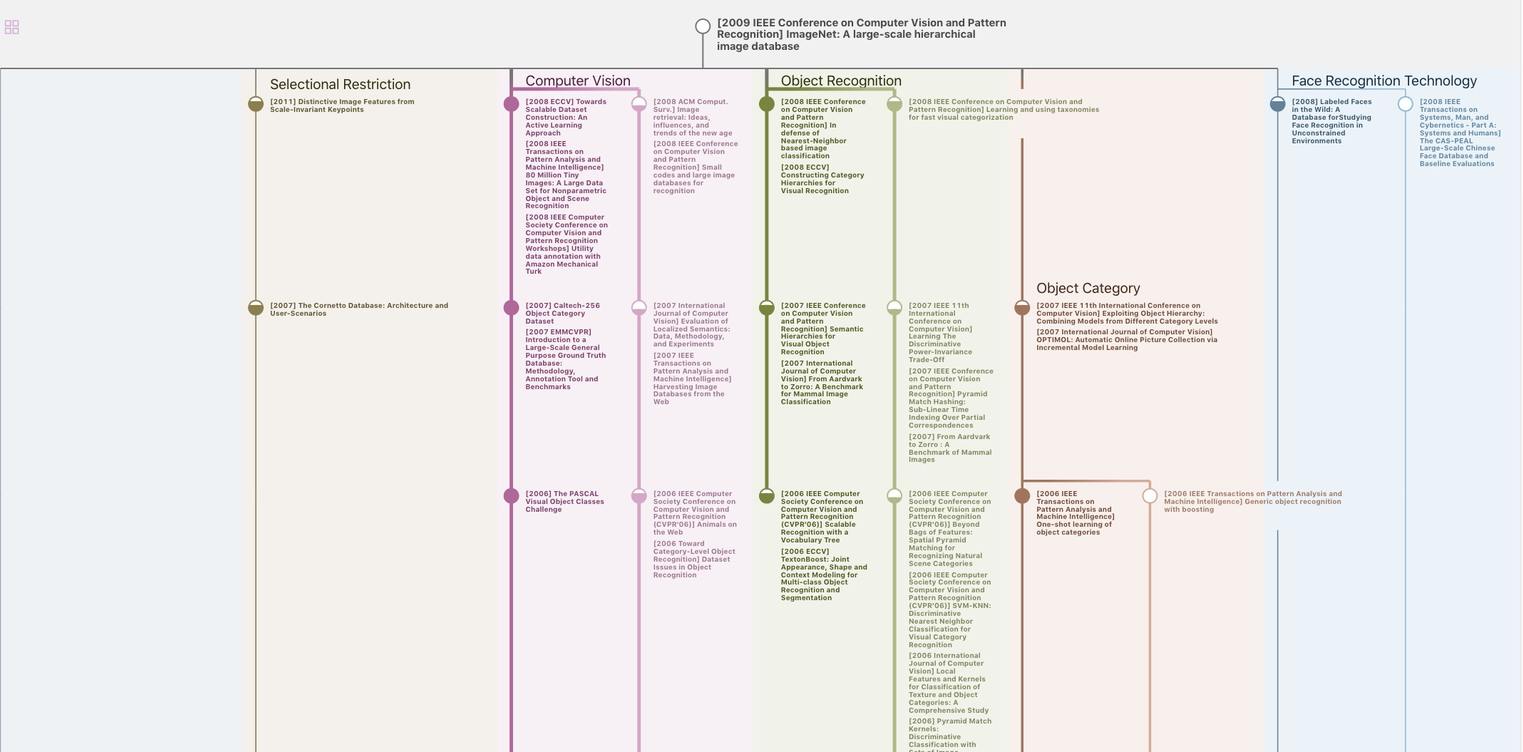
生成溯源树,研究论文发展脉络
Chat Paper
正在生成论文摘要