A Machine Learning-Based Approach to Time-series Wave Identification in the Solar Wind
ASTROPHYSICAL JOURNAL(2023)
摘要
The Wind spacecraft has yielded several decades of high-resolution magnetic field data, a large fraction of which displays small-scale structures. In particular, the solar wind is full of wavelike fluctuations that appear in both the field magnitude and its components. The nature of these fluctuations can be tied to the properties of other structures in the solar wind, such as shocks, that have implications for the time evolution of the solar wind. As such, having a large collection of wave events would facilitate further study of the effects that these fluctuations have on solar wind evolution. Given the large volume of magnetic field data available, machine learning is the most practical approach to classifying the myriad small-scale structures observed. To this end, a subset of Wind data is labeled and used as a training set for a multibranch 1D convolutional neural network aimed at classifying circularly polarized wave modes. Using this algorithm, a preliminary statistical study of 1 yr of data is performed, yielding about 300,000 wave intervals out of about 5,000,000 solar wind intervals. The wave intervals come about more often in the fast solar wind and at higher temperatures, and the number of waves per day is highly periodic. This machine learning-based approach to wave detection has the potential to be a powerful, inexpensive way to catalog waves throughout decades of spacecraft data.
更多查看译文
关键词
solar wind,machine learning–based,time-series time-series
AI 理解论文
溯源树
样例
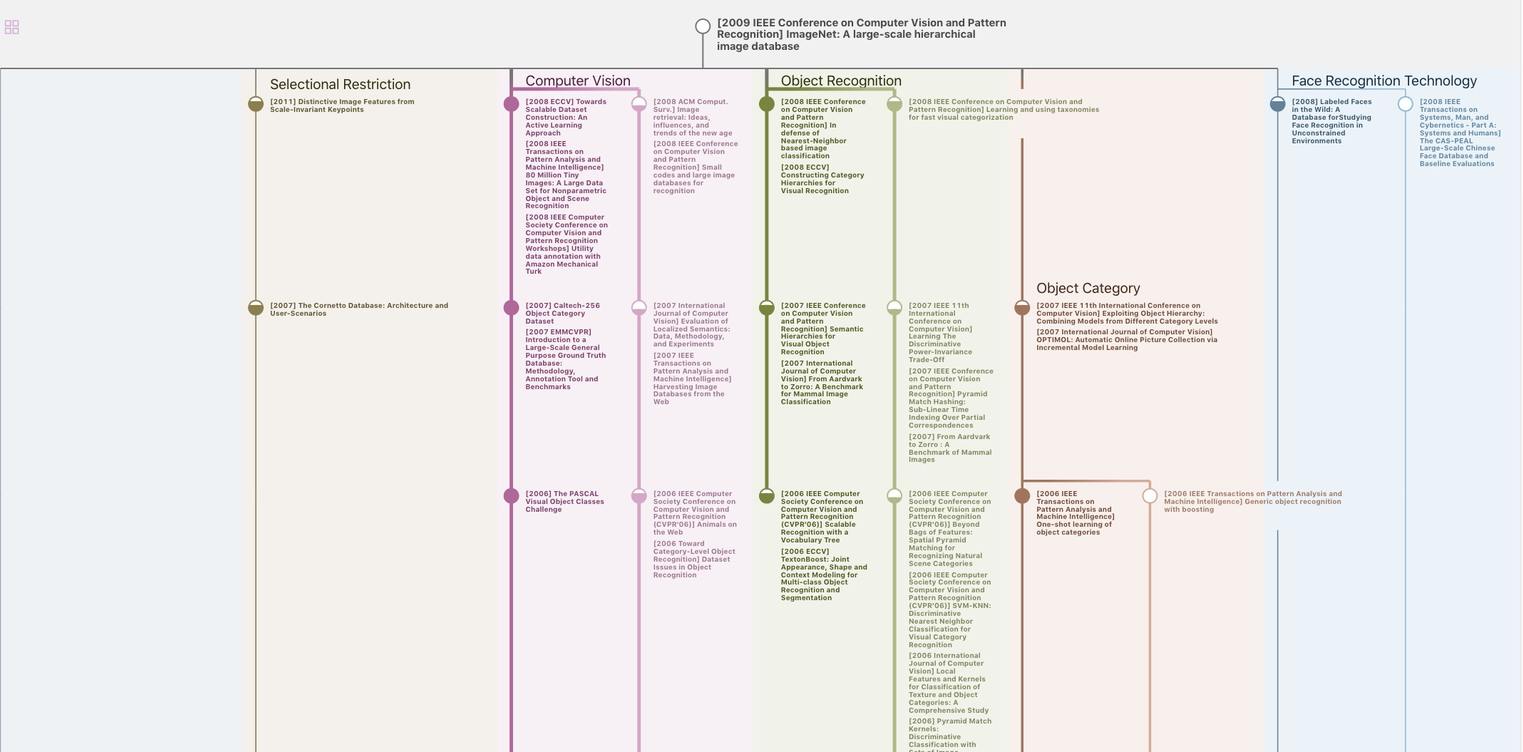
生成溯源树,研究论文发展脉络
Chat Paper
正在生成论文摘要