"I'M Sorry Dave, I'M Afraid I Can'T Do That" Deep Q-Learning From Forbidden Actions
2020 INTERNATIONAL JOINT CONFERENCE ON NEURAL NETWORKS (IJCNN)(2020)
摘要
The use of Reinforcement Learning (RL) is still restricted to simulation or to enhance human-operated systems through recommendations. Real-world environments (e.g. industrial robots or power grids) are generally designed with safety constraints in mind implemented in the shape of valid actions masks or contingency controllers. For example, the range of motion and the angles of the motors of a robot can be limited to physical boundaries. Violating constraints thus results in rejected actions or entering in a safe mode driven by an external controller, making RL agents incapable of learning from their mistakes. In this paper, we propose a simple modification of a state-of-the-art deep RL algorithm (DQN), enabling learning from forbidden actions. To do so, the standard Q-learning update is enhanced with an extra safety loss inspired by structured classification. We empirically show that it reduces the number of hit constraints during the learning phase and accelerates convergence to near-optimal policies compared to using standard DQN. Experiments are done on a Visual Grid World Environment and the TextWorld domain.
更多查看译文
关键词
Deep Reinforcement Learning, Safety, constraints, Q-Learning
AI 理解论文
溯源树
样例
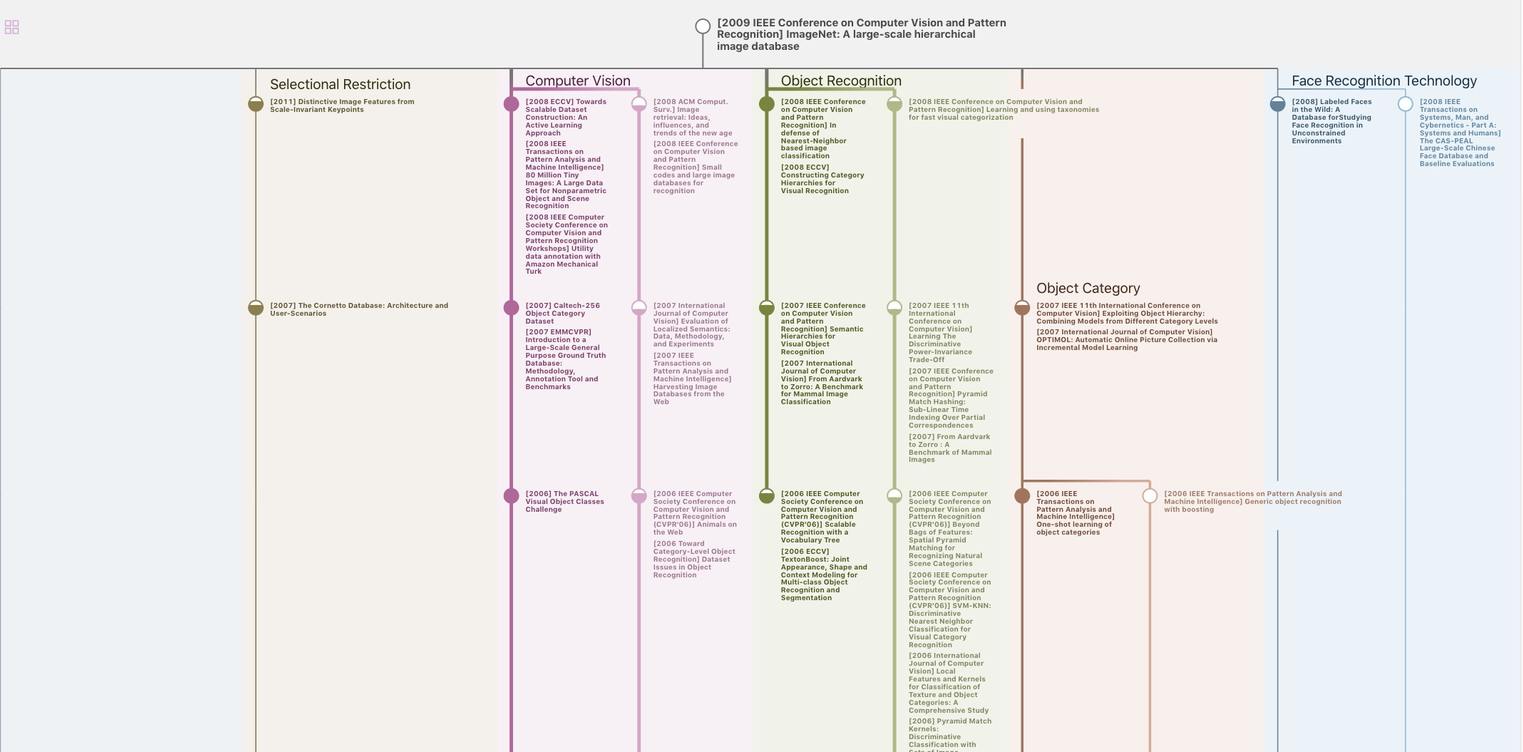
生成溯源树,研究论文发展脉络
Chat Paper
正在生成论文摘要