A New Hybrid Model for Segmentation of the Skin Lesion Based on Residual Attention U-Net
CMC-COMPUTERS MATERIALS & CONTINUA(2023)
摘要
Skin segmentation participates significantly in various biomedical applications, such as skin cancer identification and skin lesion detection. This paper presents a novel framework for segmenting the skin. The framework contains two main stages: The first stage is for removing different types of noises from the dermoscopic images, such as hair, speckle, and impulse noise, and the second stage is for segmentation of the dermoscopic images using an attention residual U-shaped Network (U-Net). The framework uses variational Autoencoders (VAEs) for removing the hair noises, the Generative Adversarial Denoising Network (DGAN-Net), the Denoising U-shaped U-Net (D-U-NET), and Batch Renormalization U-Net (Br-U-NET) for removing the speckle noise, and the Laplacian Vector Median Filter (MLVMF) for removing the impulse noise. In the second main stage, the residual attention u-net was used for segmentation. The framework achieves (35.11, 31.26, 27.01, and 26.16), (36.34, 33.23, 31.32, and 28.65), and (36.33, 32.21, 28.54, and 27.11) for removing hair, speckle, and impulse noise, respectively, based on Peak Signal Noise Ratio (PSNR) at the level of (0.1, 0.25, 0.5, and 0.75) of noise. The framework also achieves an accuracy of nearly 94.26 in the dice score in the process of segmentation before removing noise and 95.22 after removing different types of noise. The experiments have shown the efficiency of the used model in removing noise according to the structural similarity index measure (SSIM) and PSNR and in the segmentation process as well.
更多查看译文
关键词
Skin tumor,speckle noise,impulse noise,hair noise,deep learning,segmentation
AI 理解论文
溯源树
样例
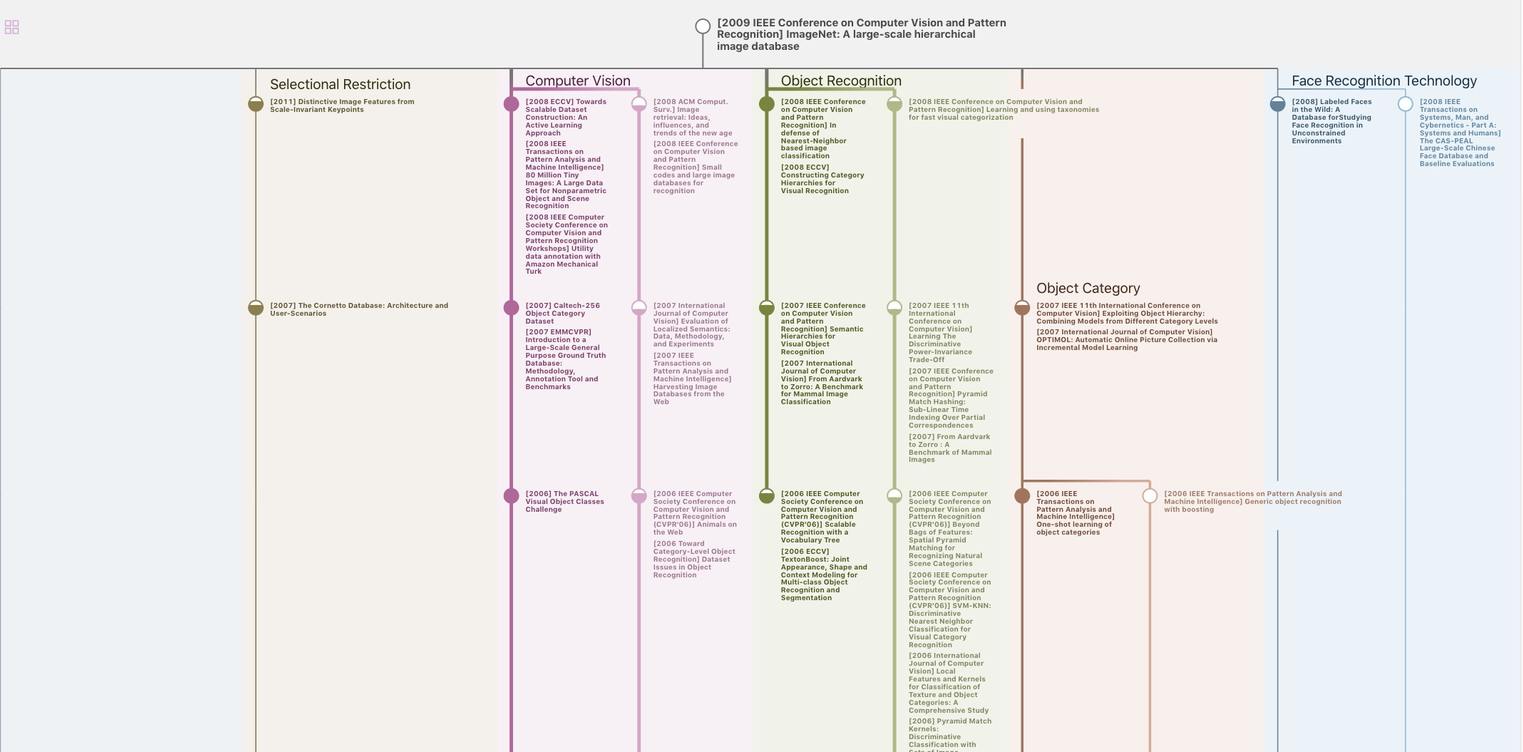
生成溯源树,研究论文发展脉络
Chat Paper
正在生成论文摘要