Physics-Informed Spatiotemporal Learning Framework for Urban Traffic State Estimation
Journal of transportation engineering Part A Systems/Journal of transportation engineering Part A Systems(2023)
摘要
Accurate traffic estimation on urban networks is a prerequisite for efficient traffic detection, congestion warning, and transportation schedule. The current estimation methods can be roughly divided into model-driven and the data-driven methods. The estimation accuracy of the model-driven methods cannot satisfy certain applications. Meanwhile, the data-driven methods have the disadvantages of poor generalization ability and weak interpretability. To overcome these challenges, this paper proposes a framework named the physics-informed spatiotemporal graph convolution neural network (PSTGCN) based on physics-informed deep learning theories. The PSTGCN uses a spatiotemporal graph convolution neural network combined with traffic flow models to estimate the traffic state. The proposed model not only considers the temporal and spatial dependence of traffic flow but also abides by the internal law of traffic flow. Furthermore, the estimation objects of the proposed model are multiple variables that comprehensively represent the traffic state. Experiments on real-world traffic data reveal the error of the PSTGCN is reduced by 38.39% compared to the baselines. Also, the PSTGCN can achieve a similar prediction effect as the baselines by using half of the global spatial information. These results demonstrate that the PSTGCN outperforms the state-of-the-art models in urban traffic estimation and is robust under variable road conditions and data scales.
更多查看译文
关键词
Urban network,Physics-informed deep learning,Traffic state estimation,Hybrid model
AI 理解论文
溯源树
样例
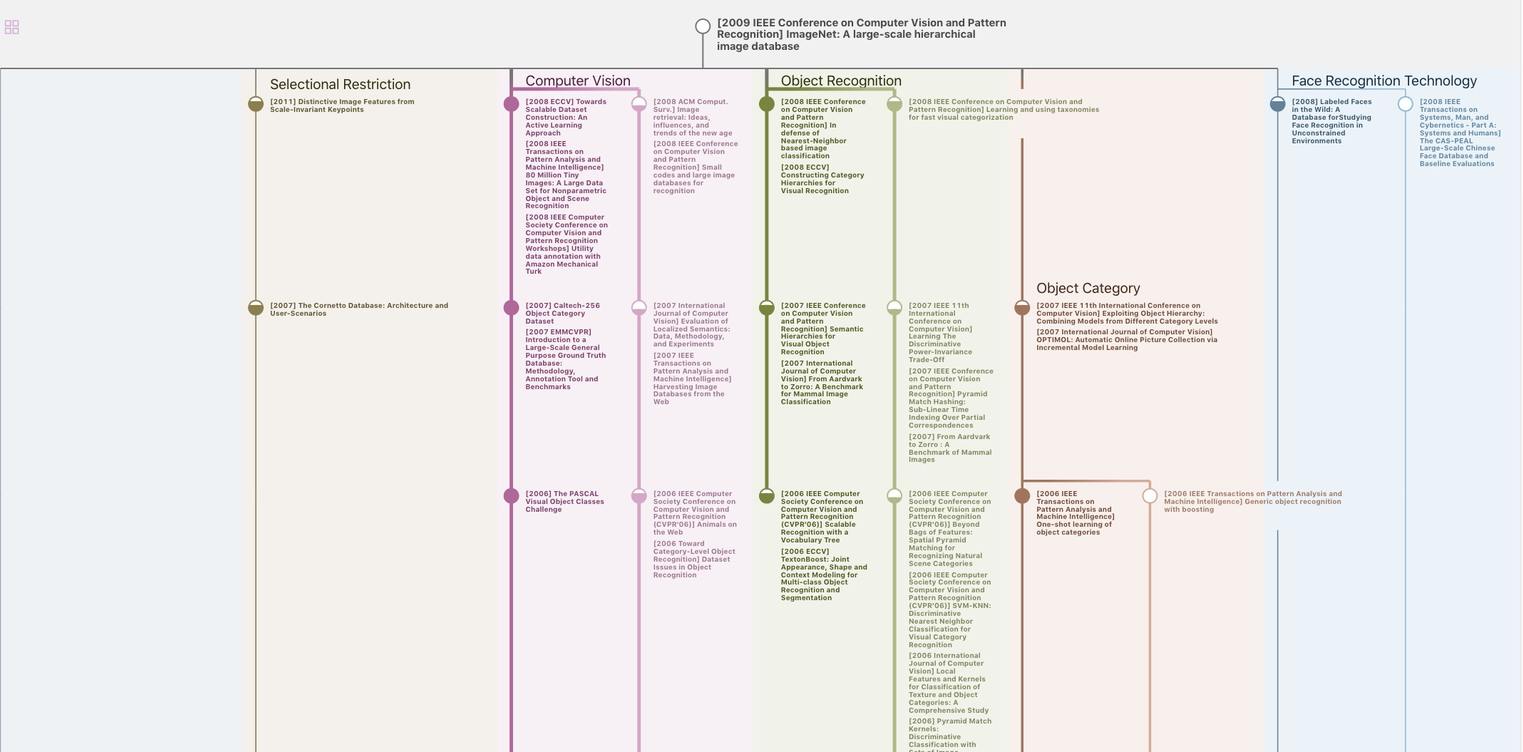
生成溯源树,研究论文发展脉络
Chat Paper
正在生成论文摘要