The Interacting Multiple Model Smooth Variable Structure Filter for Trajectory Prediction
IEEE Transactions on Intelligent Transportation Systems(2023)
摘要
An autonomous vehicle would benefit from being able to predict trajectories of other vehicles in its vicinity for improved safety. In order for the self-driving car to plan safe trajectories, paths of nearby vehicles are required to be predicted for risk assessment, decision making, and motion planning. In this study, a trajectory prediction algorithm based on the Interacting Multiple Model (IMM) estimation strategy is proposed to predict paths involving lane-changing, lane-keeping, and turning motion. More specifically, the Interacting Multiple Model estimation technique is used with models defined in curvi-linear coordinates to predict a vehicle’s trajectory based on prior behavioral maneuvers. The road geometry is used to help facilitate behavior identification and prediction. Moreover, the combination of a more recently developed estimation technique known as the Generalized Variable Boundary Layer-Smooth Variable Structure Filter and the Interacting Multiple Model Estimator is applied to track, identify behaviors, and predict trajectories of a vehicle. The performance of this technique is compared with a Kalman Filter based formulation using synthetic and experimental data. This model-based strategy is also compared with machine learning-based strategies for trajectory prediction.
更多查看译文
关键词
Trajectory,Predictive models,Current measurement,Prediction algorithms,Behavioral sciences,Neural networks,Tracking,Vehicle trajectory prediction,target tracking,interacting multiple model (IMM),smooth variable structure filter (SVSF)
AI 理解论文
溯源树
样例
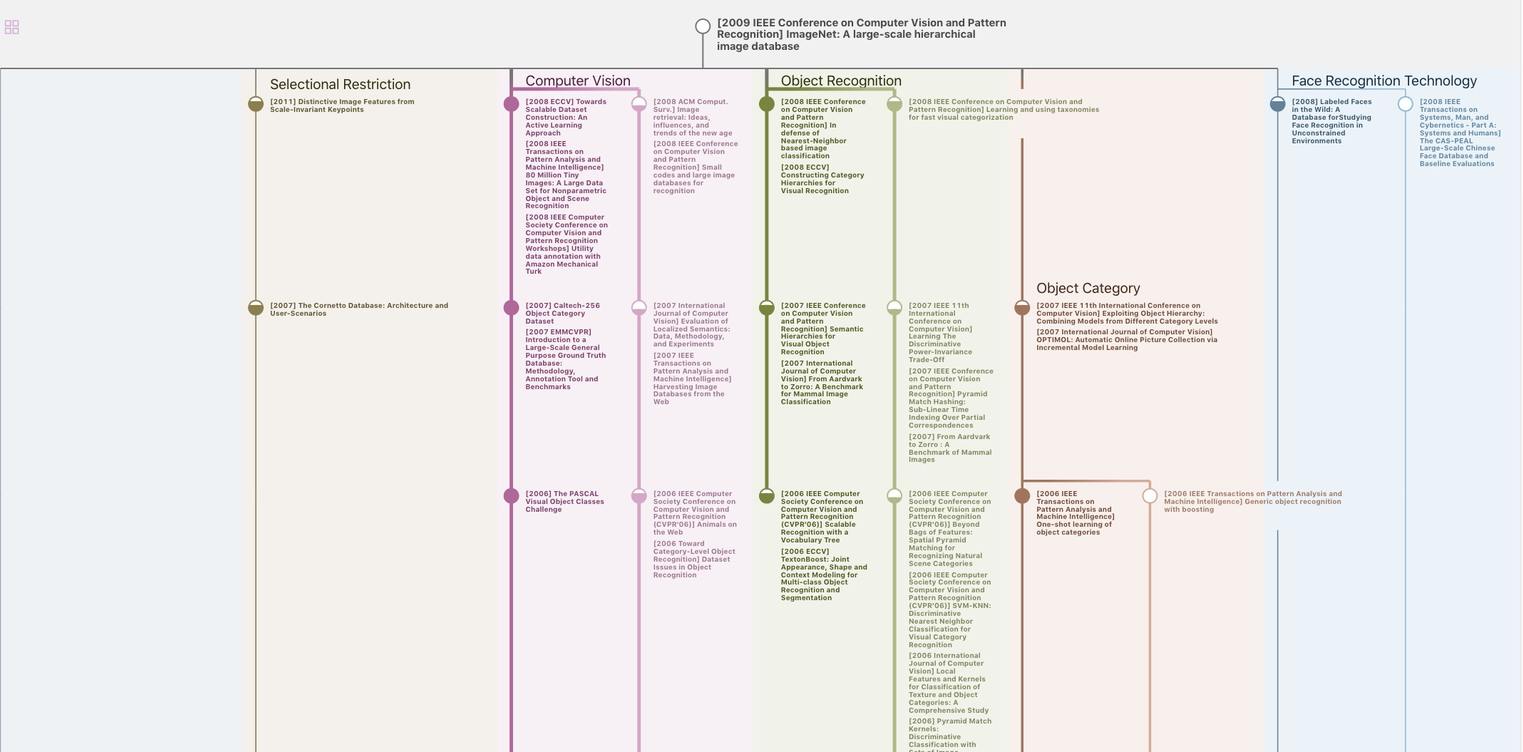
生成溯源树,研究论文发展脉络
Chat Paper
正在生成论文摘要