Machine learning approach for assessment of arsenic levels using physicochemical properties of water, soil, elevation, and land cover
Environmental monitoring and assessment(2023)
摘要
Groundwater is an essential resource; around 2.5 billion people depend on it for drinking and irrigation. Groundwater arsenic contamination is due to natural and anthropogenic sources. The World Health Organization (WHO) has proposed a guideline value for arsenic concentration in groundwater samples of 10 μ g/L. Continuous consumption of arsenic-contaminated water causes various carcinogenic and non-carcinogenic health risks. In this paper, we introduce a geospatial-based machine learning method for classifying arsenic concentration levels as high (1) or low (0) using physicochemical properties of water, soil type, land use land cover, digital elevation, subsoil sand, silt, clay, and organic content of the region. The groundwater samples were collected from multiple sites along the river Ganga’s banks of Varanasi district in Uttar Pradesh, India. The dataset was subjected to descriptive statistics and spatial analysis for all parameters. This study assesses the various contributing parameters responsible for the occurrence of arsenic in the study area based on the Pearson correlation feature selection method. The performance of machine learning models, i.e., Extreme Gradient Boosting (XGBoost), Gradient Boosting Machine (GBM), Decision Tree, Random Forest, Naïve Bayes, and Deep Neural Network (DNN), were compared to validate the parameters responsible for the dissolution of arsenic in groundwater aquifers. Among all the models, the DNN algorithm outclasses other classifiers as it has a high accuracy of 92.30
更多查看译文
关键词
Arsenic,Random forest,DNN,XGBoost,Naive bayes,GBM
AI 理解论文
溯源树
样例
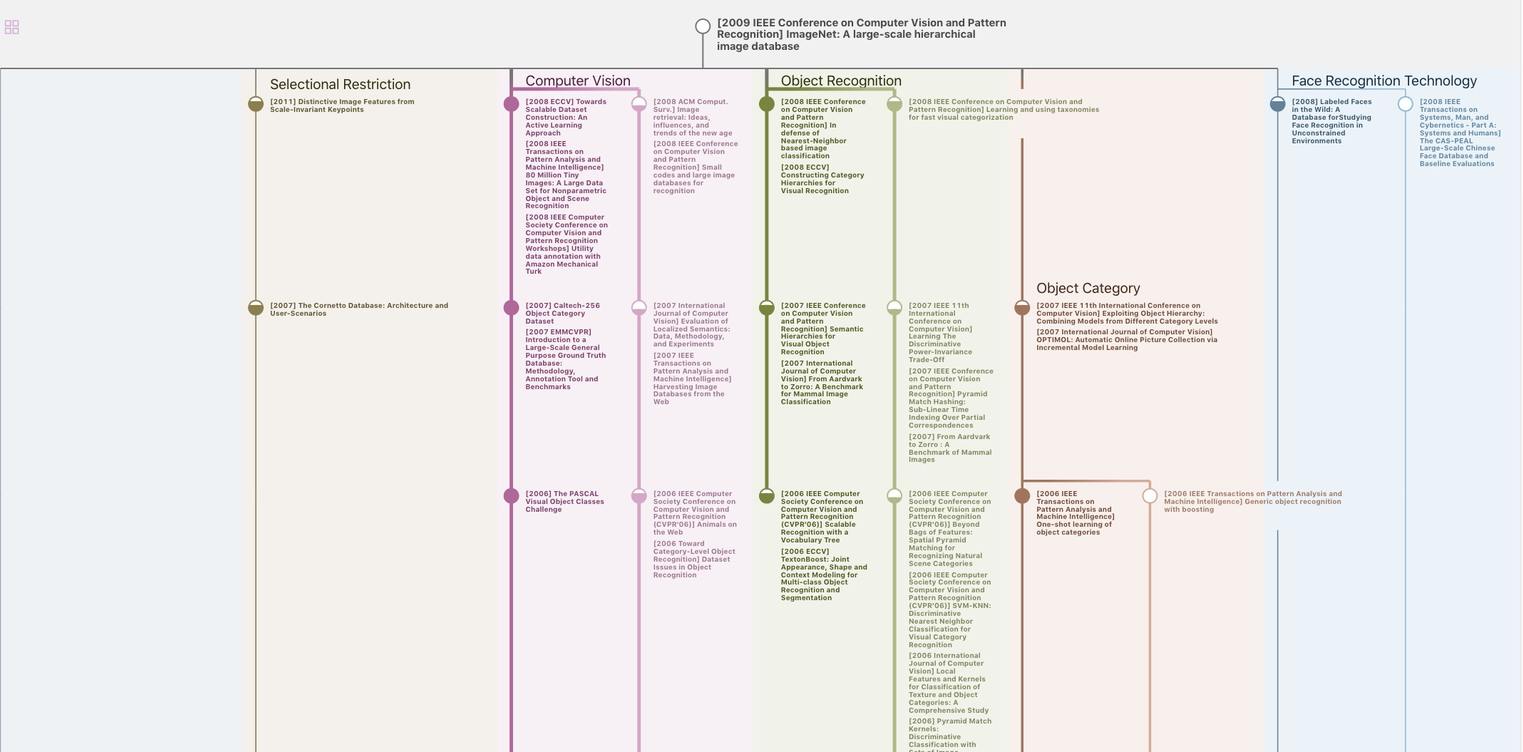
生成溯源树,研究论文发展脉络
Chat Paper
正在生成论文摘要