Dynamic kernel network for hyperspectral image classification
INTERNATIONAL JOURNAL OF REMOTE SENSING(2023)
摘要
Deep neural networks have outstanding performance as data-driven solutions for hyperspectral image classification for ground object detection. Among these, convolutional models outperform other strategies for their back-propagated local-filtering mechanisms. However, convolutional models lack the support of intuitive details learning and squeezed weighted distribution. These solutions have constraints of exploring only within convolution space. To alleviate these issues, in this paper, a multi-domain kernel network has been proposed leveraging a dynamic attention mechanism in order to harvest spectral-spatial domain information from multiple receptive regions. The Dynamic Kernel Network uses various kernel strategies to gather maximum spectral-spatial features for high-performance classification. Experimental results on the real hyperspectral images explicate the validity of the proposed dynamic kernel network. The proposed framework outperforms the base methods and attained 97.225%, 99.385% & 99.922% overall accuracy (OA) on Indian Pines (IP), University of Pavia (UP) & Salinas Valley (SV) datasets, respectively. Also, the ablative analysis on spatial windows, spectral bands, computation time and training samples proves the robustness of the spectral-spatial kernel learning strategy.
更多查看译文
关键词
Hyperspectral imagery,deep learning,dynamic kernel learning
AI 理解论文
溯源树
样例
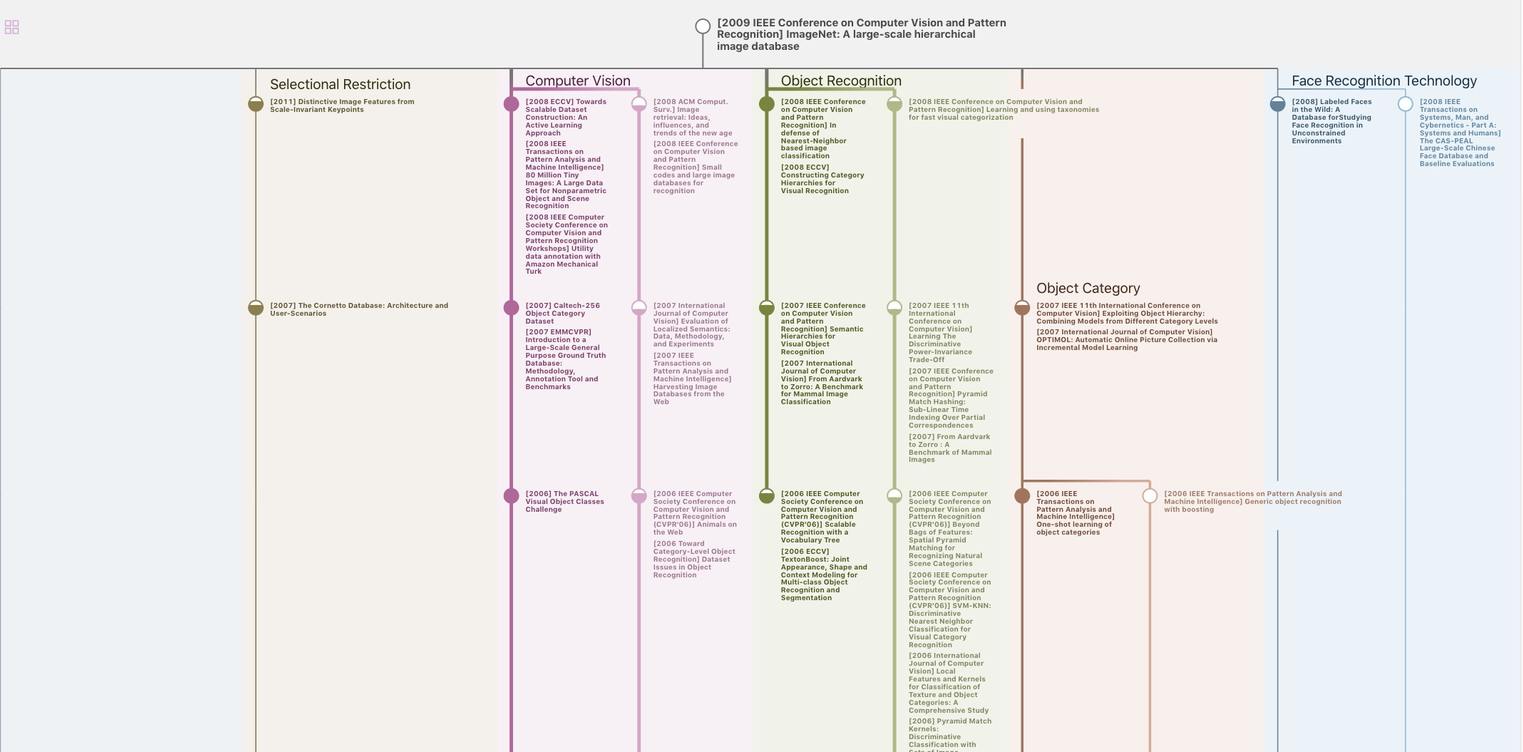
生成溯源树,研究论文发展脉络
Chat Paper
正在生成论文摘要