Domain Generalization by Distribution Estimation.
International Journal of Machine Learning and Cybernetics(2023)
摘要
Domain generalization generalizes a prediction model trained on multiple source domains to an unseen target domain. The source and target domains are different but related, making cross domain model generalization challenging but possible. Existing works assume that the domains are related by a feature transformation that makes the marginal distributions, the class-conditional distributions, or the posterior distributions similar among the domains, and learn this transformation via kernel mean matching or adversarial training. Here, in a neural network context we relate the source and target domains via the network mapping, innovatively learn this mapping by matching multiple source joint distributions to their mixture distribution, and simultaneously learn a subsequent probabilistic classifier for target domain classification. To quantify the discrepancy among the source joint distributions, we exploit the Kullback–Leibler (KL) divergence, and show that in our case the KL divergence can be approximated via estimating a domain label posterior distribution. We model this discrete posterior distribution as multiple linear functions, and obtain their optimal parameters in an analytic manner. The resulting cost function is a combination of the cross-entropy loss and the estimated KL divergence, which is directly minimized via optimizing the network parameters. The experiments on several publicly available datasets demonstrate the effectiveness of our proposal. We release the source code at https://github.com/sentaochen/Domain-Generalization-by-Distribution-Estimation .
更多查看译文
关键词
Domain generalization,Distribution estimation,KL divergence,Neural network
AI 理解论文
溯源树
样例
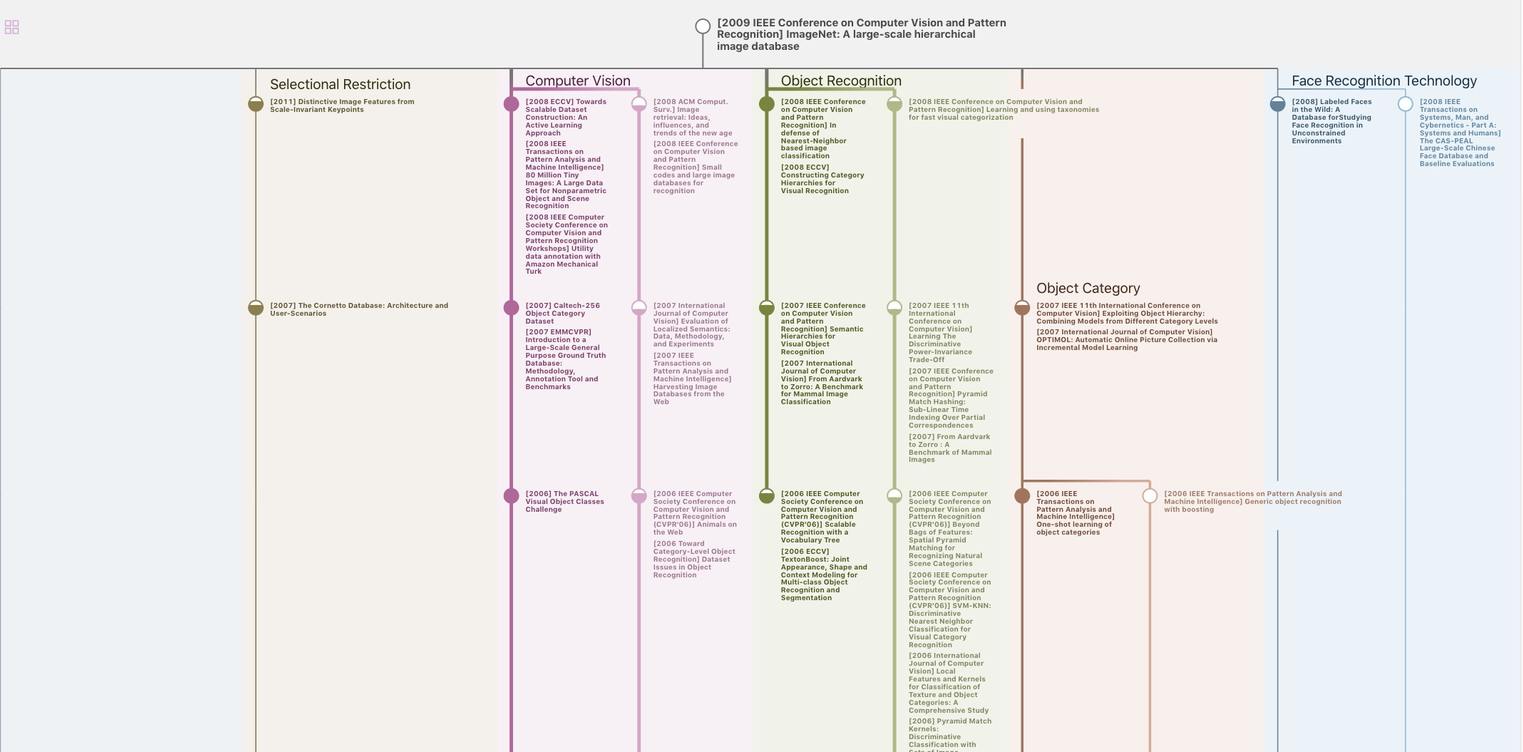
生成溯源树,研究论文发展脉络
Chat Paper
正在生成论文摘要