Category-aware dual adversarial domain adaptation model for rolling bearings fault diagnosis under variable conditions
MEASUREMENT SCIENCE AND TECHNOLOGY(2023)
摘要
The domain adaptation methods have good performance in solving the distribution discrepancy of vibration signals of rolling bearings under variable conditions, but without considering the alignment of different categories. To this end, a new dual adversarial domain adaptation (2ADA) mechanism for feature intra-category is proposed and a fault diagnosis model based on 2ADA is built in this paper. The method effectively uses category information to achieve category awareness, and avoids misclassification at the fuzzy decision boundary. In the training process, the multiple-kernel maximum mean discrepancy is used to reduce the discrepancy and perform a global alignment. The category-level alignment is performed when 2ADA is activated, which due to obtain more comprehensive domain adaptation performance and improve the accuracy of fault classification. The results of fault diagnosis experiments on the Case Western Reserve University (CWRU) bearing dataset and the rotating machinery fault platform dataset demonstrate that, the diagnosis accuracy of the proposed method is improved by up to 15.46% and 5.75% on tasks with high domain shift when compared with convolutional neural network method, which verifies the effectiveness of the method.
更多查看译文
关键词
fault diagnosis,rolling bearings,dual adversarial domain adaptation,variable conditions
AI 理解论文
溯源树
样例
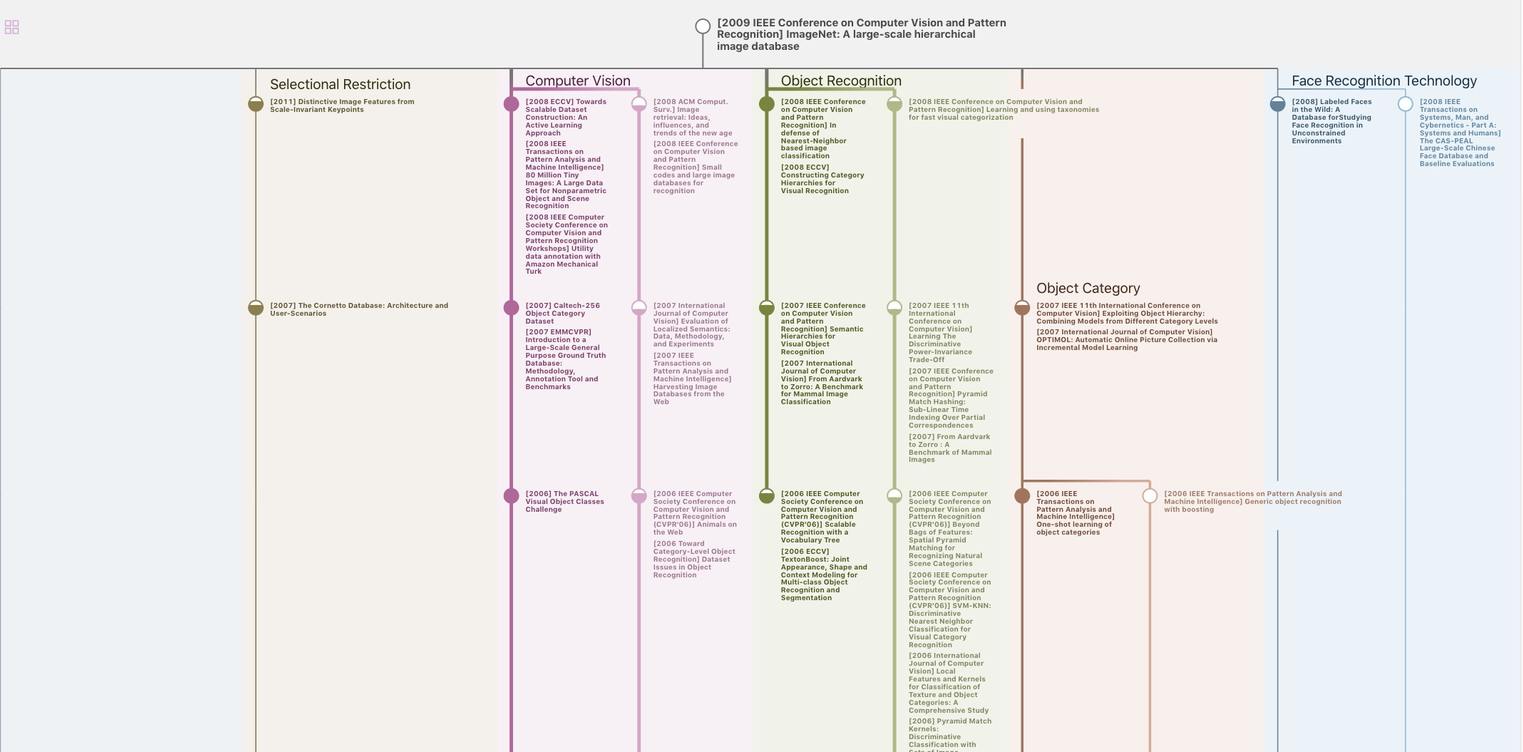
生成溯源树,研究论文发展脉络
Chat Paper
正在生成论文摘要